A framework for interpretation and testing of sparse canonical correlations
arXiv (Cornell University)(2023)
摘要
In clinical and biomedical research, multiple high-dimensional datasets are nowadays routinely collected from omics and imaging devices. Multivariate methods, such as Canonical Correlation Analysis (CCA), integrate two (or more) datasets to discover and understand underlying biological mechanisms. For an explorative method like CCA, interpretation is key. We present a sparse CCA method based on soft-thresholding that produces near-orthogonal components, allows for browsing over various sparsity levels, and permutation-based hypothesis testing. Our soft-thresholding approach avoids tuning of a penalty parameter. Such tuning is computationally burdensome and may render unintelligible results. In addition, unlike alternative approaches, our method is less dependent on the initialisation. We examined the performance of our approach with simulations and illustrated its use on real cancer genomics data from drug sensitivity screens. Moreover, we compared its performance to Penalised Matrix Analysis (PMA), which is a popular alternative of sparse CCA with a focus on yielding interpretable results. Compared to PMA, our method offers improved interpretability of the results, while not compromising, or even improving, signal discovery. he software and simulation framework are available at https://github.com/nuria-sv/toscca.
更多查看译文
关键词
correlations
AI 理解论文
溯源树
样例
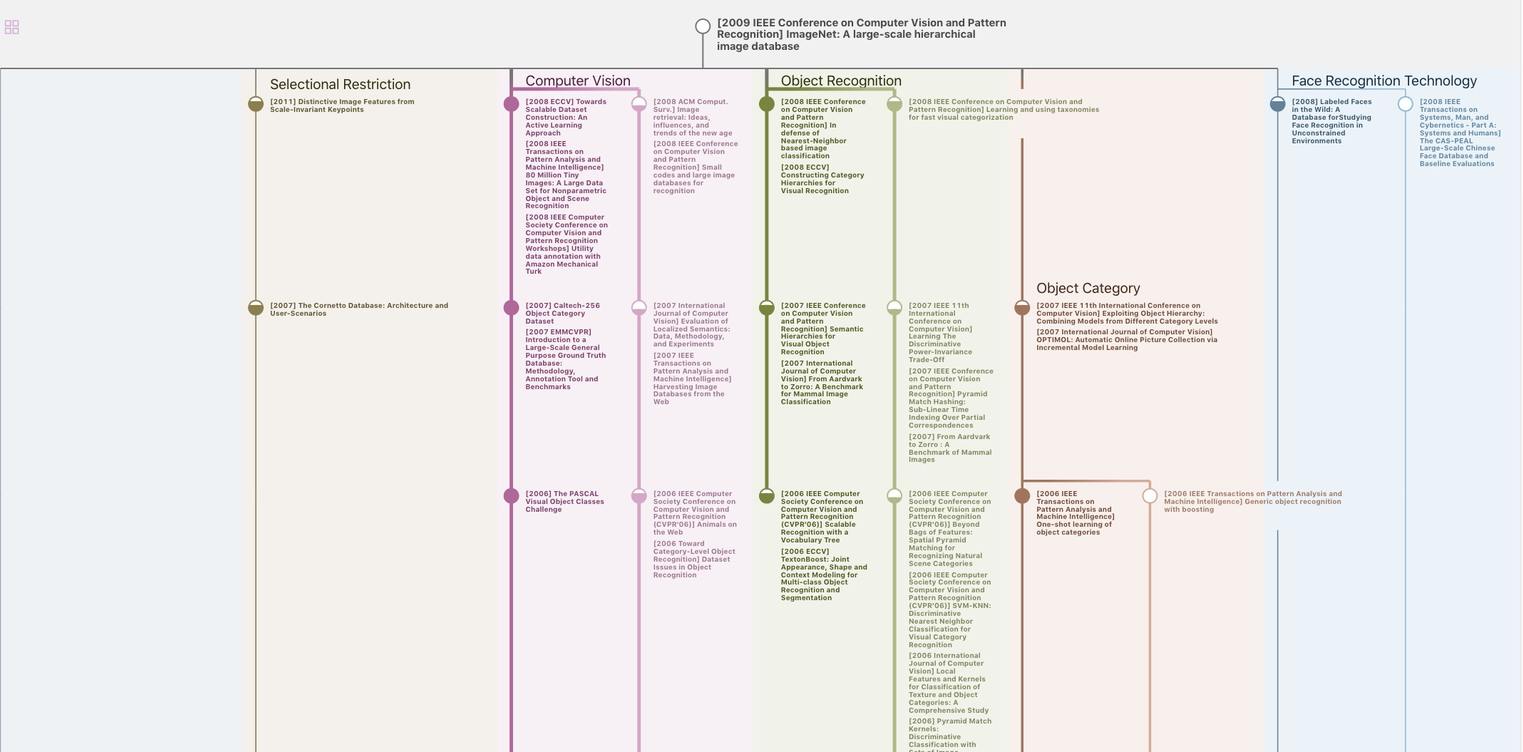
生成溯源树,研究论文发展脉络
Chat Paper
正在生成论文摘要