Memory in Plain Sight: Surveying the Uncanny Resemblances of Associative Memories and Diffusion Models
arXiv (Cornell University)(2023)
摘要
The generative process of Diffusion Models (DMs) has recently set
state-of-the-art on many AI generation benchmarks. Though the generative
process is traditionally understood as an "iterative denoiser", there is no
universally accepted language to describe it. We introduce a novel perspective
to describe DMs using the mathematical language of memory retrieval from the
field of energy-based Associative Memories (AMs), making efforts to keep our
presentation approachable to newcomers to both of these fields. Unifying these
two fields provides insight that DMs can be seen as a particular kind of AM
where Lyapunov stability guarantees are bypassed by intelligently engineering
the dynamics (i.e., the noise and step size schedules) of the denoising
process. Finally, we present a growing body of evidence that records DMs
exhibiting empirical behavior we would expect from AMs, and conclude by
discussing research opportunities that are revealed by understanding DMs as a
form of energy-based memory.
更多查看译文
关键词
uncanny resemblances,diffusion models,memory
AI 理解论文
溯源树
样例
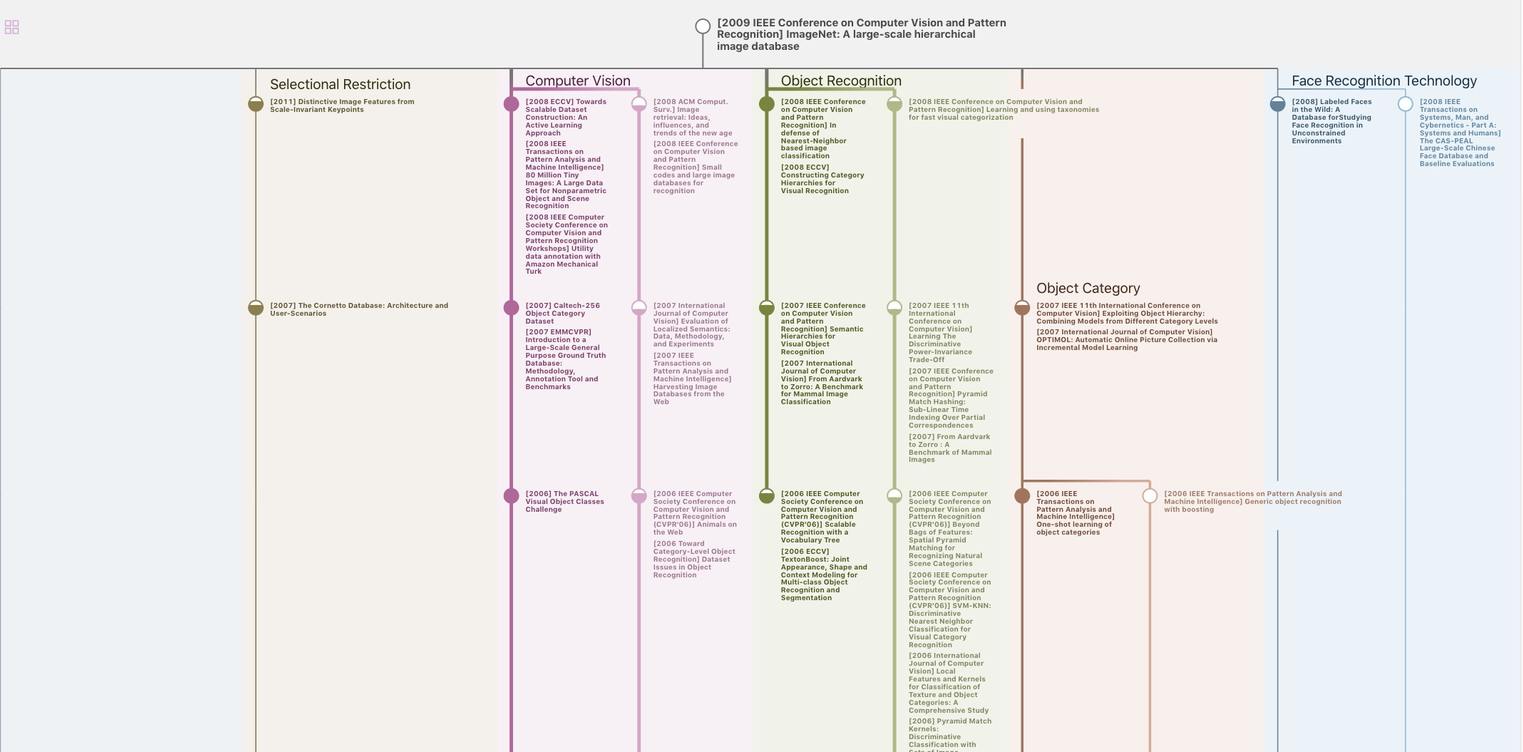
生成溯源树,研究论文发展脉络
Chat Paper
正在生成论文摘要