Predicting response to CCRT for esophageal squamous carcinoma by a radiomics-clinical SHAP model
BMC MEDICAL IMAGING(2023)
摘要
Background Radical concurrent chemoradiotherapy (CCRT) is frequently used as the first-line treatment for patients with locally advanced esophageal cancer. Unfortunately, some patients respond poorly. To predict response to radical concurrent chemoradiotherapy in pre-treatment patients with esophageal squamous carcinoma (ESCC), and compare the predicting efficacies of radiomics features of primary tumor with or without regional lymph nodes, we developed a radiomics-clinical model based on the positioning CT images. Finally, SHapley Additive exPlanation (SHAP) was used to explain the models.Methods This retrospective study enrolled 105 patients with medically inoperable and/or unresectable ESCC who underwent radical concurrent chemoradiotherapy (CCRT) between October 2018 and May 2023. Patients were classified into responder and non-responder groups with RECIST standards. The 11 recently admitted patients were chosen as the validation set, previously admitted patients were randomly split into the training set (n = 70) and the testing set (n = 24). Primary tumor site (GTV), the primary tumor and the uninvolved lymph nodes at risk of microscopic disease (CTV) were identified as Regions of Interests (ROIs). 1762 radiomics features from GTV and CTV were respectively extracted and then filtered by statistical differential analysis and Least Absolute Shrinkage and Selection Operator (LASSO). The filtered radiomics features combined with 13 clinical features were further filtered with Mutual Information (MI) algorithm. Based on the filtered features, we developed five models (Clinical Model, GTV Model, GTV-Clinical Model, CTV Model, and CTV-Clinical Model) using the random forest algorithm and evaluated for their accuracy, precision, recall, F1-Score and AUC. Finally, SHAP algorithm was adopted for model interpretation to achieve transparency and utilizability.Results The GTV-Clinical model achieves an AUC of 0.82 with a 95% confidence interval (CI) of 0.76-0.99 on testing set and an AUC of 0.97 with a 95% confidence interval (CI) of 0.84-1.0 on validation set, which are significantly higher than those of other models in predicting ESCC response to CCRT. The SHAP force map provides an integrated view of the impact of each feature on individual patients, while the SHAP summary plots indicate that radiomics features have a greater influence on model prediction than clinical factors in our model.Conclusion GTV-Clinical model based on texture features and the maximum diameter of lesion (MDL) may assist clinicians in pre-treatment predicting ESCC response to CCRT.
更多查看译文
关键词
esophageal squamous carcinoma,ccrt,radiomics-clinical
AI 理解论文
溯源树
样例
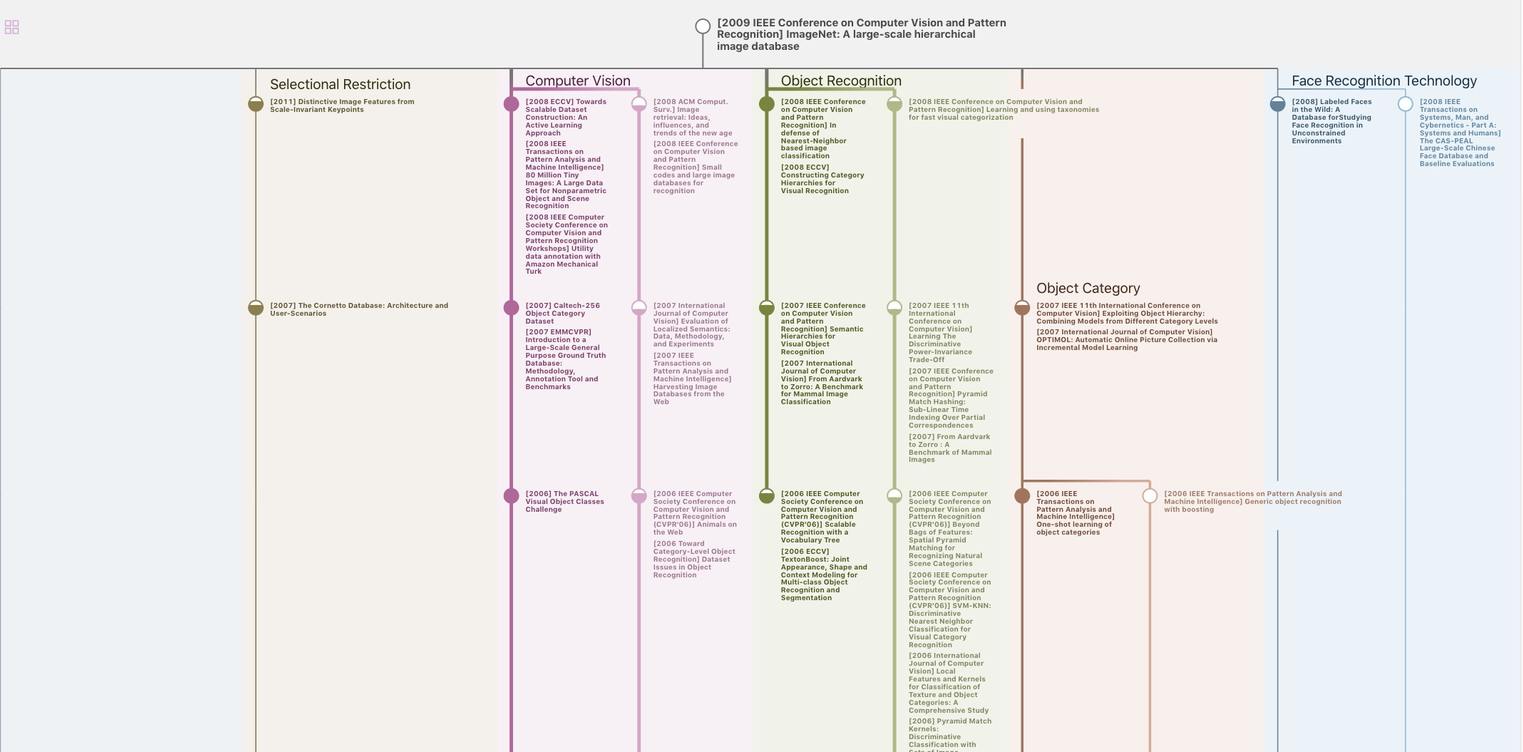
生成溯源树,研究论文发展脉络
Chat Paper
正在生成论文摘要