Self-distilled Masked Attention guided masked image modeling with noise Regularized Teacher (SMART) for medical image analysis
CoRR(2023)
摘要
Hierarchical shifted window transformers (Swin) are a computationally efficient and more accurate alternative to plain vision transformers. Masked image modeling (MIM)-based pretraining is highly effective in increasing models' transferability to a variety of downstream tasks. However, more accurate and efficient attention guided MIM approaches are difficult to implement with Swin due to it's lack of an explicit global attention. We thus architecturally enhanced Swin with semantic class attention for self-supervised attention guided co-distillation with MIM. We also introduced a noise injected momentum teacher, implemented with patch dropout of teacher's inputs for improved training regularization and accuracy. Our approach, called \underline{s}elf-distilled \underline{m}asked \underline{a}ttention MIM with noise \underline{r}egularized \underline{t}eacher (SMART) was pretrained with \textbf{10,412} unlabeled 3D computed tomography (CT)s of multiple disease sites and sourced from institutional and public datasets. We evaluated SMART for multiple downstream tasks involving analysis of 3D CTs of lung cancer (LC) patients for: (i) [Task I] predicting immunotherapy response in advanced stage LC (n = 200 internal dataset), (ii) [Task II] predicting LC recurrence in early stage LC before surgery (n = 156 public dataset), (iii) [Task III] LC segmentation (n = 200 internal, 21 public dataset), and (iv) [Task IV] unsupervised clustering of organs in the chest and abdomen (n = 1,743 public dataset) \underline{without} finetuning. SMART predicted immunotherapy response with an AUC of 0.916, LC recurrence with an AUC of 0.793, segmented LC with Dice accuracy of 0.81, and clustered organs with an inter-class cluster distance of 5.94, indicating capability of attention guided MIM for Swin in medical image analysis.
更多查看译文
关键词
regularized teacher,masked attention,self-distilled
AI 理解论文
溯源树
样例
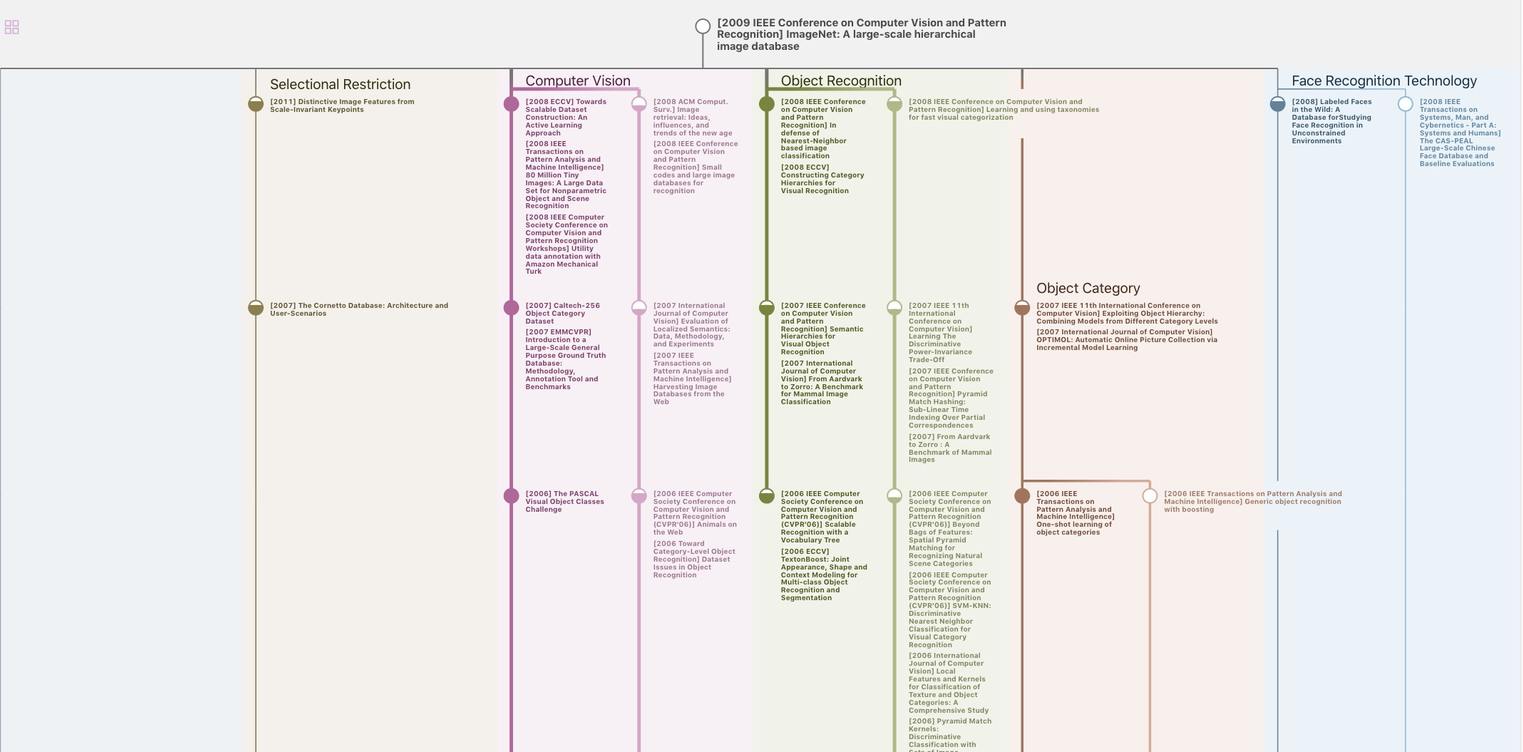
生成溯源树,研究论文发展脉络
Chat Paper
正在生成论文摘要