Accelerating the prediction of CO 2 capture at low partial pressures in metal-organic frameworks using new machine learning descriptors
Communications chemistry(2023)
摘要
Metal-Organic frameworks (MOFs) have been considered for various gas storage and separation applications. Theoretically, there are an infinite number of MOFs that can be created; however, a finite amount of resources are available to evaluate each one. Computational methods can be adapted to expedite the process of evaluation. In the context of CO 2 capture, this paper investigates the method of screening MOFs using machine learning trained on molecular simulation data. New descriptors are introduced to aid this process. Using all descriptors, it is shown that machine learning can predict the CO 2 adsorption, with an R 2 of above 0.9. The introduced Effective Point Charge (EPoCh) descriptors, which assign values to frameworks’ partial charges based on the expected CO 2 uptake of an equivalent point charge in isolation, are shown to be the second most important group of descriptors, behind the Henry coefficient. Furthermore, the EPoCh descriptors are hundreds of thousands of times faster to obtain compared with the Henry coefficient, and they achieve similar results when identifying top candidates for CO 2 capture using pseudo-classification predictions.
更多查看译文
关键词
co2 capture,machine learning,low partial pressures,metal-organic
AI 理解论文
溯源树
样例
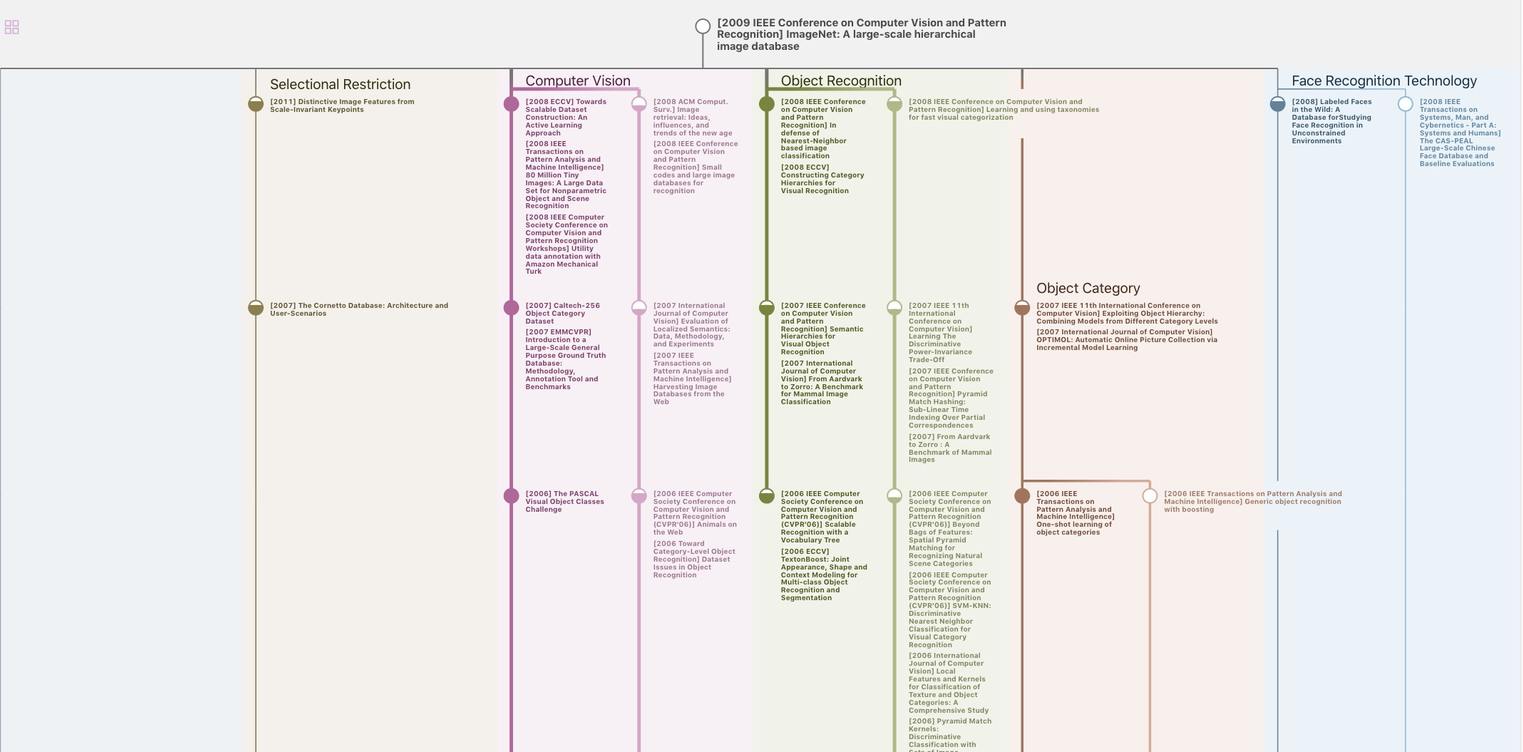
生成溯源树,研究论文发展脉络
Chat Paper
正在生成论文摘要