High-dimensional SGD Aligns with Emerging Outlier Eigenspaces
ICLR 2024(2024)
关键词
stochastic gradient descent,Hessian,multi-layer neural networks,high-dimensional classification,Gaussian mixture model,XOR problem
AI 理解论文
溯源树
样例
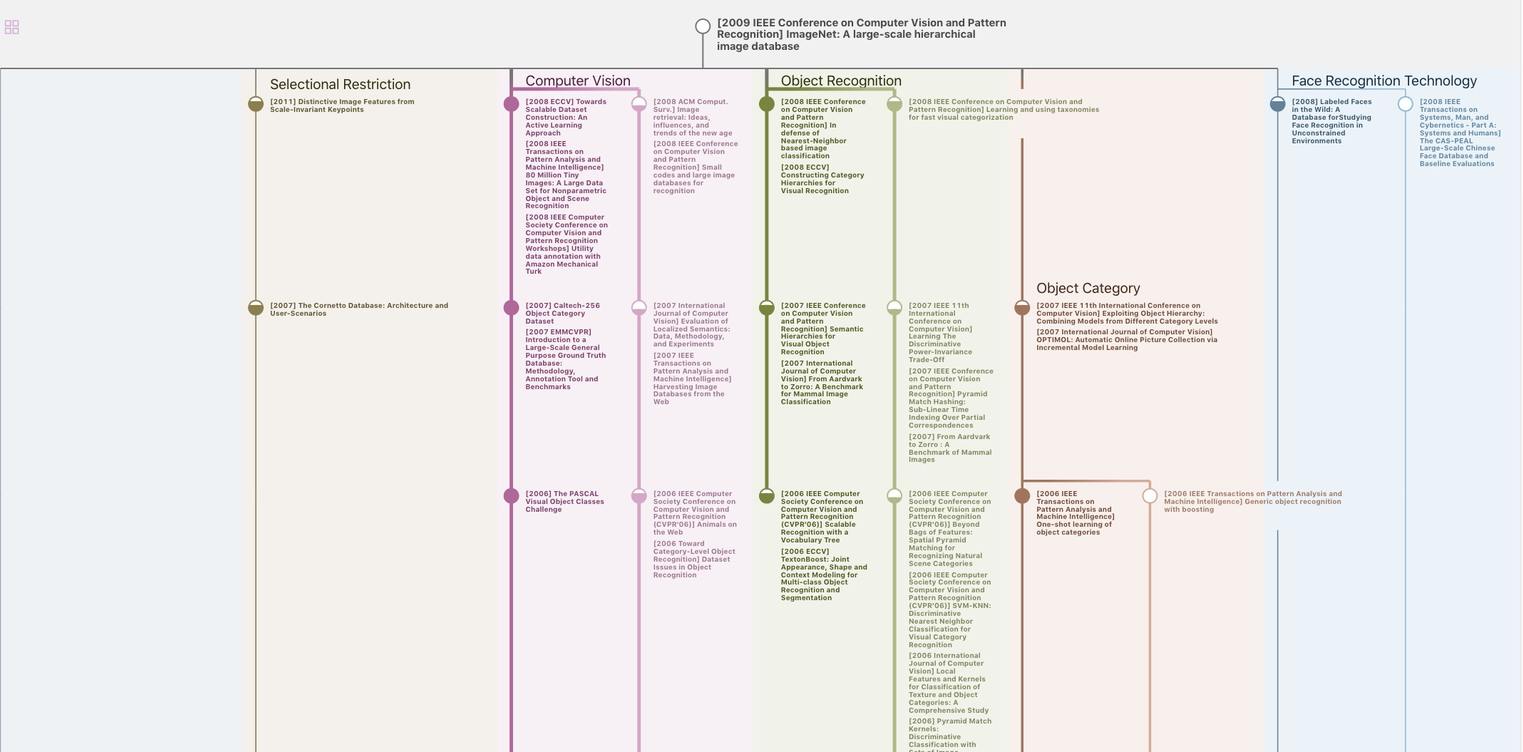
生成溯源树,研究论文发展脉络
Chat Paper
正在生成论文摘要