Multi-view Graph Network Learning Framework for Identification of Major Depressive Disorder
COMPUTERS IN BIOLOGY AND MEDICINE(2023)
Abstract
Functional connectivity (FC) derived from resting-state functional magnetic resonance imaging (rs-fMRI) exhibits non-Euclidean topological structures, which have pathological foundations and serve as ideal objective data for intelligent diagnosis of major depressive disorder (MDD) patients. Additionally, the fully connected FC demonstrates uniform spatial structures. To learn and integrate information from these two structural forms for a more comprehensive identification of MDD patients, we propose a novel hierarchical learning structure called Multi-View Graph Neural Network (MV-GNN). In MV-GNN, the collaborative FC of subjects is filtered and reconstructed from topological view to obtain the reconstructed FC, incorporating various threshold values to calculate the topological attributes of brain regions. ROC analysis is performed on the average scores of these attributes for MDD and healthy control (HC) groups to determine an efficient threshold. Group differences analysis is conducted on the efficient topological attributes of brain regions, followed by their selection. These efficient attributes, along with the reconstructed FC, are combined to construct a graph view using self-attention graph pooling and graph convolutional neural networks, enabling efficient embedding. To extract efficient FC pattern difference information from spatial view, a dual leave-one-out cross-feature selection method is proposed. It selects and extracts relevant information from uniformly sized FC structures' high-dimensional spatial features, constructing a relationship view between brain regions. This approach incorporates both the whole graph topological view and spatial relationship view in a multi-layered structure, fusing them using gating mechanisms. By incorporating multiple views, it enhances the inference of whether subjects suffer from MDD and reveals differential information between MDD and HC groups across different perspectives. The proposed model structure is evaluated through leave-one-site cross-validation and achieves an average accuracy of 65.61% in identifying MDD patients at a single-center site, surpassing state-of-the-art methods in MDD recognition. The model provides valuable discriminatory information for objective diagnosis of MDD and serves as a reference for pathological foundations.
MoreTranslated text
Key words
Major depressive disorder,Resting-state fMRI,Functional connectivity,Multi-view graph neural network,Intelligent diagnosis
AI Read Science
Must-Reading Tree
Example
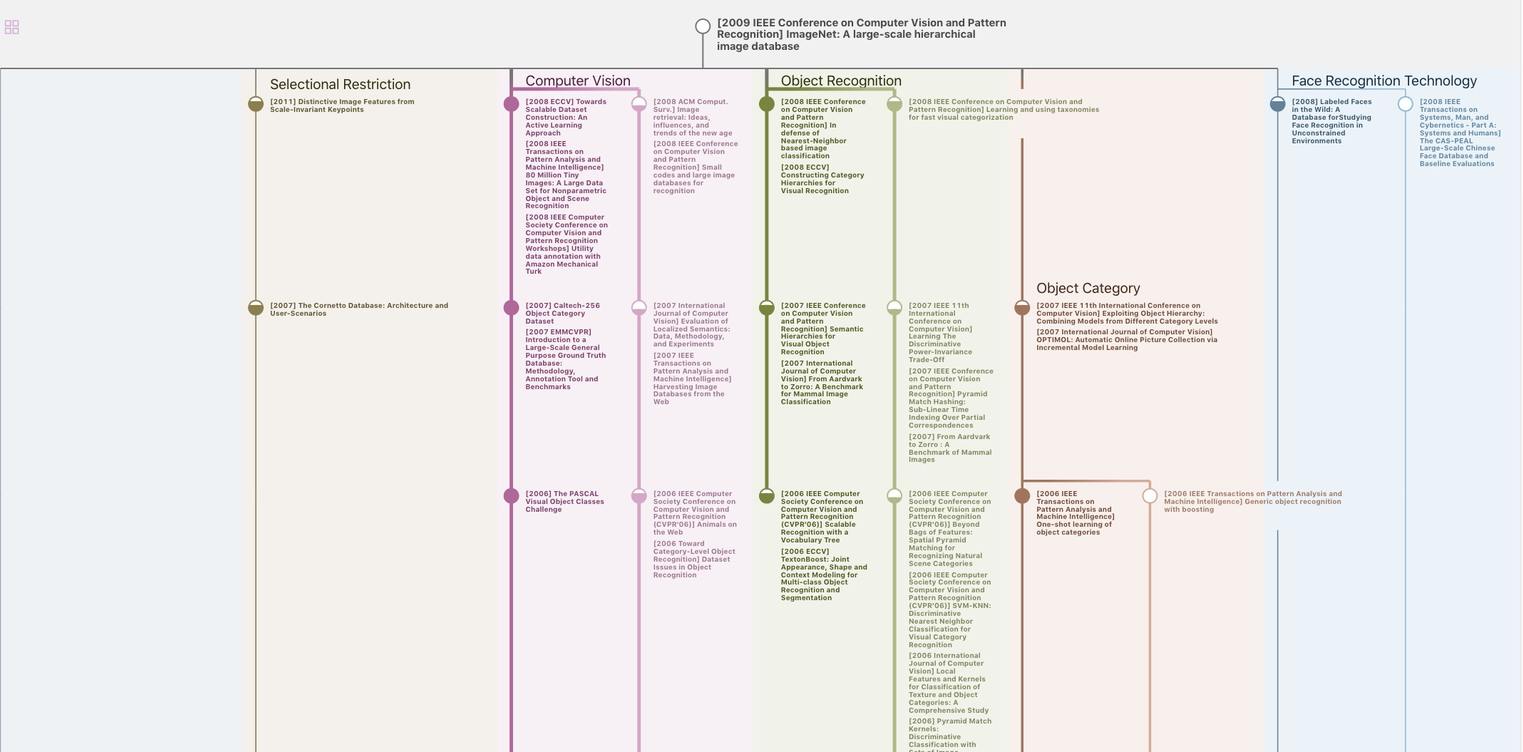
Generate MRT to find the research sequence of this paper
Chat Paper
Summary is being generated by the instructions you defined