SimPINNs: Simulation-Driven Physics-Informed Neural Networks for Enhanced Performance in Nonlinear Inverse Problems
2023 13th Workshop on Hyperspectral Imaging and Signal Processing: Evolution in Remote Sensing (WHISPERS)(2023)
摘要
This paper introduces a novel approach to solve inverse problems by leveraging deep learning techniques. The objective is to infer unknown parameters that govern a physical system based on observed data. We focus on scenarios where the underlying forward model demonstrates pronounced nonlinear behaviour, and where the dimensionality of the unknown parameter space is substantially smaller than that of the observations. Our proposed method builds upon physics-informed neural networks (PINNs) trained with a hybrid loss function that combines observed data with simulated data generated by a known (approximate) physical model. Experimental results on an orbit restitution problem demonstrate that our approach surpasses the performance of standard PINNs, providing improved accuracy and robustness.
更多查看译文
关键词
Inverse problems,Neural Networks,Physics-Informed,Simulation
AI 理解论文
溯源树
样例
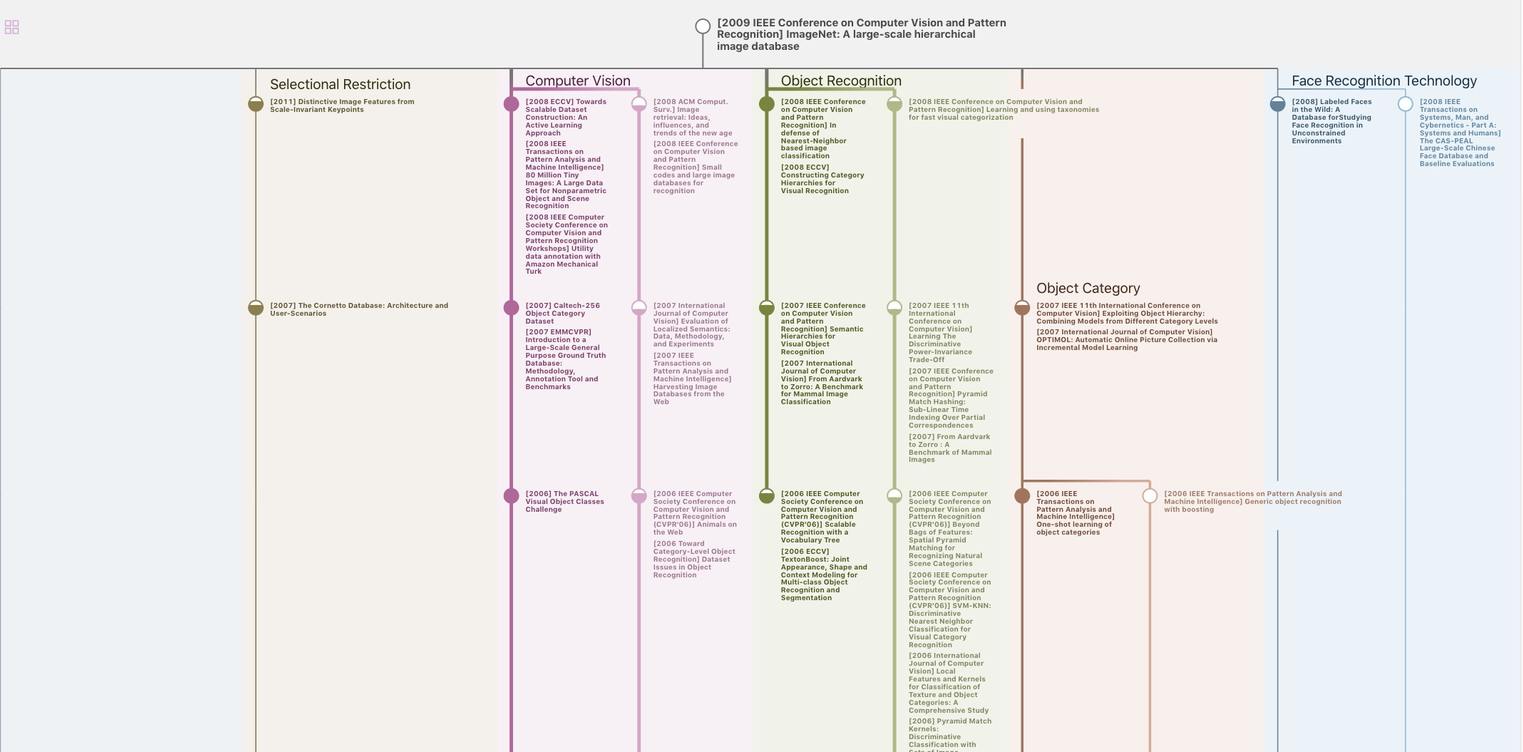
生成溯源树,研究论文发展脉络
Chat Paper
正在生成论文摘要