Towards a Unified Framework for Adaptable Problematic Content Detection via Continual Learning
CoRR(2023)
摘要
Detecting problematic content, such as hate speech, is a multifaceted and ever-changing task, influenced by social dynamics, user populations, diversity of sources, and evolving language. There has been significant efforts, both in academia and in industry, to develop annotated resources that capture various aspects of problematic content. Due to researchers' diverse objectives, the annotations are inconsistent and hence, reports of progress on detection of problematic content are fragmented. This pattern is expected to persist unless we consolidate resources considering the dynamic nature of the problem. We propose integrating the available resources, and leveraging their dynamic nature to break this pattern. In this paper, we introduce a continual learning benchmark and framework for problematic content detection comprising over 84 related tasks encompassing 15 annotation schemas from 8 sources. Our benchmark creates a novel measure of progress: prioritizing the adaptability of classifiers to evolving tasks over excelling in specific tasks. To ensure the continuous relevance of our framework, we designed it so that new tasks can easily be integrated into the benchmark. Our baseline results demonstrate the potential of continual learning in capturing the evolving content and adapting to novel manifestations of problematic content.
更多查看译文
关键词
adaptable problematic content detection
AI 理解论文
溯源树
样例
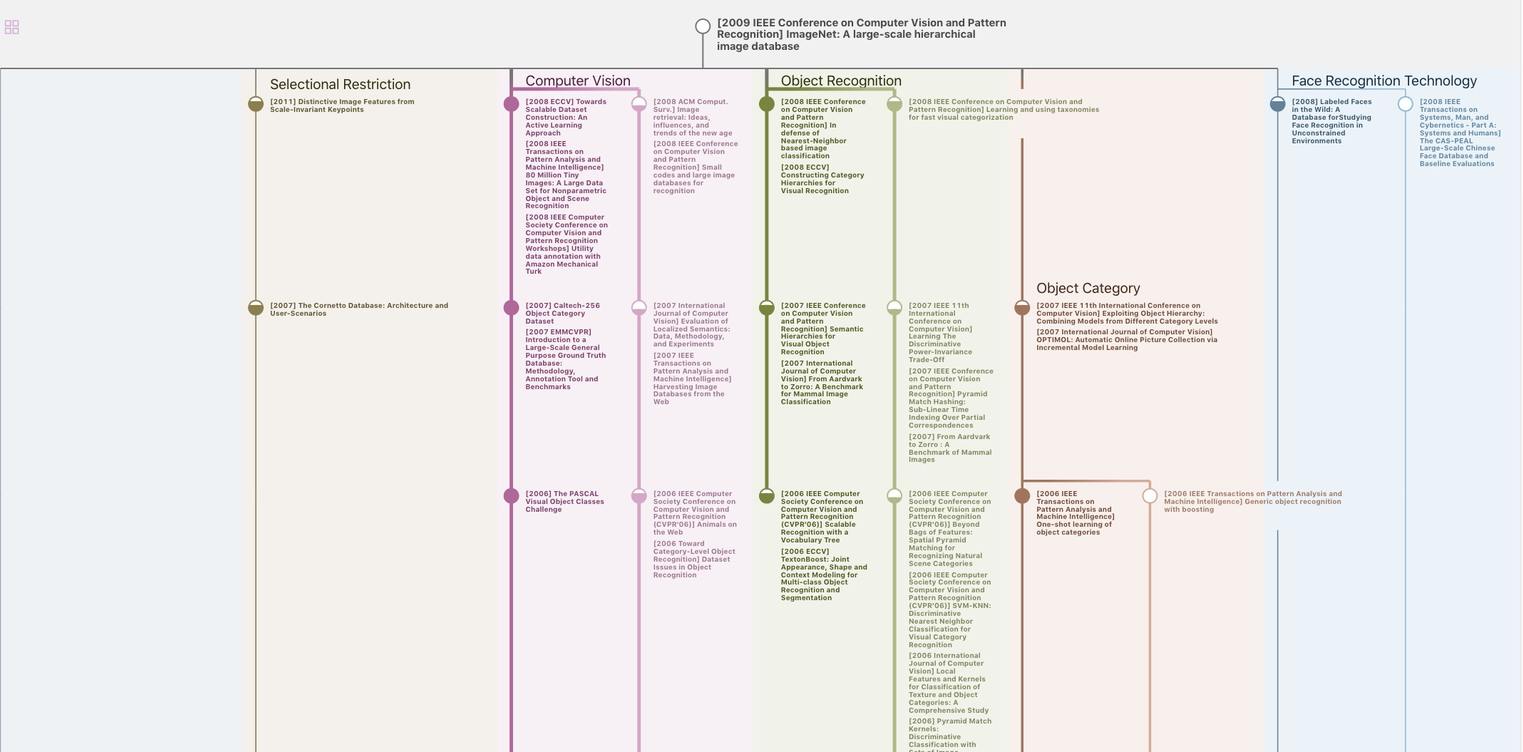
生成溯源树,研究论文发展脉络
Chat Paper
正在生成论文摘要