Empowering Many, Biasing a Few: Generalist Credit Scoring through Large Language Models
CoRR(2023)
摘要
In the financial industry, credit scoring is a fundamental element, shaping
access to credit and determining the terms of loans for individuals and
businesses alike. Traditional credit scoring methods, however, often grapple
with challenges such as narrow knowledge scope and isolated evaluation of
credit tasks. Our work posits that Large Language Models (LLMs) have great
potential for credit scoring tasks, with strong generalization ability across
multiple tasks. To systematically explore LLMs for credit scoring, we propose
the first open-source comprehensive framework. We curate a novel benchmark
covering 9 datasets with 14K samples, tailored for credit assessment and a
critical examination of potential biases within LLMs, and the novel instruction
tuning data with over 45k samples. We then propose the first Credit and Risk
Assessment Large Language Model (CALM) by instruction tuning, tailored to the
nuanced demands of various financial risk assessment tasks. We evaluate CALM,
existing state-of-art (SOTA) methods, open source and closed source LLMs on the
build benchmark. Our empirical results illuminate the capability of LLMs to not
only match but surpass conventional models, pointing towards a future where
credit scoring can be more inclusive, comprehensive, and unbiased. We
contribute to the industry's transformation by sharing our pioneering
instruction-tuning datasets, credit and risk assessment LLM, and benchmarks
with the research community and the financial industry.
更多查看译文
关键词
generalist credit scoring,language
AI 理解论文
溯源树
样例
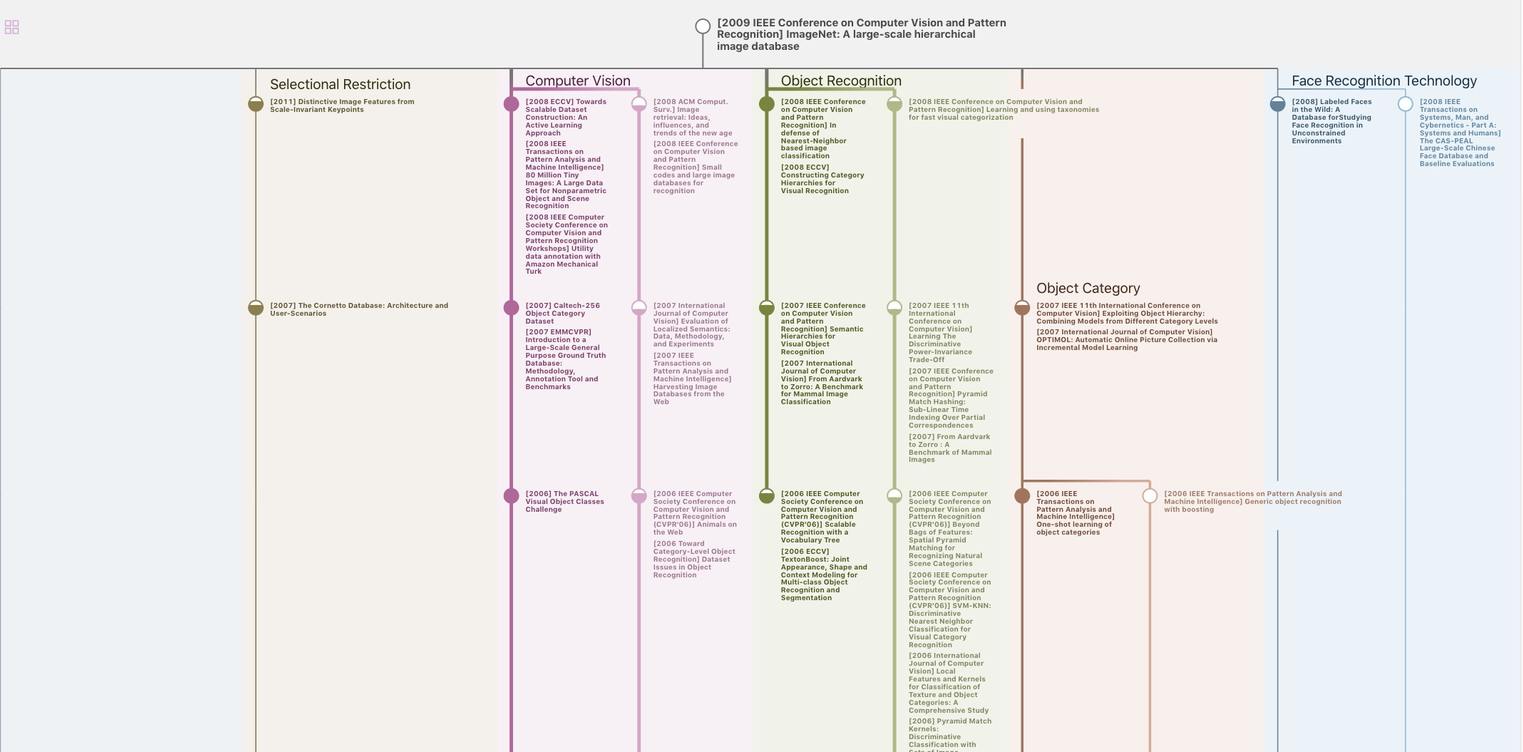
生成溯源树,研究论文发展脉络
Chat Paper
正在生成论文摘要