DON-LSTM: Multi-Resolution Learning with DeepONets and Long Short-Term Memory Neural Networks
CoRR(2023)
摘要
Deep operator networks (DeepONets, DONs) offer a distinct advantage over traditional neural networks in their ability to be trained on multi-resolution data. This property becomes especially relevant in real-world scenarios where high-resolution measurements are difficult to obtain, while low-resolution data is more readily available. Nevertheless, DeepONets alone often struggle to capture and maintain dependencies over long sequences compared to other state-of-the-art algorithms. We propose a novel architecture, named DON-LSTM, which extends the DeepONet with a long short-term memory network (LSTM). Combining these two architectures, we equip the network with explicit mechanisms to leverage multi-resolution data, as well as capture temporal dependencies in long sequences. We test our method on long-time-evolution modeling of multiple non-linear systems and show that the proposed multi-resolution DON-LSTM achieves significantly lower generalization error and requires fewer high-resolution samples compared to its vanilla counterparts.
更多查看译文
关键词
memory neural networks,deeponets,learning,don-lstm,multi-resolution,short-term
AI 理解论文
溯源树
样例
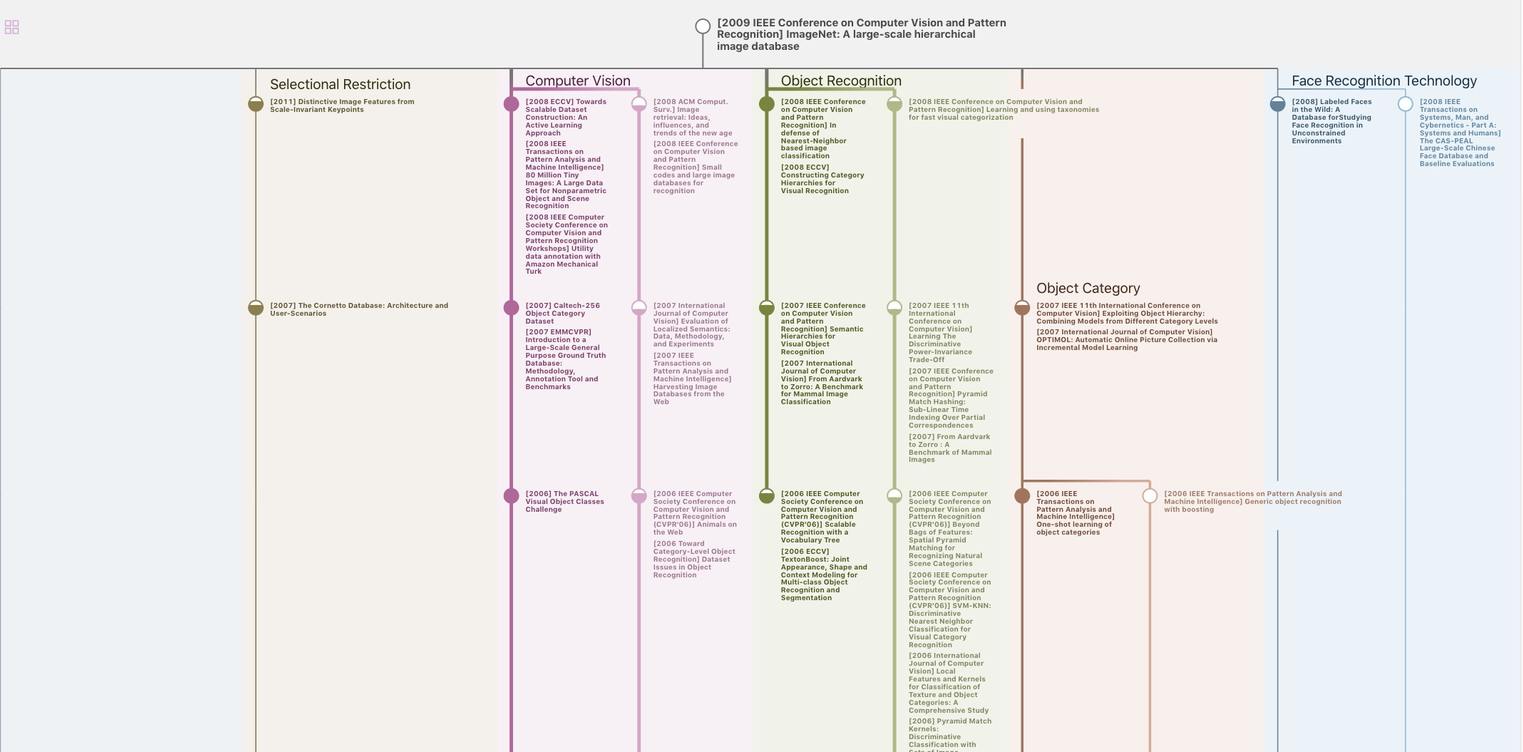
生成溯源树,研究论文发展脉络
Chat Paper
正在生成论文摘要