Fine-Grained Road Quality Monitoring Using Deep Learning
IEEE Transactions on Intelligent Transportation Systems(2023)
摘要
Existing solutions for road surface monitoring have assumed that events (both normal and abnormal) have a defined duration, and these methods fail to provide a universal framework that can be used to assess the quality of roads in real-world scenarios where events do not have to be of fixed duration. This article aims to improve road quality assessment systems by overcoming the constraint of fixed window size and taking into account the real-world scenario of variable-length events. First, we annotate a big heterogeneous data set without partitioning it into fixed-size windows. Second, we suggest two distinct approaches for detecting and characterizing anomalies utilizing deep learning architectures comprising Bi-Directional LSTM units. The first strategy is sequence classification (using a many to one correspondence to classify the entire sequence), and the second approach is endpoint detection (classify each time step as a normal or anomalous event using a many to many approach). The solutions presented in this article are intended for use with non-anomalous (normal) signals as well as with four distinct types of anomalies: cat-eyes, manholes, potholes, and speed bumps. Our sequence classification model (Bi-LSTM model) is capable of detecting anomalies with a 97.3% True Positive Rate when the anomalies are considered a positive class. On the other hand, our end-point detection framework is able to mark the exact end-point of anomalous signals with a true positive rate of 90.2% as shown in II. Our dataset and annotation are publicly available at:
https://drive.google.com/drive/folders/
1Qf-4D6P9Oeu-yw3yc3UY55V7wDCWiumI
更多查看译文
关键词
deep learning,road,fine-grained
AI 理解论文
溯源树
样例
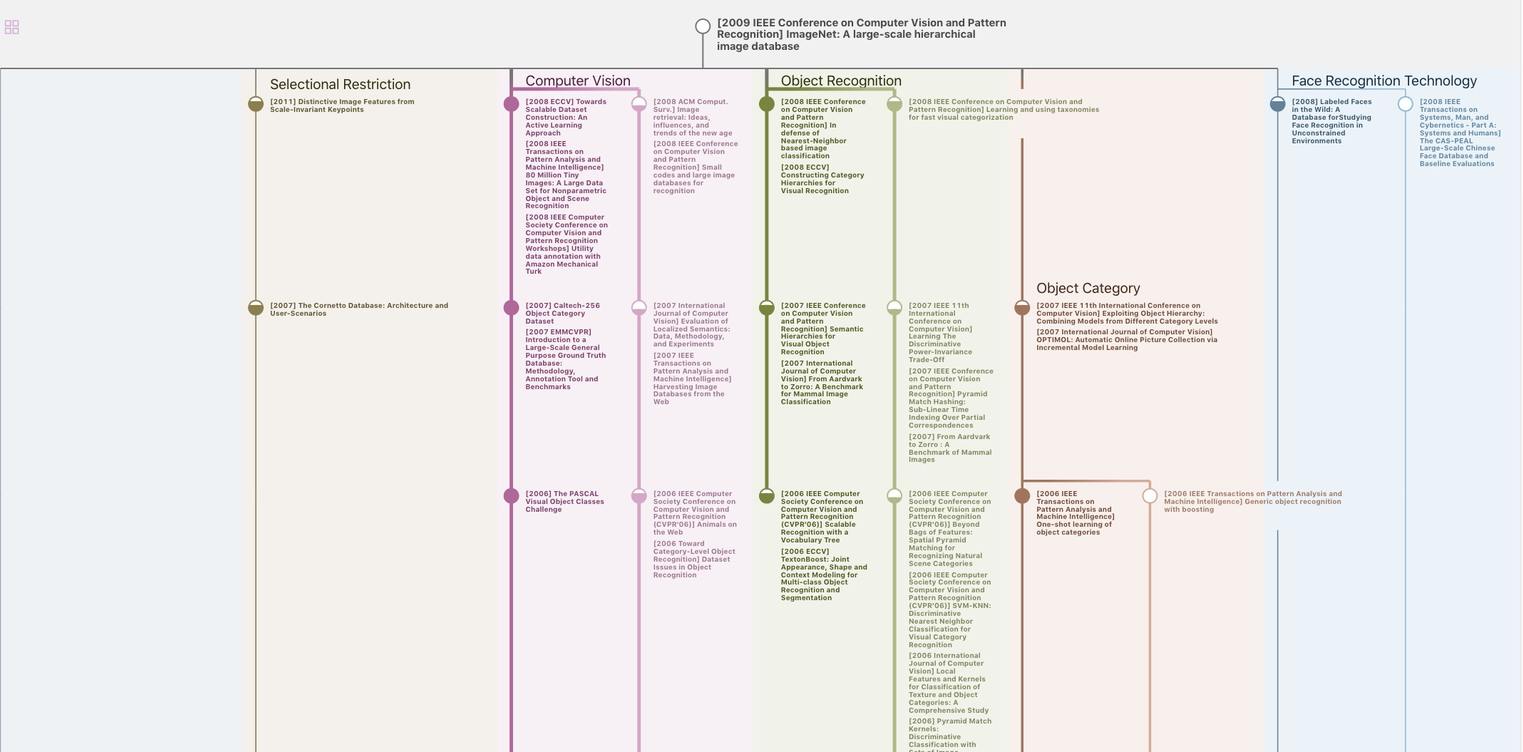
生成溯源树,研究论文发展脉络
Chat Paper
正在生成论文摘要