Decoding Realistic Images from Brain Activity with Contrastive Self-supervision and Latent Diffusion
ECAI 2023(2023)
摘要
Reconstructing visual stimuli from human brain activities provides a promising opportunity to advance our understanding of the brain's visual system and its connection with computer vision models. Although deep generative models have been employed for this task, the challenge of generating high-quality images with accurate semantics persists due to the intricate underlying representations of brain signals and the limited availability of parallel data. In this paper, we propose a two-phase framework named Contrast and Diffuse (CnD) to decode realistic images from functional magnetic resonance imaging (fMRI) recordings. In the first phase, we acquire representations of fMRI data through self-supervised contrastive learning. In the second phase, the encoded fMRI representations condition the diffusion model to reconstruct visual stimulus through our proposed concept-aware conditioning method. Experimental results show that CnD reconstructs highly plausible images on challenging benchmarks. We also provide a quantitative interpretation of the connection between the latent diffusion model (LDM) components and the human brain's visual system. In summary, we present an effective approach for reconstructing visual stimuli based on human brain activity and offer a novel framework to understand the relationship between the diffusion model and the human brain visual system.
更多查看译文
关键词
latent diffusion,brain activity,realistic images,self-supervision
AI 理解论文
溯源树
样例
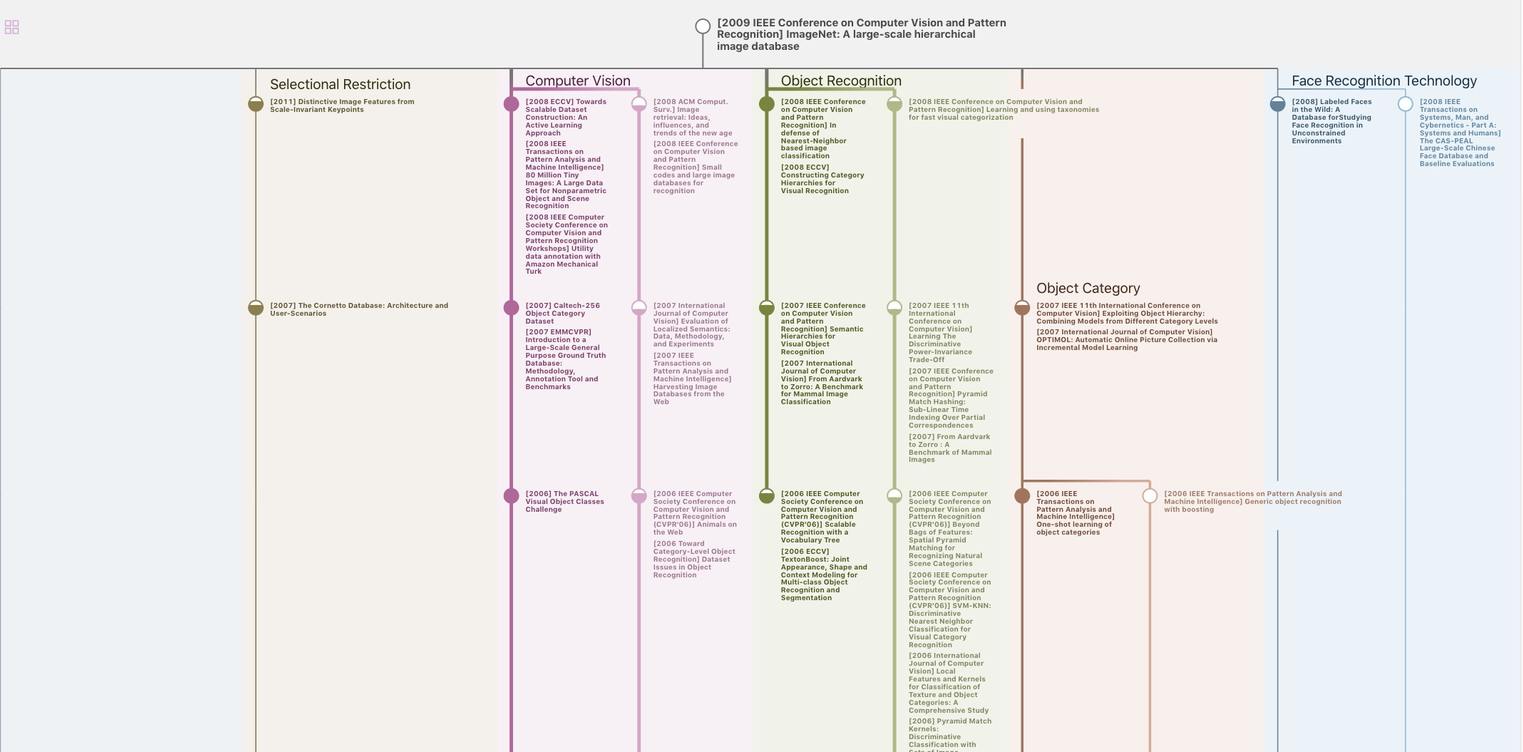
生成溯源树,研究论文发展脉络
Chat Paper
正在生成论文摘要