A Comparison of Machine Learning Models for Predicting CRISPR/Cas On-target Efficacy
2023 IEEE Conference on Computational Intelligence in Bioinformatics and Computational Biology (CIBCB)(2023)
摘要
CRISPR/Cas (Clustered Regularly Interspaced Short Palindromic Repeats and CRISPR-associated protein) is a powerful technology that can precisely modify DNA, enabling the treatment of several genetic disorders. The CRISPR/Cas system is comprised of a nuclease, which induces the modification, and a sgRNA (single guide RNA), which targets the nuclease to a precise location in the DNA. Designing sgRNAs is time-consuming and resource-intensive, thus computational tools are used to screen sgRNAs for their on-target efficacy. However, to date, models for predicting on-target efficacy have been restricted in complexity due to data limitations. Recently, a large on-target dataset has been published, relieving some of the restraints towards creating larger computational models. Herein, we present a comparison of several types of deep learning models, including CNNs (Convolutional Neural Networks) and RNNs (Recurrent Neural Networks), using the new dataset to predict the on-target efficacy of sgRNAs. After determining which general model performs best on this dataset, we assessed the impact of adding an important biological feature, the GC content, and compared the models to a state-of-the-art competitor, DeepHF [1].
更多查看译文
关键词
CRISPR-Cas, sgRNA, Genome editing, Machine learning, Deep learning
AI 理解论文
溯源树
样例
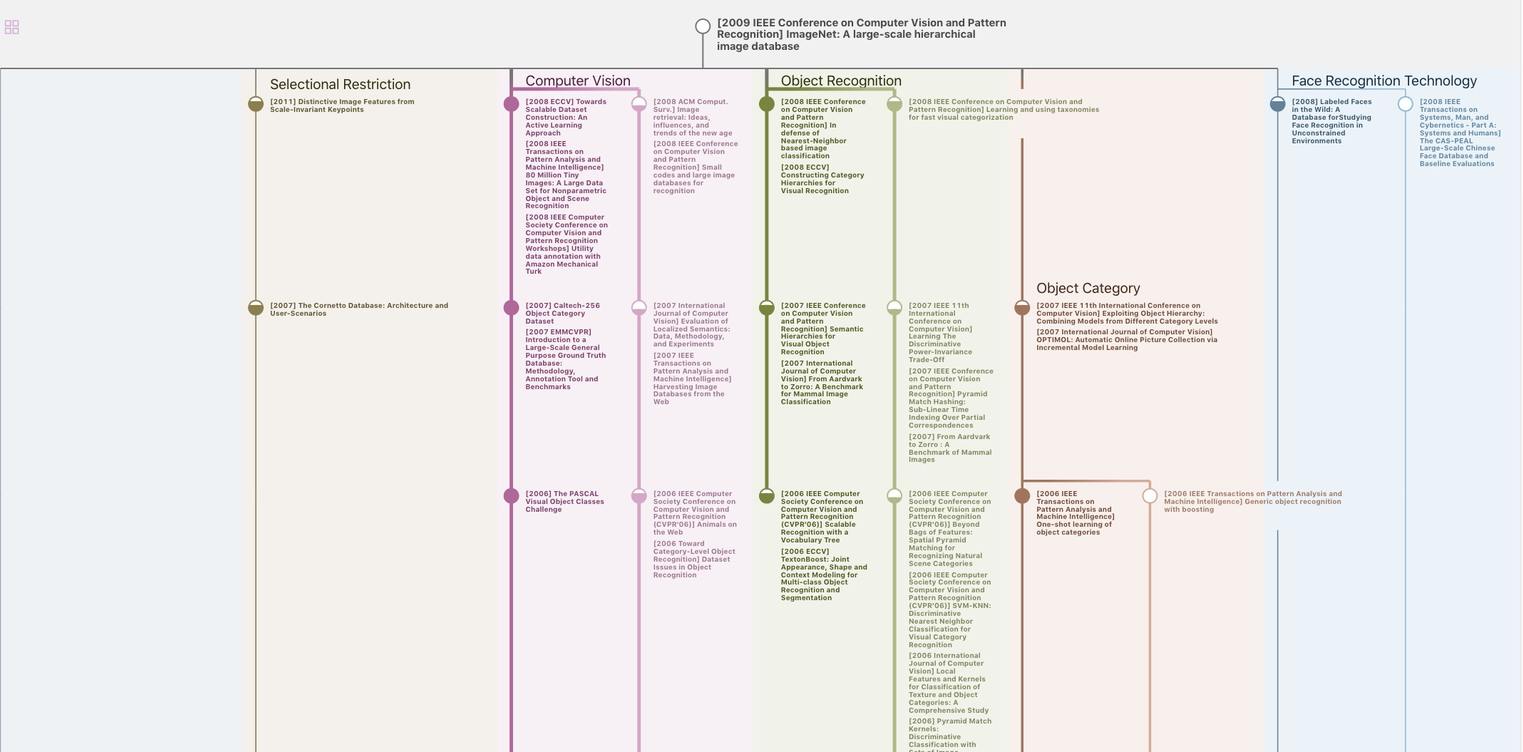
生成溯源树,研究论文发展脉络
Chat Paper
正在生成论文摘要