Grid-Robust Efficient Neural Interface Model for Universal Molecule Surface Construction from Point Clouds
The journal of physical chemistry letters(2023)
摘要
Molecular surfaces play a pivotal role in elucidating the properties and functions of biological complexes. While various surfaces have been proposed for specific scenarios, their widespread adoption faces challenges due to limited efficiency stemming from hand-crafted modeling designs. In this work, we proposed a general framework that incorporates both the point cloud concept and neural networks. The use of matrix multiplication in this framework enables efficient implementation across diverse platforms and libraries. We applied this framework to develop the GENIUSES (Grid-robust Efficient Neural Interface for Universal Solvent -Excluded Surface) model for constructing SES. GENIUSES demonstrates high accuracy and efficiency across data sets with varying conformations and complexities. Compared to the classical implementation of SES in the AMBER software package, our framework achieved a 26-fold speedup while retaining similar to 95% accuracy when ported to the GPU platform using CUDA. Greater speedups can be obtained in large-scale systems. Importantly, our model exhibits robustness against variations in the grid spacing. We have integrated this infrastructure into AMBER to enhance accessibility for research in drug screening and related fields, where efficiency is of paramount importance.
更多查看译文
关键词
universal molecule surface construction,grid-robust
AI 理解论文
溯源树
样例
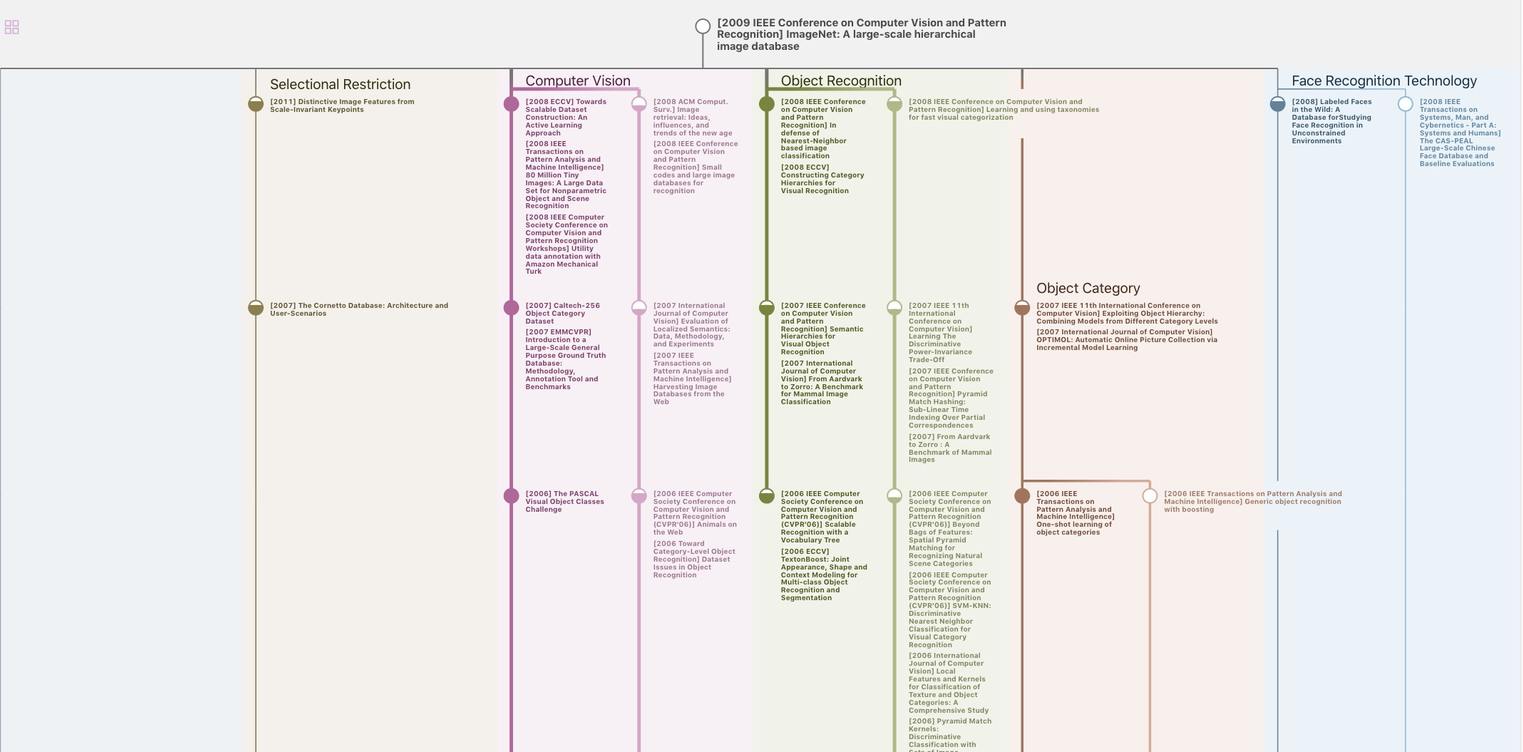
生成溯源树,研究论文发展脉络
Chat Paper
正在生成论文摘要