Zero-Shot Continuous Prompt Transfer: Generalizing Task Semantics Across Language Models
arXiv (Cornell University)(2023)
摘要
Prompt tuning in natural language processing (NLP) has become an increasingly popular method for adapting large language models to specific tasks. However, the transferability of these prompts, especially continuous prompts, between different models remains a challenge. In this work, we propose a zero-shot continuous prompt transfer method, where source prompts are encoded into relative space and the corresponding target prompts are searched for transferring to target models. Experimental results confirm the effectiveness of our method, showing that 'task semantics' in continuous prompts can be generalized across various language models. Moreover, we find that combining 'task semantics' from multiple source models can further enhance the generalizability of transfer.
更多查看译文
关键词
task semantics across
AI 理解论文
溯源树
样例
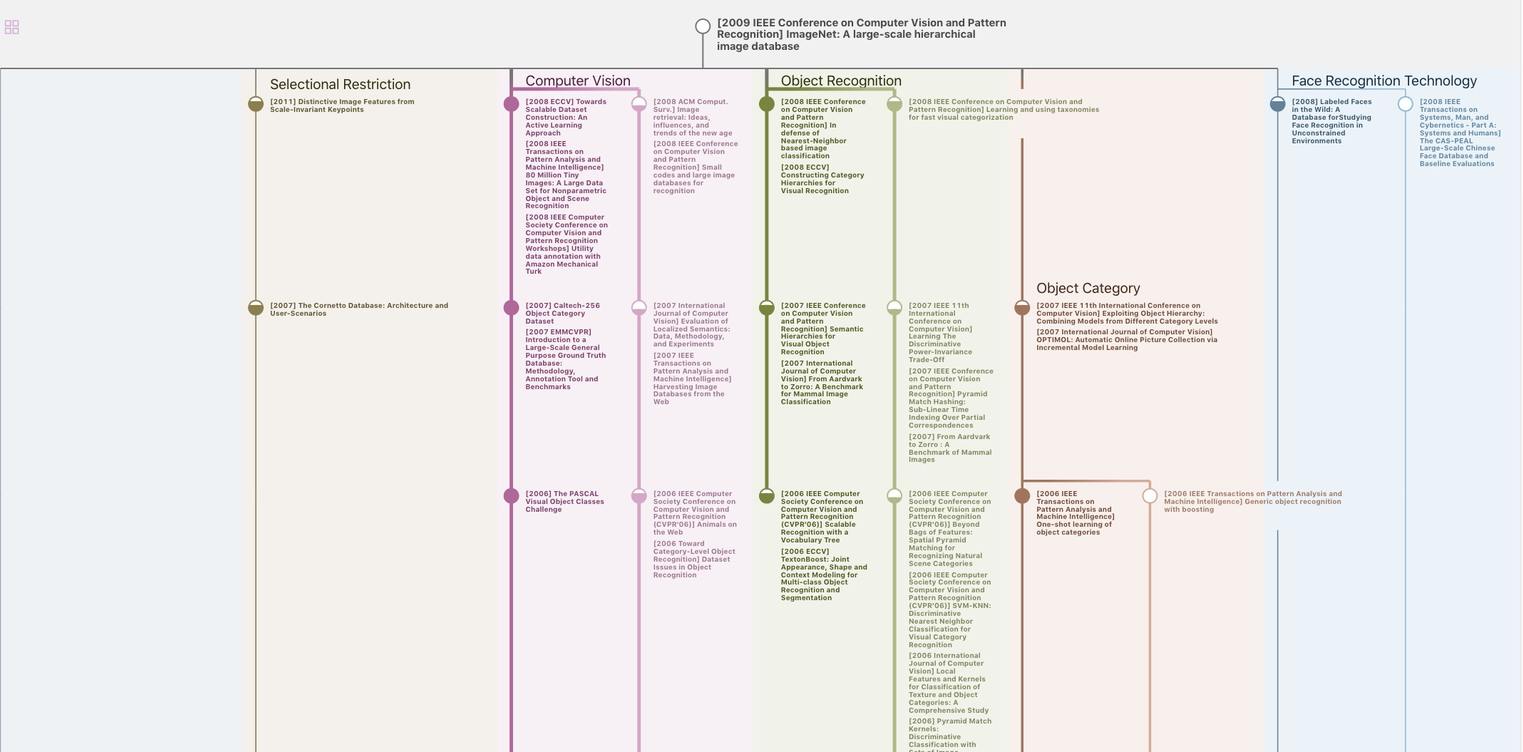
生成溯源树,研究论文发展脉络
Chat Paper
正在生成论文摘要