Soft Actor-Critic-Based Computation Offloading in Multiuser MEC-Enabled IoT-A Lifetime Maximization Perspective
IEEE Internet of Things Journal(2023)
摘要
This article studies the network lifetime optimization problem in a multiuser mobile-edge computing (MEC)-enabled Internet of Things (IoT) system comprising an access point (AP), a MEC server, and a set of K mobile devices (MDs) with limited battery capacity. Considering the residual battery energy at the MDs, stochastic task arrivals, and time-varying wireless fading channels, a soft actor-critic (SAC)-based deep reinforcement learning (DRL) lifetime maximization, called DeepLM, is proposed to jointly optimize the task splitting ratio, the local CPU-cycle frequencies at the MDs, the bandwidth allocation, and the CPU-cycle frequency allocation at the MEC server subject to the task queuing backlogs constraint, the bandwidth constraint, and maximum CPU-cycle frequency constraints at the MDs and the MEC server. Our results reveal that DeepLM enjoys a fast convergence rate and a small oscillation amplitude. We also compare the performance of DeepLM with three benchmark offloading schemes, namely, fully edge computing (FEC), fully local computing (FLC), and random computation offloading (RCO). DeepLM increases the network lifetime by 496% and 229% compared to the FLC and RCO schemes. Interestingly, it achieves such a colossal lifetime improvement when its nonbacklog probability is 0.99, while that of FEC, FLC, and RCO is 0.69, 0.53, and 0.25, respectively, showing a significant performance gain of 30%, 46%, and 74%.
更多查看译文
关键词
Deep reinforcement learning (DRL),Internet of Things (IoT),lifetime maximization,mobile-edge computing (MEC),soft actor-critic (SAC)
AI 理解论文
溯源树
样例
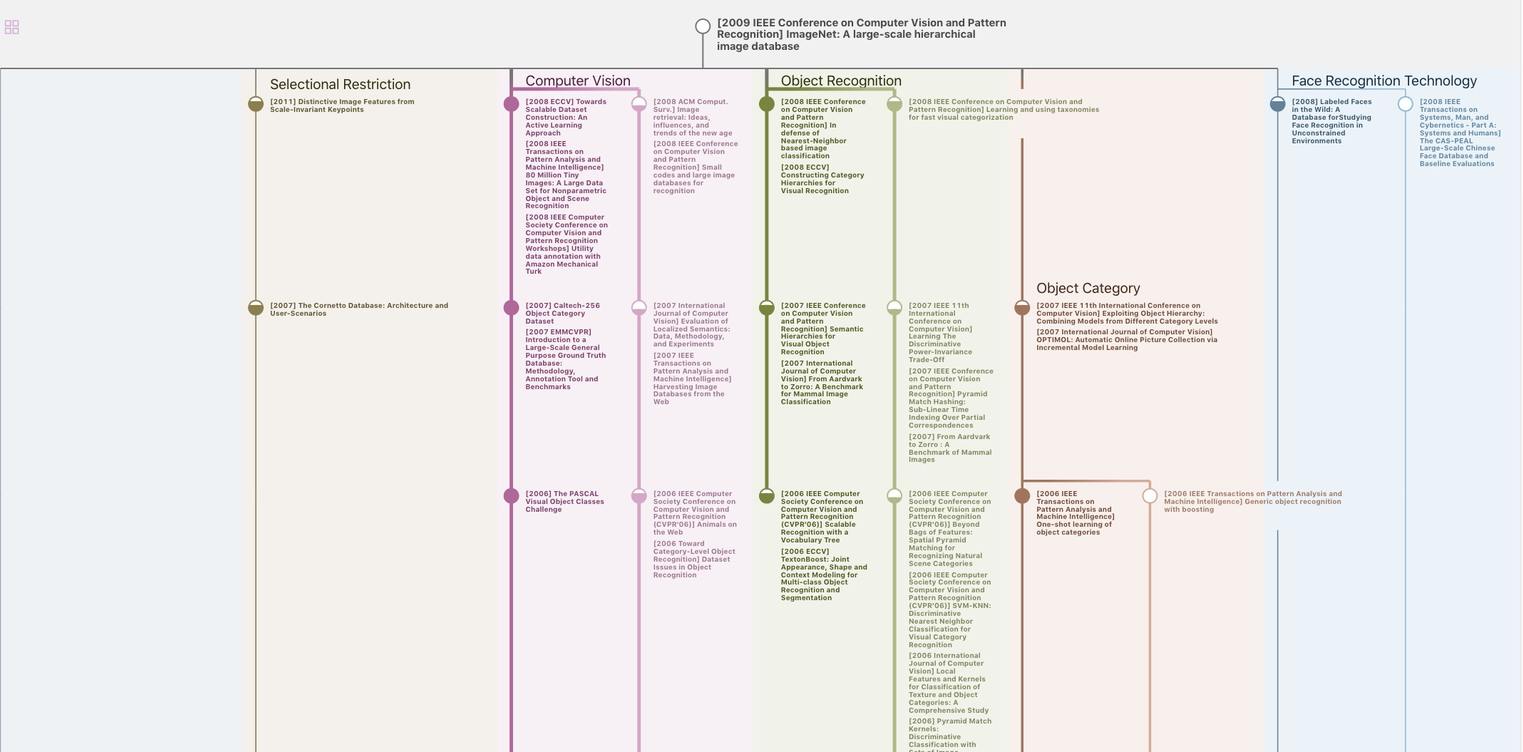
生成溯源树,研究论文发展脉络
Chat Paper
正在生成论文摘要