Learning Energy-Based Prior Model with Diffusion-Amortized MCMC
NeurIPS(2023)
摘要
Latent space Energy-Based Models (EBMs), also known as energy-based priors, have drawn growing interests in the field of generative modeling due to its flexibility in the formulation and strong modeling power of the latent space. However, the common practice of learning latent space EBMs with non-convergent short-run MCMC for prior and posterior sampling is hindering the model from further progress; the degenerate MCMC sampling quality in practice often leads to degraded generation quality and instability in training, especially with highly multi-modal and/or high-dimensional target distributions. To remedy this sampling issue, in this paper we introduce a simple but effective diffusion-based amortization method for long-run MCMC sampling and develop a novel learning algorithm for the latent space EBM based on it. We provide theoretical evidence that the learned amortization of MCMC is a valid long-run MCMC sampler. Experiments on several image modeling benchmark datasets demonstrate the superior performance of our method compared with strong counterparts
更多查看译文
关键词
prior model,learning,energy-based,diffusion-amortized
AI 理解论文
溯源树
样例
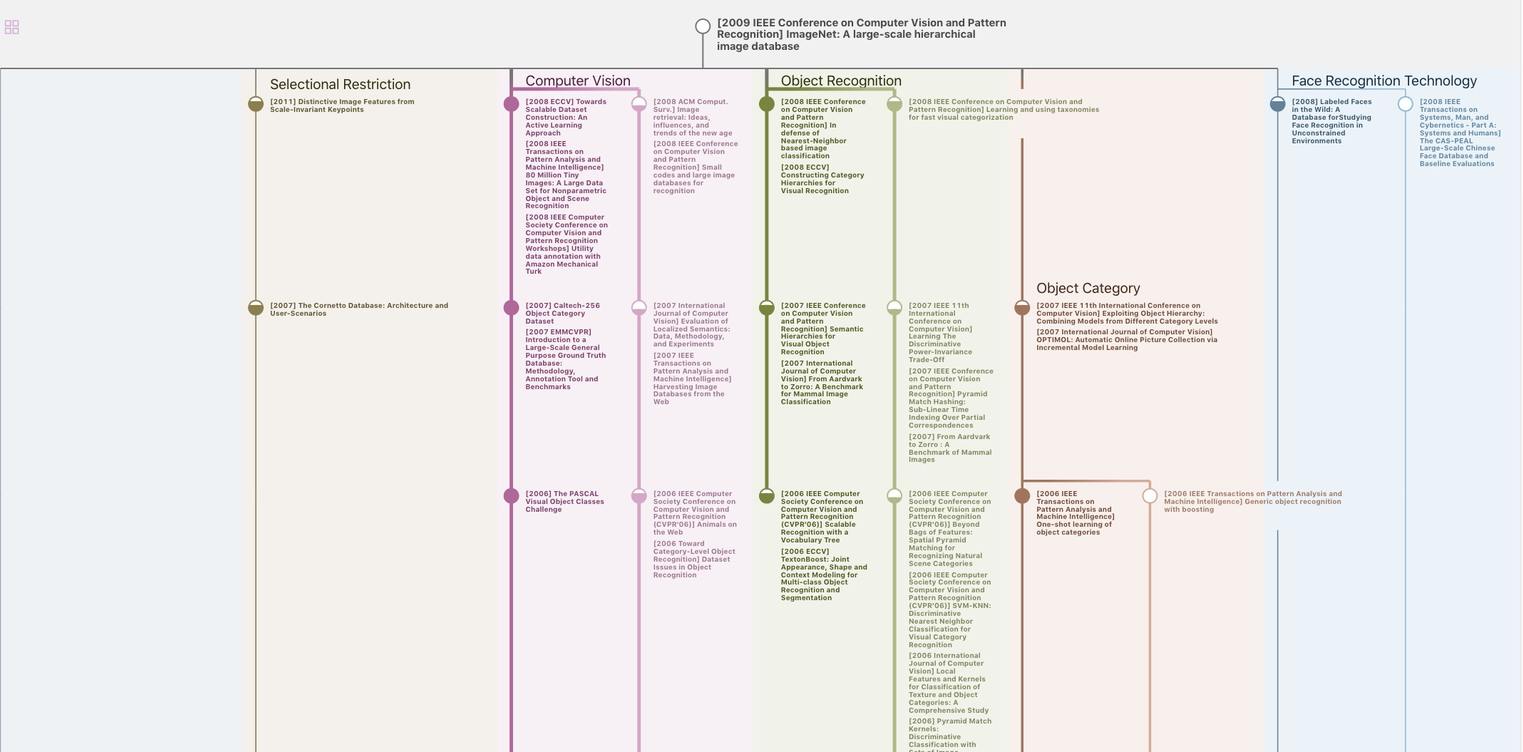
生成溯源树,研究论文发展脉络
Chat Paper
正在生成论文摘要