Denoising and Selecting Pseudo-Heatmaps for Semi-Supervised Human Pose Estimation
2024 IEEE/CVF Winter Conference on Applications of Computer Vision (WACV)(2023)
Abstract
We propose a new semi-supervised learning design for human pose estimation that revisits the popular dual-student framework and enhances it two ways. First, we introduce a denoising scheme to generate reliable pseudo-heatmaps as targets for learning from unlabeled data. This uses multi-view augmentations and a threshold-and-refine procedure to produce a pool of pseudo-heatmaps. Second, we select the learning targets from these pseudo-heatmaps guided by the estimated cross-student uncertainty. We evaluate our proposed method on multiple evaluation setups on the COCO benchmark. Our results show that our model outperforms previous state-of-the-art semi-supervised pose estimators, especially in extreme low-data regime. For example with only 0.5K labeled images our method is capable of surpassing the best competitor by 7.22 mAP (+25% absolute improvement). We also demonstrate that our model can learn effectively from unlabeled data in the wild to further boost its generalization and performance.
MoreTranslated text
Key words
Algorithms,Biometrics,face,gesture,body pose,Algorithms,Machine learning architectures,formulations,and algorithms
AI Read Science
Must-Reading Tree
Example
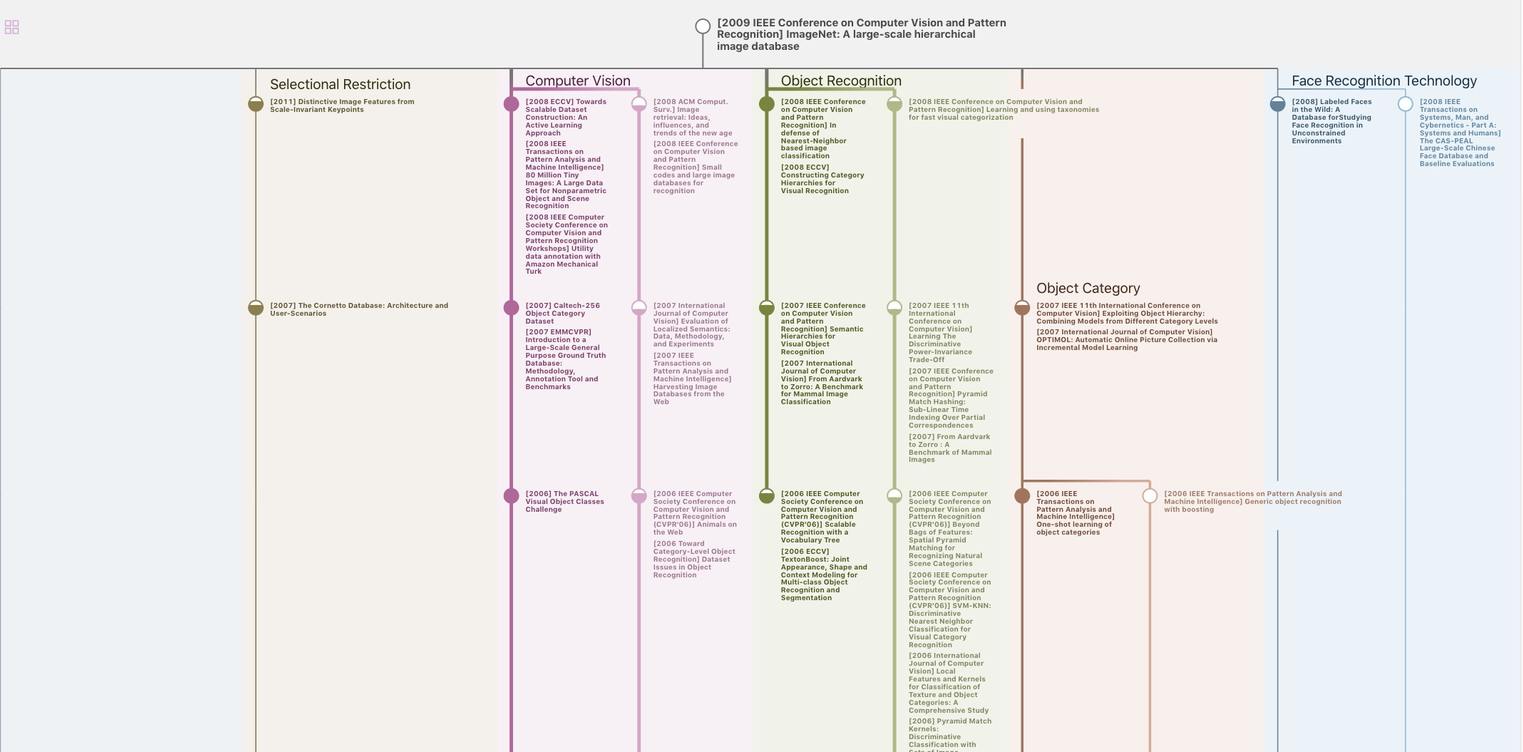
Generate MRT to find the research sequence of this paper
Chat Paper
Summary is being generated by the instructions you defined