An Explainable Deep Learning Model for Prediction of Severity of Alzheimer’s Disease
2023 IEEE Conference on Computational Intelligence in Bioinformatics and Computational Biology (CIBCB)(2023)
摘要
Deep Convolutional Neural Networks (CNNs) have become the go-to method for medical imaging classification on various imaging modalities for binary and multiclass problems. Deep CNNs extract spatial features from image data hierarchically, with deeper layers learning more relevant features for the classification application. Despite the high predictive accuracy, usability lags in practical applications due to the black-box model perception. Model explainability and interpretability are essential for successfully integrating artificial intelligence into healthcare practice. This work addresses the challenge of an explainable deep learning model for the prediction of the severity of Alzheimer’s disease (AD). AD diagnosis and prognosis heavily rely on neuroimaging information, particularly magnetic resonance imaging (MRI). We present a deep learning model framework that integrates a local data-driven interpretation method that explains the relationship between the predicted AD severity from the CNN and the input MR brain image. The deep explainer uses SHapley Additive exPlanation values to quantity the contribution of different brain regions utilized by the CNN to predict outcomes. We conduct a comparative analysis of three high-performing CNN models: DenseNet121, DenseNet169, and Inception-ResNet-v2. The framework shows high sensitivity and specificity in the test sample of subjects with varying levels of AD severity. We also correlated five key AD neurocognitive assessment outcome measures and the APOE genotype biomarker with model misclassifications to facilitate a better understanding of model performance.
更多查看译文
关键词
Alzheimer’s,Disease,MRI,Deep learning,Explainability,Prediction models
AI 理解论文
溯源树
样例
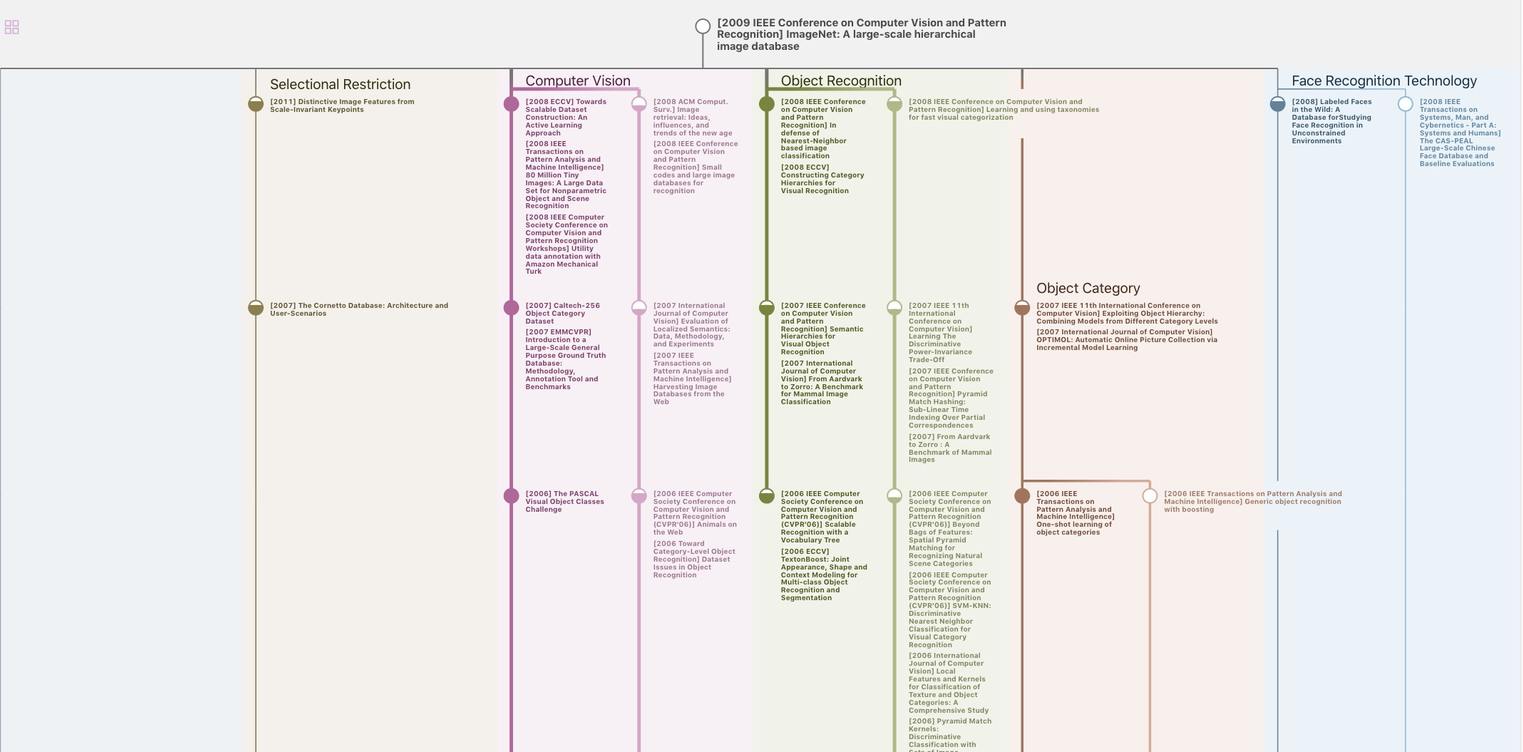
生成溯源树,研究论文发展脉络
Chat Paper
正在生成论文摘要