Memory-efficient particle filter recurrent neural network for object localization
CoRR(2023)
摘要
This study proposes a novel memory-efficient recurrent neural network (RNN) architecture specified to solve the object localization problem. This problem is to recover the object states along with its movement in a noisy environment. We take the idea of the classical particle filter and combine it with GRU RNN architecture. The key feature of the resulting memory-efficient particle filter RNN model (mePFRNN) is that it requires the same number of parameters to process environments of different sizes. Thus, the proposed mePFRNN architecture consumes less memory to store parameters compared to the previously proposed PFRNN model. To demonstrate the performance of our model, we test it on symmetric and noisy environments that are incredibly challenging for filtering algorithms. In our experiments, the mePFRNN model provides more precise localization than the considered competitors and requires fewer trained parameters.
更多查看译文
关键词
localization,particle,object
AI 理解论文
溯源树
样例
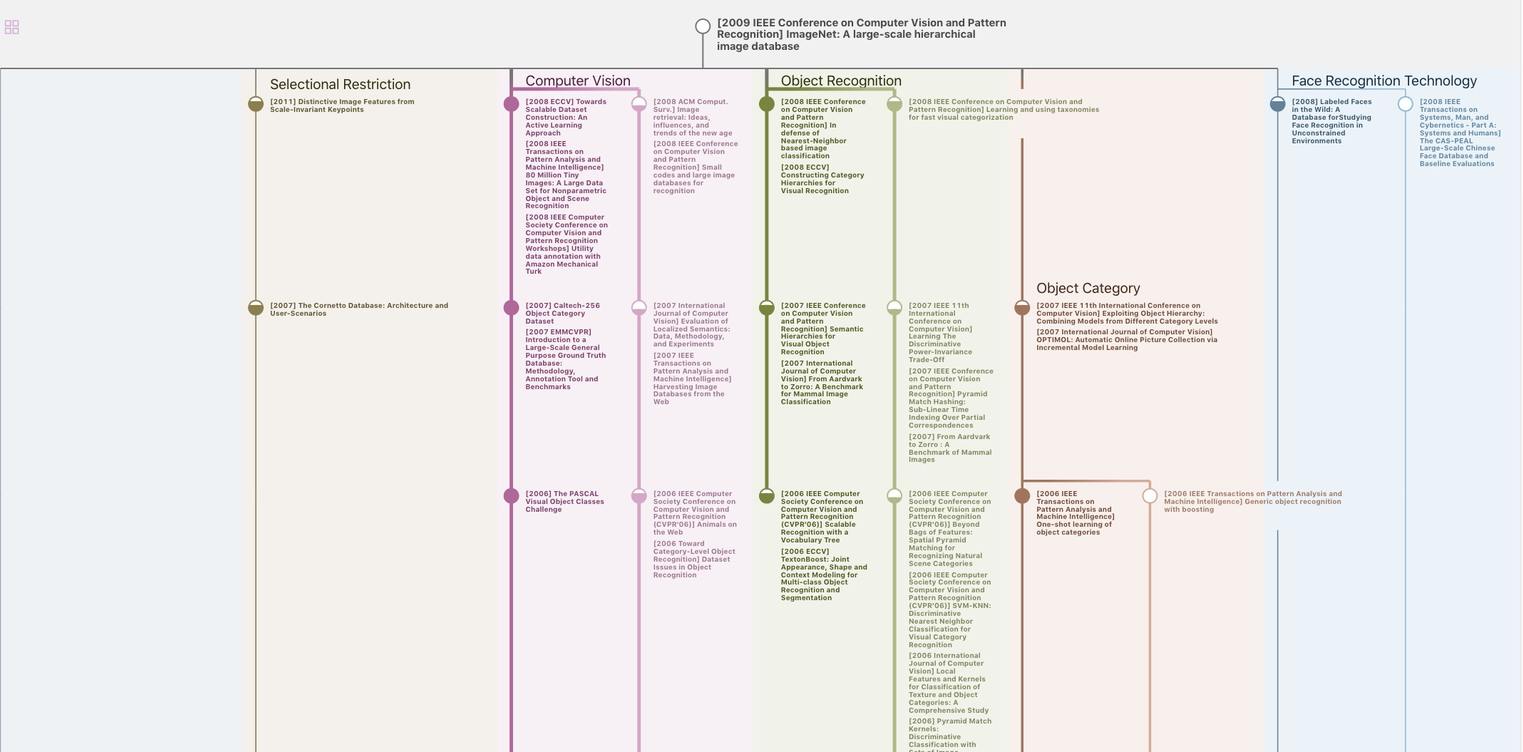
生成溯源树,研究论文发展脉络
Chat Paper
正在生成论文摘要