Federated Wasserstein Distance
ICLR 2024(2023)
摘要
We introduce a principled way of computing the Wasserstein distance between two distributions in a federated manner. Namely, we show how to estimate the Wasserstein distance between two samples stored and kept on different devices/clients whilst a central entity/server orchestrates the computations (again, without having access to the samples). To achieve this feat, we take advantage of the geometric properties of the Wasserstein distance -- in particular, the triangle inequality -- and that of the associated {\em geodesics}: our algorithm, FedWad (for Federated Wasserstein Distance), iteratively approximates the Wasserstein distance by manipulating and exchanging distributions from the space of geodesics in lieu of the input samples. In addition to establishing the convergence properties of FedWad, we provide empirical results on federated coresets and federate optimal transport dataset distance, that we respectively exploit for building a novel federated model and for boosting performance of popular federated learning algorithms.
更多查看译文
关键词
distance
AI 理解论文
溯源树
样例
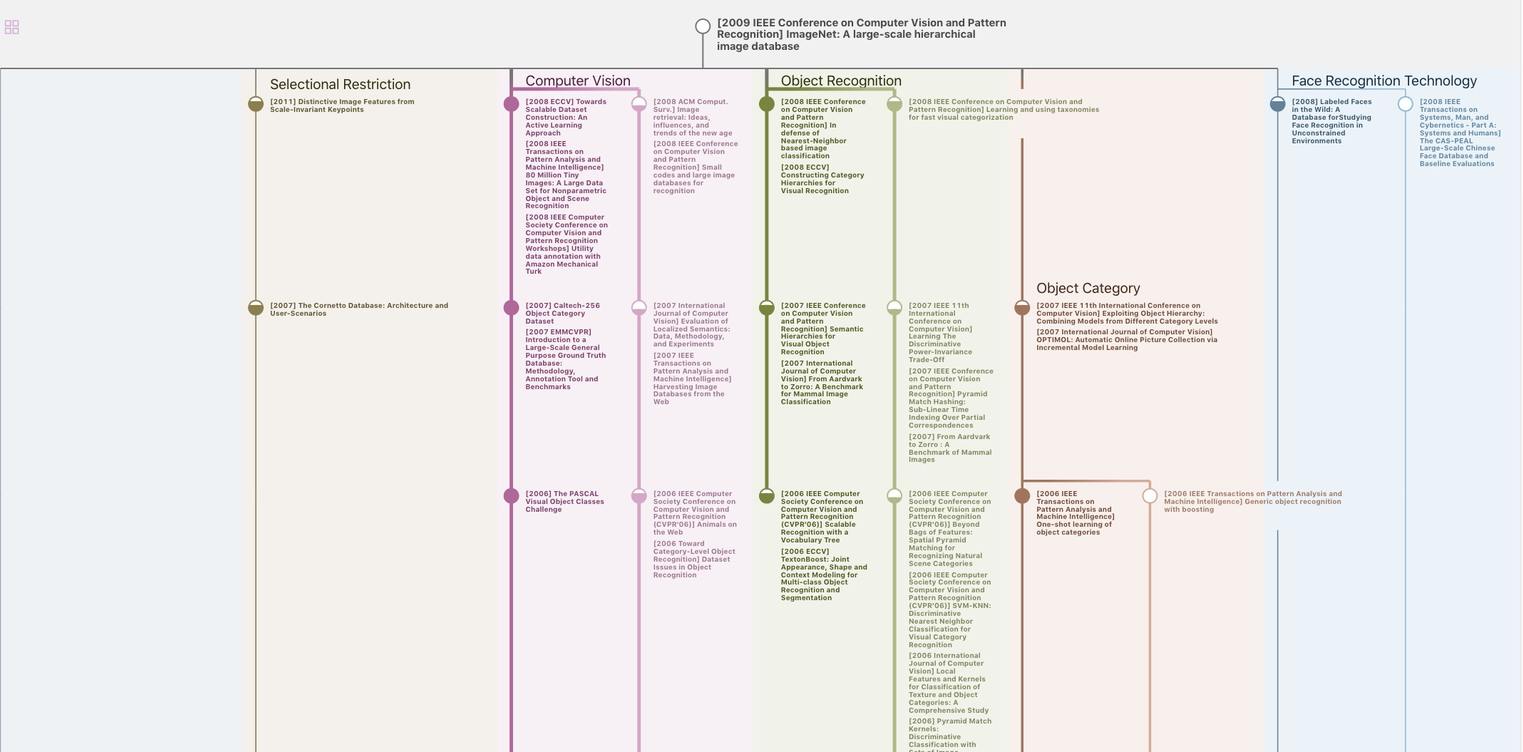
生成溯源树,研究论文发展脉络
Chat Paper
正在生成论文摘要