WDVR-RAM: A 0.25–1.2 V, 2.6–76 POPS/W Charge-Domain In-Memory-Computing Binarized CNN Accelerator for Dynamic AIoT Workloads
IEEE Transactions on Circuits and Systems I: Regular Papers(2023)
摘要
In-memory computing (IMC) is an effective approach to accelerate the interference tasks of binary neural networks (BNN), which has been widely used in artificial intelligence of things (AIoT) applications. However, previous researches only focus on optimizing energy efficiency for a narrow voltage range. This poses a severe limitation for some AIoT applications, because they may have very dynamic workloads that require the energy efficiency optimization of BNN for a wide dynamic voltage range (WDVR). To address this issue, we have developed a novel IMC-based BNN accelerator, supporting energy-efficient operations in a wide dynamic voltage range. First, we analyze different charge-domain architectures in terms of their errors and energy characteristics, and decide the best architecture that is suitable for a wide dynamic voltage range. Second, we introduce a lazy convolution bitline reset (LCBR) scheme to further optimize the energy of multiplication and accumulation (MAC) within the entire voltage range. Third, we design a subthreshold differential batch-normalization amplifier (SDBNA) array to compensate for the inference accuracy loss for the lower end of the voltage range. A 16-Kb WDVR-RAM has been designed and fabricated in a 55-nm CMOS process. Measurement results show that the test chip achieves a peak energy efficiency of 76193-2645 TOPS/W at 0.25-1.2 V for MAC, and a peak energy efficiency of 56141-1973 TOPS/W at 0.25-1.2V for both MAC and batch normalization (BN) layers. For throughput, our work obtains a peak computing density range of 692-144078 GOPS/mm2 with 28.31-0.17 ms/frame (CIFAR-10). Moreover, it also achieves the highest 14.75% and 31.87% recovery for Top-1 accuracy observed for MNIST and CIFAR-10 so far, respectively.
更多查看译文
关键词
Binary neural network, in-memory computing, SRAM, wide dynamic voltage range, energy efficiency, dynamic workloads, AIoT
AI 理解论文
溯源树
样例
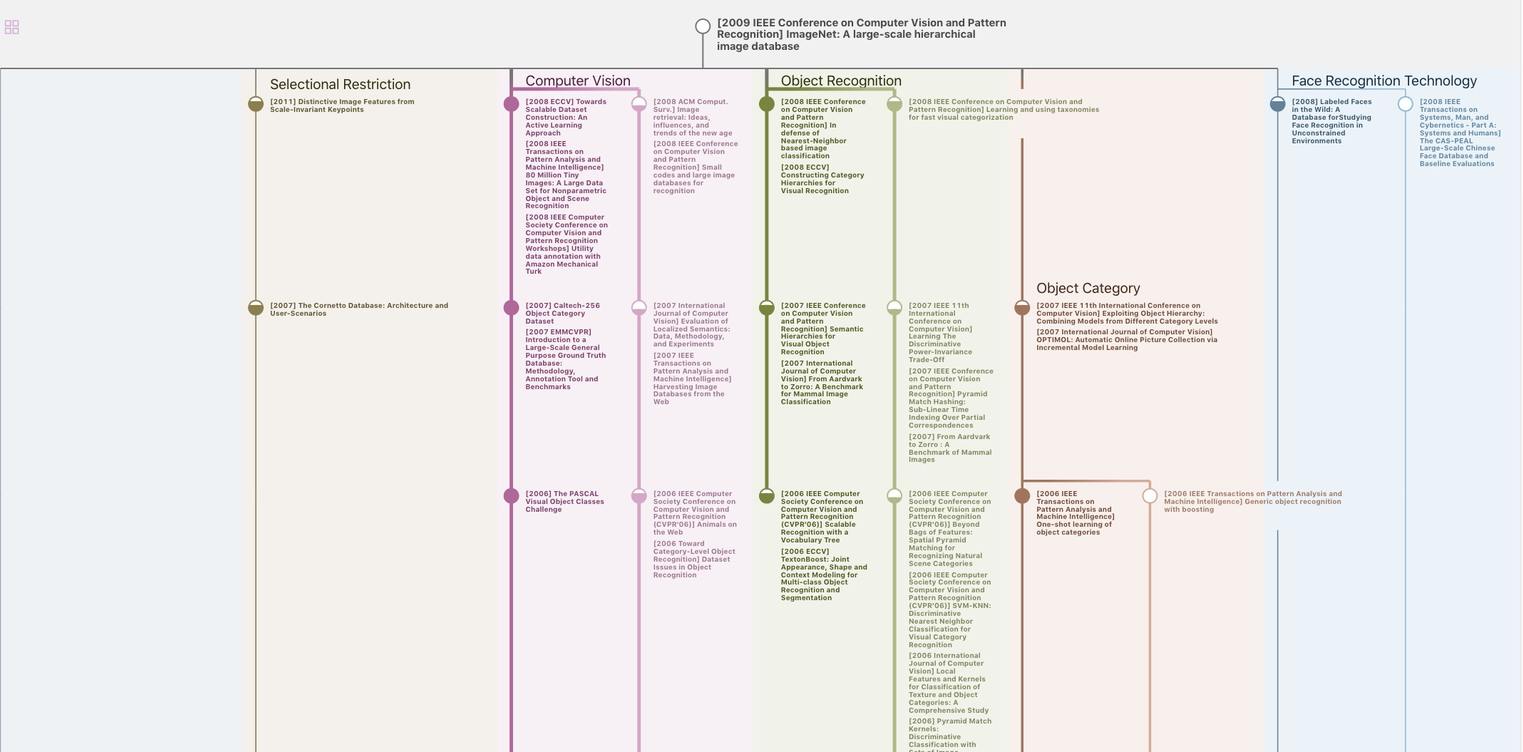
生成溯源树,研究论文发展脉络
Chat Paper
正在生成论文摘要