A Model-Agnostic Meta-Learning Fault Diagnosis Method Based on Dynamic Weighting
2023 IEEE PELS Students and Young Professionals Symposium (SYPS)(2023)
Abstract
Motor is an important component in industrial production, so it is very important to detect and diagnose the motor fault in time. The performance of traditional deep learning methods is often limited by the amount of data, which leads to the problem of unreliable model performance in the face of few shot fault diagnosis problem. Meta-learning can use previous few shot learning experience to quickly guide a new few shot problem to build a reliable model. However, traditional meta-learning methods only focus on training the meta model by accumulating loss functions for different tasks, without considering the specific contribution from different tasks. Therefore, when some data is polluted by noise, the construction of meta model may be affected, thus affect the accuracy of fault diagnosis. Therefore, this paper aims to propose a meta-learning fault diagnosis method based on dynamic weighting to overcome the above problems. Different weights are assigned according to the size of the training tasks’ loss function, and smaller weights are assigned to tasks with larger loss functions, so as to realize dynamic weakening of the negative effects of unreliable tasks and build a meta model that can quickly adapt to powerful fault diagnosis performance. Experimental verification for motor fault diagnosis shows that the fault diagnosis accuracy of this method is improved significantly.
MoreTranslated text
Key words
deep learning,fault diagnosis,meta-learning,noise pollution
求助PDF
上传PDF
View via Publisher
AI Read Science
AI Summary
AI Summary is the key point extracted automatically understanding the full text of the paper, including the background, methods, results, conclusions, icons and other key content, so that you can get the outline of the paper at a glance.
Example
Background
Key content
Introduction
Methods
Results
Related work
Fund
Key content
- Pretraining has recently greatly promoted the development of natural language processing (NLP)
- We show that M6 outperforms the baselines in multimodal downstream tasks, and the large M6 with 10 parameters can reach a better performance
- We propose a method called M6 that is able to process information of multiple modalities and perform both single-modal and cross-modal understanding and generation
- The model is scaled to large model with 10 billion parameters with sophisticated deployment, and the 10 -parameter M6-large is the largest pretrained model in Chinese
- Experimental results show that our proposed M6 outperforms the baseline in a number of downstream tasks concerning both single modality and multiple modalities We will continue the pretraining of extremely large models by increasing data to explore the limit of its performance
Upload PDF to Generate Summary
Must-Reading Tree
Example
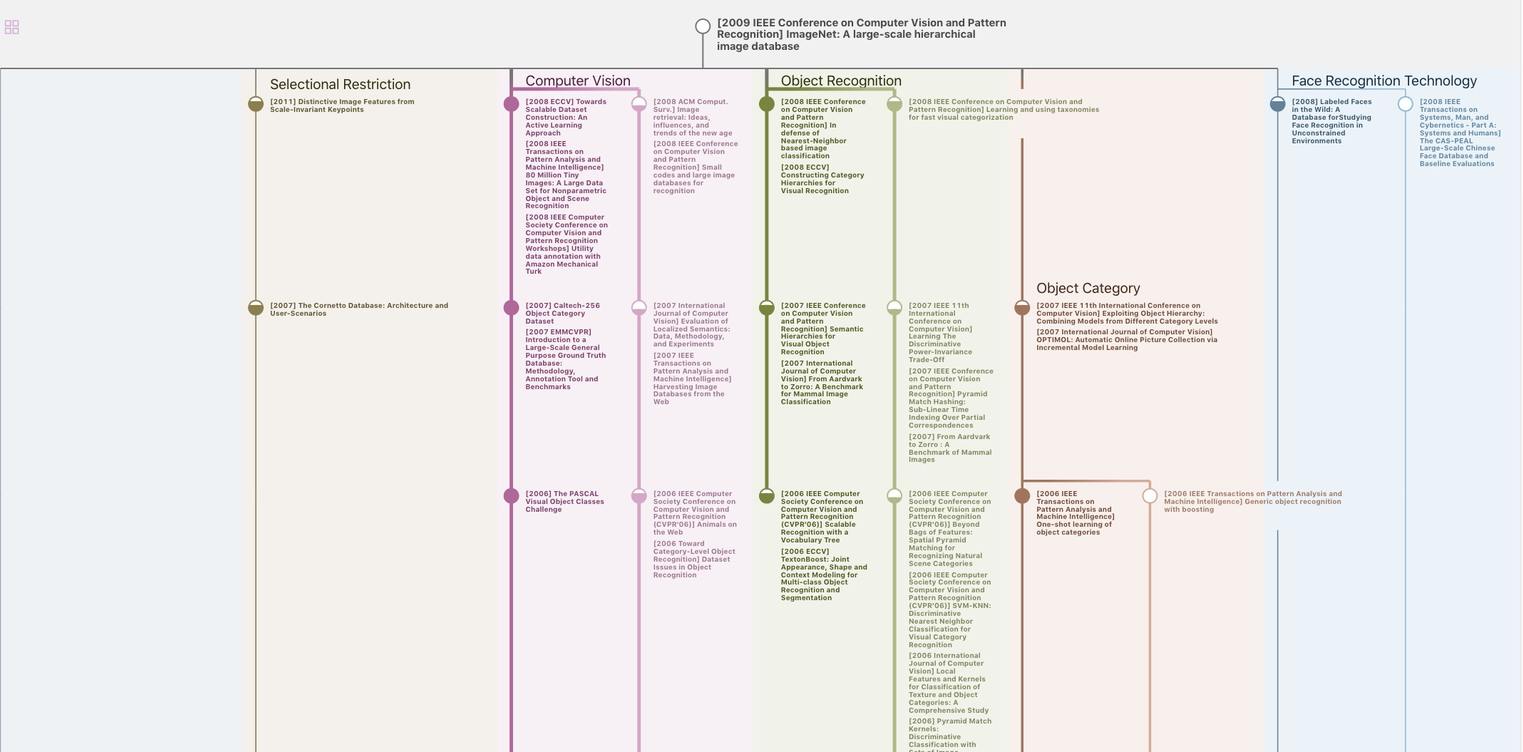
Generate MRT to find the research sequence of this paper
Related Papers
2015
被引用1485 | 浏览
2019
被引用83 | 浏览
2019
被引用140 | 浏览
2022
被引用73 | 浏览
2022
被引用9 | 浏览
Data Disclaimer
The page data are from open Internet sources, cooperative publishers and automatic analysis results through AI technology. We do not make any commitments and guarantees for the validity, accuracy, correctness, reliability, completeness and timeliness of the page data. If you have any questions, please contact us by email: report@aminer.cn
Chat Paper