Identifying Physical Structures in Our Galaxy with Gaussian Mixture Models: An Unsupervised Machine Learning Technique
ASTROPHYSICAL JOURNAL(2023)
摘要
We explore the potential of the Gaussian mixture model (GMM), an unsupervised machine-learning method, to identify coherent physical structures in the interstellar medium. The implementation we present can be used on any kind of spatially and spectrally resolved data set. We provide a step-by-step guide to use these models on different sources and data sets. Following the guide, we run the models on NGC 1977, RCW 120, and RCW 49 using the [C ii] 158 mu m mapping observations from the SOFIA telescope. We find that the models identified six, four, and five velocity coherent physical structures in NGC 1977, RCW 120, and RCW 49, respectively, which are validated by analyzing the observed spectra toward these structures and by comparison to earlier findings. In this work we demonstrate that GMM is a powerful tool that can better automate the process of spatial and spectral analysis to interpret mapping observations.
更多查看译文
AI 理解论文
溯源树
样例
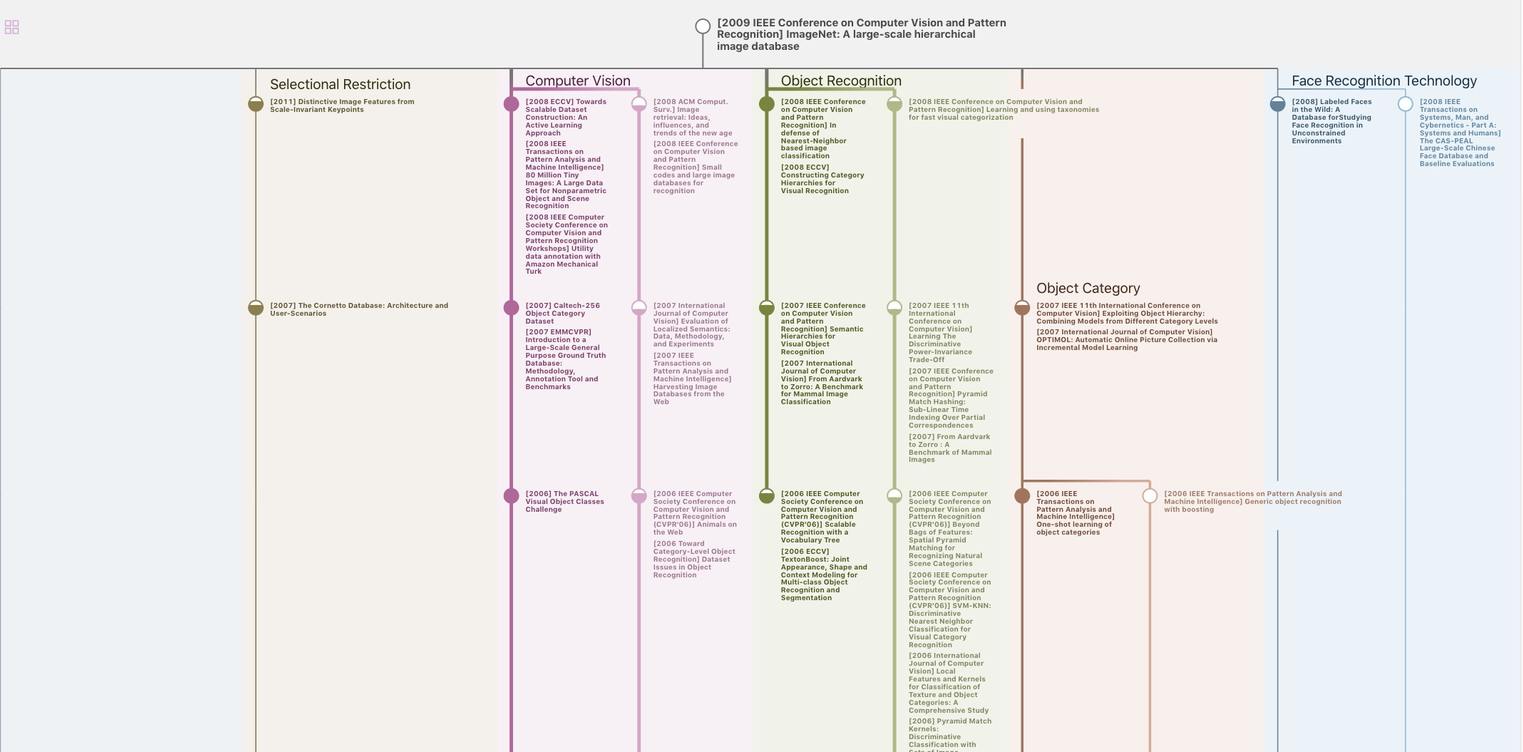
生成溯源树,研究论文发展脉络
Chat Paper
正在生成论文摘要