Stability and Generalization for Minibatch SGD and Local SGD
CoRR(2023)
摘要
The increasing scale of data propels the popularity of leveraging parallelism to speed up the optimization. Minibatch stochastic gradient descent (minibatch SGD) and local SGD are two popular methods for parallel optimization. The existing theoretical studies show a linear speedup of these methods with respect to the number of machines, which, however, is measured by optimization errors. As a comparison, the stability and generalization of these methods are much less studied. In this paper, we pioneer the stability and generalization analysis of minibatch and local SGD to understand their learnability. We incorporate training errors into the stability analysis, which shows how small training errors help generalization for overparameterized models. Our stability bounds imply optimistic risk bounds which decay fast under a low noise condition. We show both minibatch and local SGD achieve a linear speedup to attain the optimal risk bounds.
更多查看译文
关键词
minibatch sgd,local sgd,stability,generalization
AI 理解论文
溯源树
样例
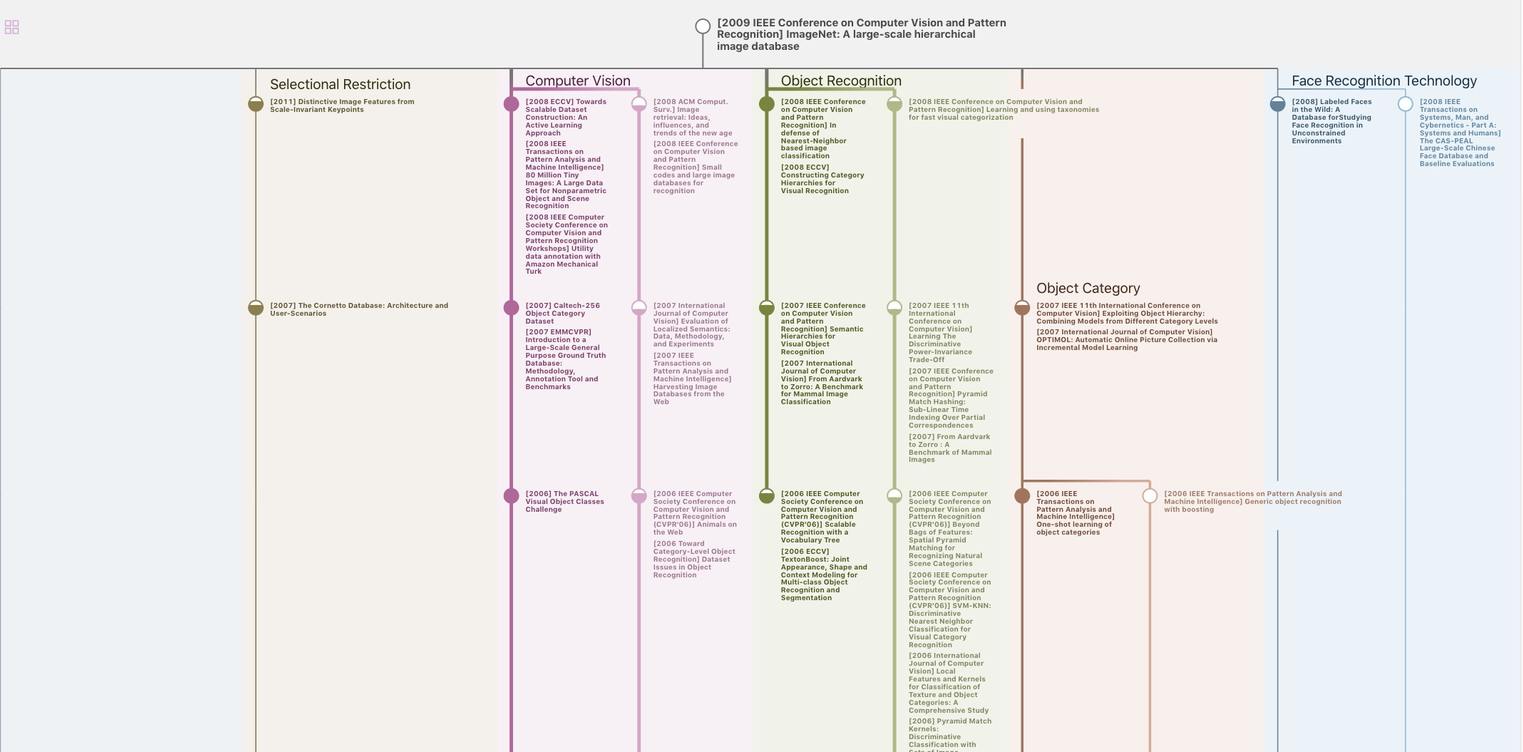
生成溯源树,研究论文发展脉络
Chat Paper
正在生成论文摘要