Active Learning Based Fine-Tuning Framework for Speech Emotion Recognition
CoRR(2023)
摘要
Speech emotion recognition (SER) has drawn increasing attention for its applications in human-machine interaction. However, existing SER methods ignore the information gap between the pre-training speech recognition task and the downstream SER task, leading to sub-optimal performance. Moreover, they require much time to fine-tune on each specific speech dataset, restricting their effectiveness in real-world scenes with large-scale noisy data. To address these issues, we propose an active learning (AL) based Fine-Tuning framework for SER that leverages task adaptation pre-training (TAPT) and AL methods to enhance performance and efficiency. Specifically, we first use TAPT to minimize the information gap between the pre-training and the downstream task. Then, AL methods are used to iteratively select a subset of the most informative and diverse samples for fine-tuning, reducing time consumption. Experiments demonstrate that using only 20\%pt. samples improves 8.45\%pt. accuracy and reduces 79\%pt. time consumption.
更多查看译文
关键词
speech emotion
AI 理解论文
溯源树
样例
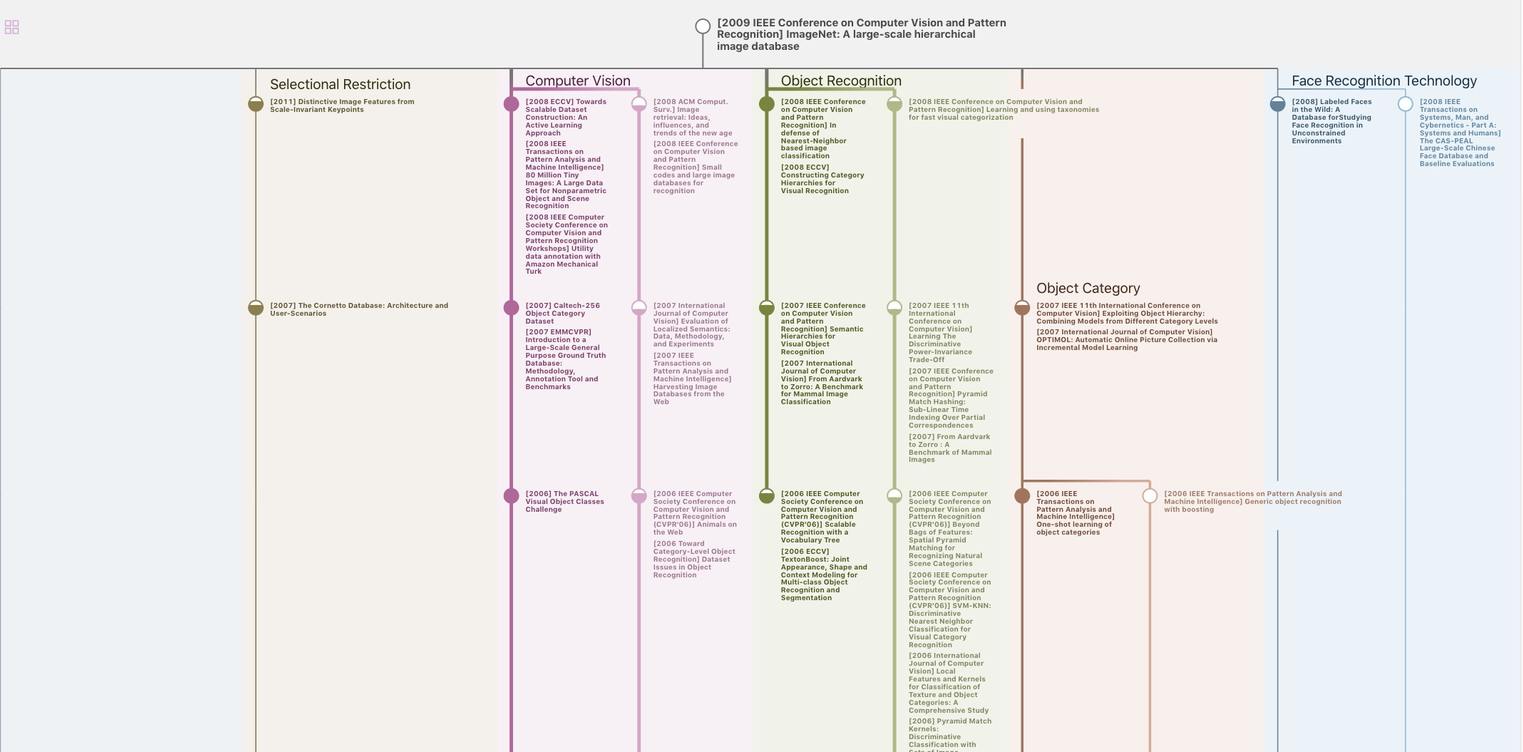
生成溯源树,研究论文发展脉络
Chat Paper
正在生成论文摘要