Jailbreaker in Jail: Moving Target Defense for Large Language Models.
CoRR(2023)
Abstract
Large language models (LLMs), known for their capability in understanding and following instructions, are vulnerable to adversarial attacks. Researchers have found that current commercial LLMs either fail to be "harmless" by presenting unethical answers, or fail to be "helpful" by refusing to offer meaningful answers when faced with adversarial queries. To strike a balance between being helpful and harmless, we design a moving target defense (MTD) enhanced LLM system. The system aims to deliver non-toxic answers that align with outputs from multiple model candidates, making them more robust against adversarial attacks. We design a query and output analysis model to filter out unsafe or non-responsive answers. %to achieve the two objectives of randomly selecting outputs from different LLMs. We evaluate over 8 most recent chatbot models with state-of-the-art adversarial queries. Our MTD-enhanced LLM system reduces the attack success rate from 37.5\% to 0\%. Meanwhile, it decreases the response refusal rate from 50\% to 0\%.
MoreTranslated text
Key words
jailbreaker,jailbreaker,target defense,language,models
AI Read Science
Must-Reading Tree
Example
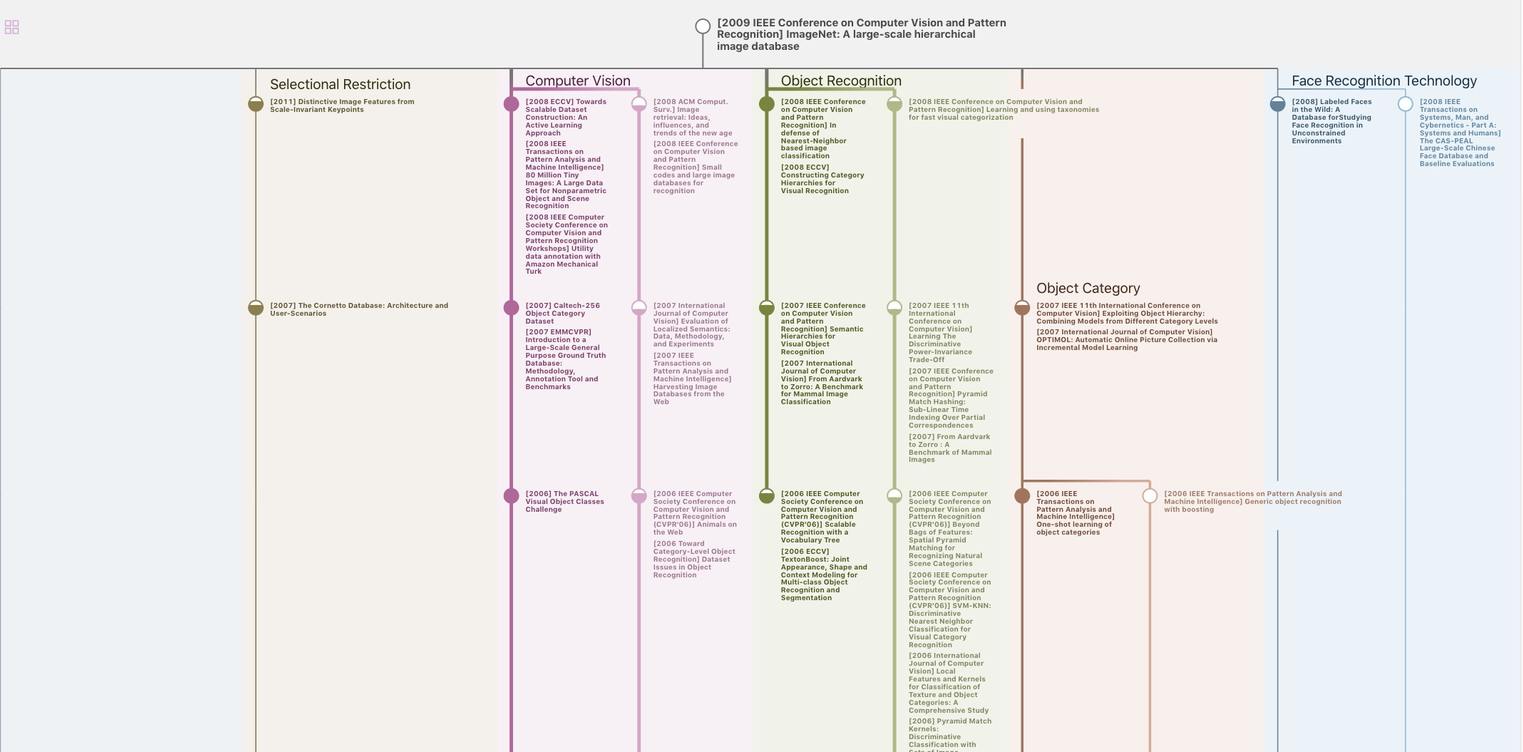
Generate MRT to find the research sequence of this paper
Chat Paper
Summary is being generated by the instructions you defined