Physics-Aware Neural Dynamic Equivalence of Power Systems
IEEE TRANSACTIONS ON POWER SYSTEMS(2024)
摘要
This letter devises Neural Dynamic Equivalence (NeuDyE), which explores physics-aware machine learning and neural-ordinary-differential-equations (ODE-Net) to discover a dynamic equivalence of external power grids while preserving its dynamic behaviors after disturbances. The contributions are threefold: 1) an ODE-Net-enabled NeuDyE formulation to enable a continuous-time, data-driven dynamic equivalence of power systems; 2) a physics-informed NeuDyE learning method (PI-NeuDyE) to actively control the closed-loop accuracy of NeuDyE without an additional verification module; 3) a physics-guided NeuDyE (PG-NeuDyE) to enhance the method's applicability even in the absence of analytical physics models. Extensive case studies in the NPCC system validate the efficacy of NeuDyE, and, in particular, its capability under various contingencies.
更多查看译文
关键词
Dynamic equivalence,ODE-Net,physics-informed machine learning,model order reduction
AI 理解论文
溯源树
样例
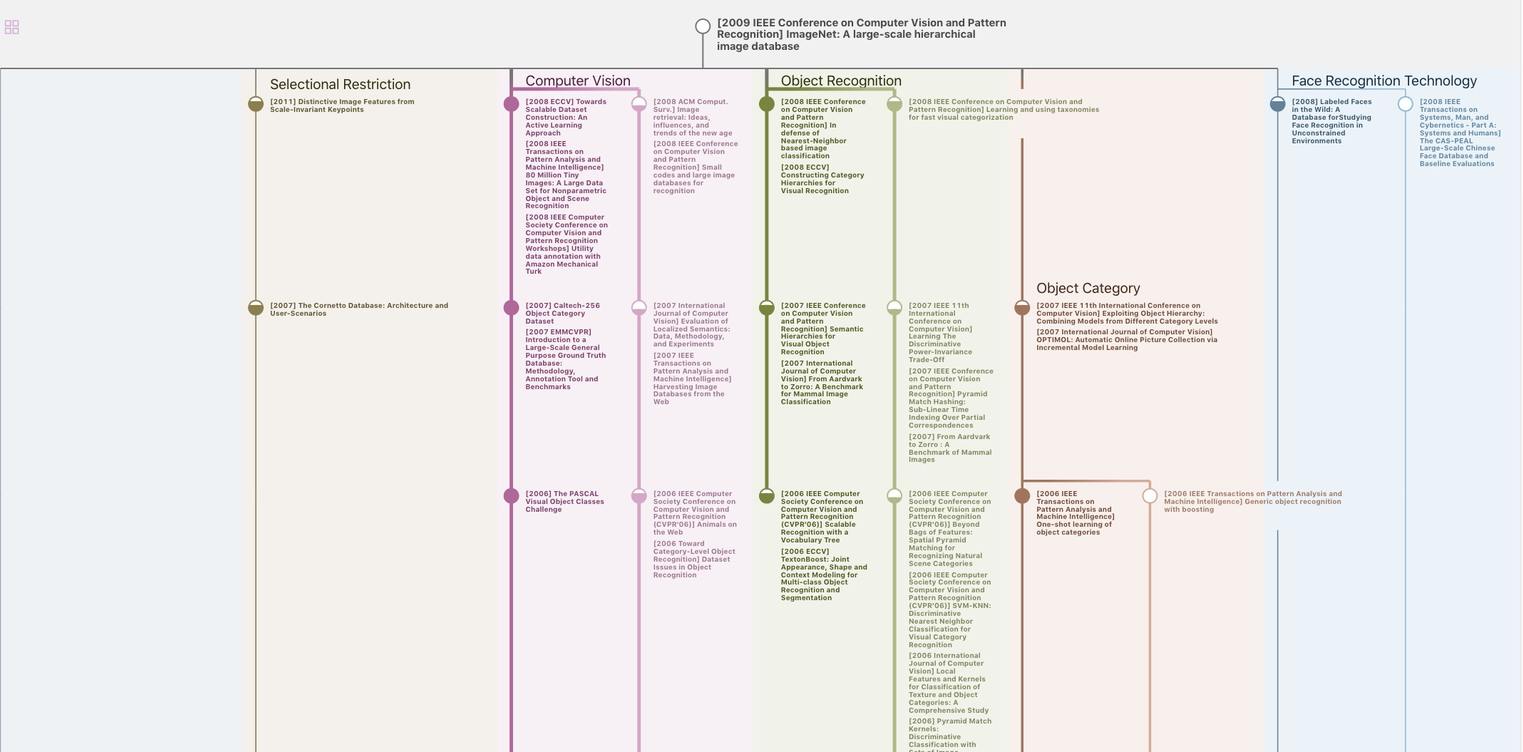
生成溯源树,研究论文发展脉络
Chat Paper
正在生成论文摘要