INVADEseq to identify cell-adherent or invasive bacteria and the associated host transcriptome at single-cell-level resolution
Nature protocols(2023)
摘要
Single-cell RNA sequencing (scRNAseq) technologies have been beneficial in revealing and describing cellular heterogeneity within mammalian tissues, including solid tumors. However, many of these techniques apply poly(A) selection of RNA, and thus have primarily focused on determining the gene signatures of eukaryotic cellular components of the tumor microenvironment. Microbiome analysis has revealed the presence of microbial ecosystems, including bacteria and fungi, within human tumor tissues from major cancer types. Imaging data have revealed that intratumoral bacteria may be located within epithelial and immune cell types. However, as bacterial RNA typically lacks a poly(A) tail, standard scRNAseq approaches have limited ability to capture this microbial component of the tumor microenvironment. To overcome this, we describe the invasion–adhesion-directed expression sequencing (INVADEseq) approach, whereby we adapt 10x Genomics 5′ scRNAseq protocol by introducing a primer that targets a conserved region of the bacterial 16S ribosomal RNA gene in addition to the standard primer for eukaryotic poly(A) RNA selection. This ‘add-on’ approach enables the generation of eukaryotic and bacterial DNA libraries at eukaryotic single-cell level resolution, utilizing the 10x barcode to identify single cells with intracellular bacteria. The INVADEseq method takes 30 h to complete, including tissue processing, sequencing and computational analysis. As an output, INVADEseq has shown to be a reliable tool in human cancer cell lines and patient tumor specimens by detecting the proportion of human cells that harbor bacteria and the identities of human cells and intracellular bacteria, along with identifying host transcriptional programs that are modulated on the basis of associated bacteria.
更多查看译文
关键词
associated host transcriptome,invasive bacteria,cell-adherent,single-cell-level
AI 理解论文
溯源树
样例
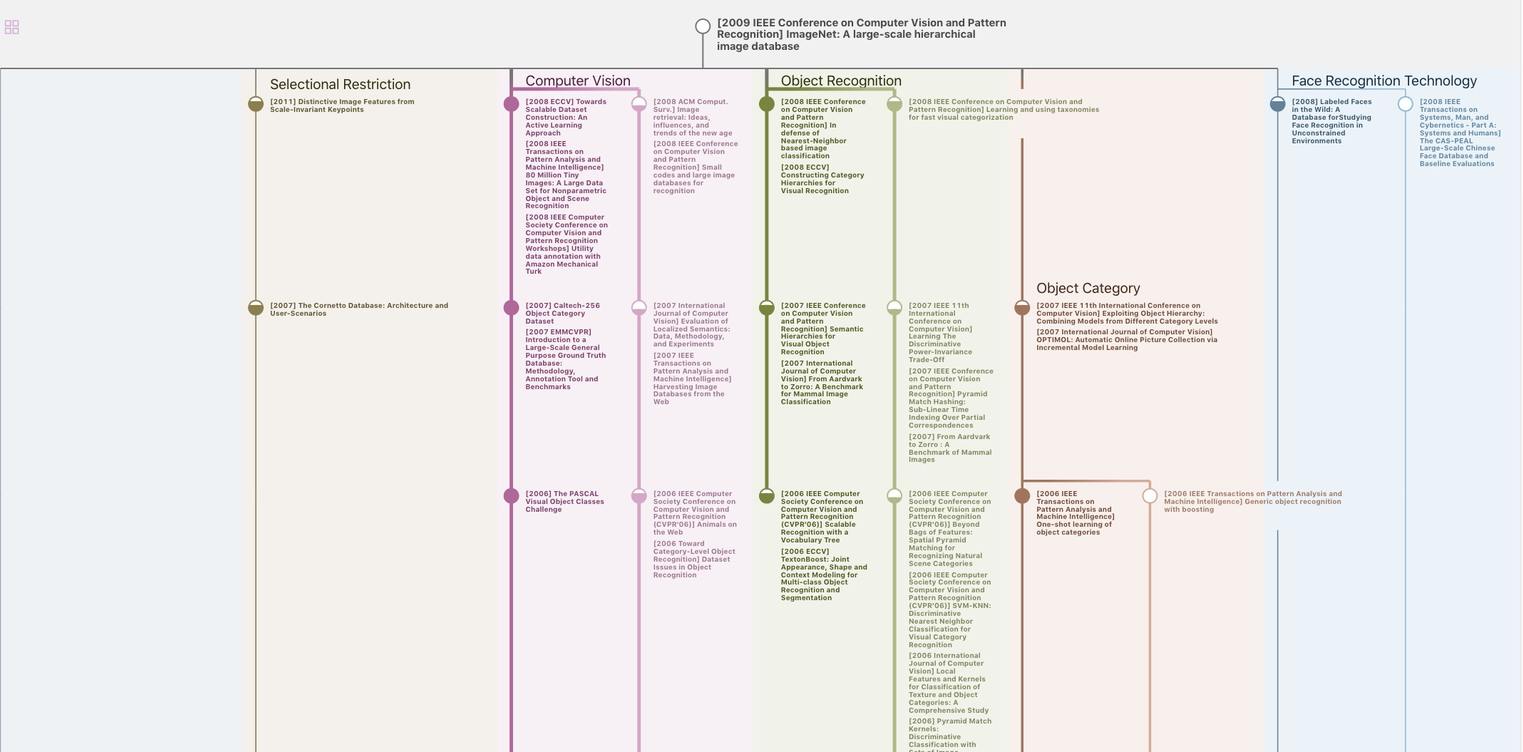
生成溯源树,研究论文发展脉络
Chat Paper
正在生成论文摘要