Primal-dual hybrid gradient algorithms for computing time-implicit Hamilton-Jacobi equations
CoRR(2023)
摘要
Hamilton-Jacobi (HJ) partial differential equations (PDEs) have diverse applications spanning physics, optimal control, game theory, and imaging sciences. This research introduces a first-order optimization-based technique for HJ PDEs, which formulates the time-implicit update of HJ PDEs as saddle point problems. We remark that the saddle point formulation for HJ equations is aligned with the primal-dual formulation of optimal transport and potential mean-field games (MFGs). This connection enables us to extend MFG techniques and design numerical schemes for solving HJ PDEs. We employ the primal-dual hybrid gradient (PDHG) method to solve the saddle point problems, benefiting from the simple structures that enable fast computations in updates. Remarkably, the method caters to a broader range of Hamiltonians, encompassing non-smooth and spatiotemporally dependent cases. The approach's effectiveness is verified through various numerical examples in both one-dimensional and two-dimensional examples, such as quadratic and $L^1$ Hamiltonians with spatial and time dependence.
更多查看译文
关键词
gradient,primal-dual,time-implicit,hamilton-jacobi
AI 理解论文
溯源树
样例
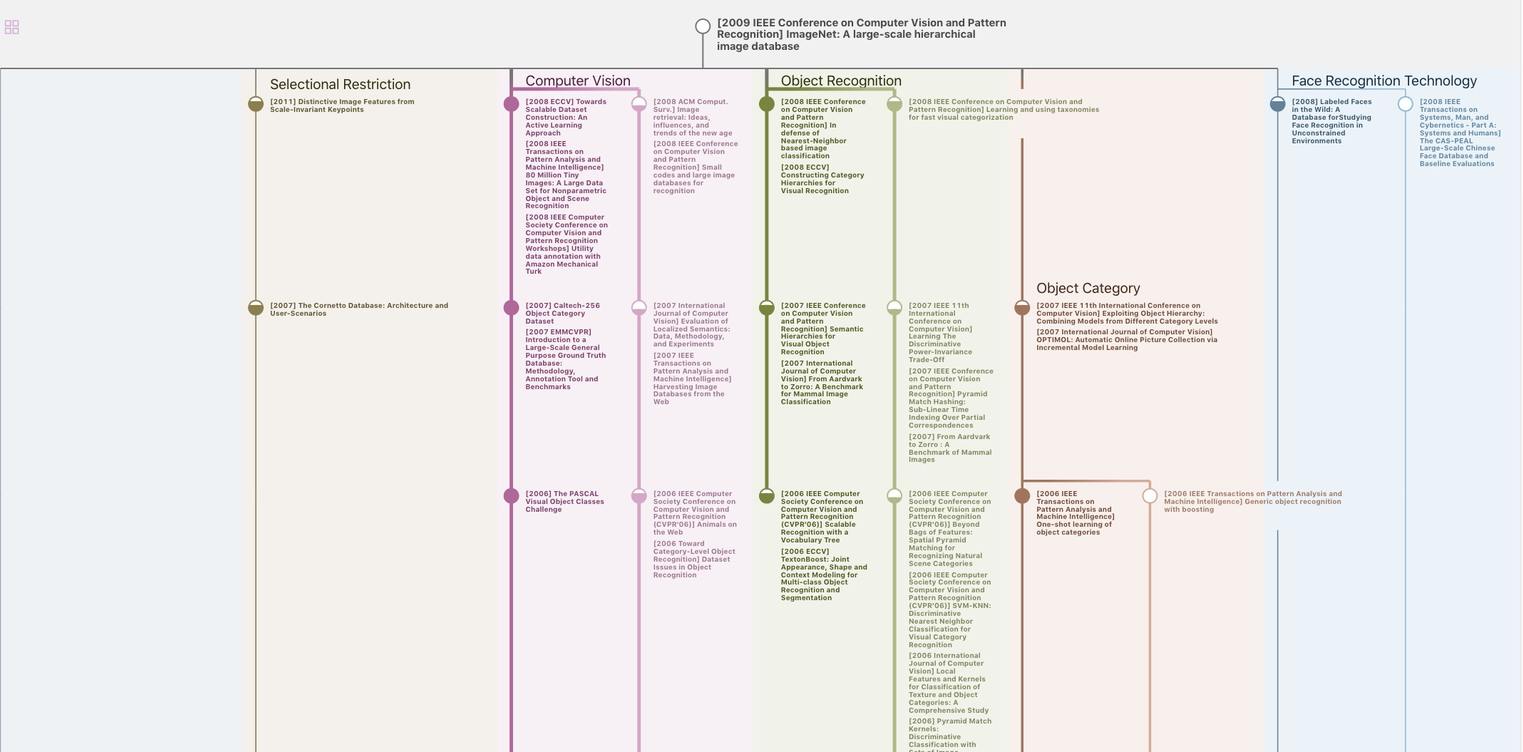
生成溯源树,研究论文发展脉络
Chat Paper
正在生成论文摘要