Learning to Simplify Spatial-Temporal Graphs in Gait Analysis.
CoRR(2023)
摘要
Gait analysis leverages unique walking patterns for person identification and assessment across multiple domains. Among the methods used for gait analysis, skeleton-based approaches have shown promise due to their robust and interpretable features. However, these methods often rely on hand-crafted spatial-temporal graphs that are based on human anatomy disregarding the particularities of the dataset and task. This paper proposes a novel method to simplify the spatial-temporal graph representation for gait-based gender estimation, improving interpretability without losing performance. Our approach employs two models, an upstream and a downstream model, that can adjust the adjacency matrix for each walking instance, thereby removing the fixed nature of the graph. By employing the Straight-Through Gumbel-Softmax trick, our model is trainable end-to-end. We demonstrate the effectiveness of our approach on the CASIA-B dataset for gait-based gender estimation. The resulting graphs are interpretable and differ qualitatively from fixed graphs used in existing models. Our research contributes to enhancing the explainability and task-specific adaptability of gait recognition, promoting more efficient and reliable gait-based biometrics.
更多查看译文
关键词
graphs,learning,spatial-temporal
AI 理解论文
溯源树
样例
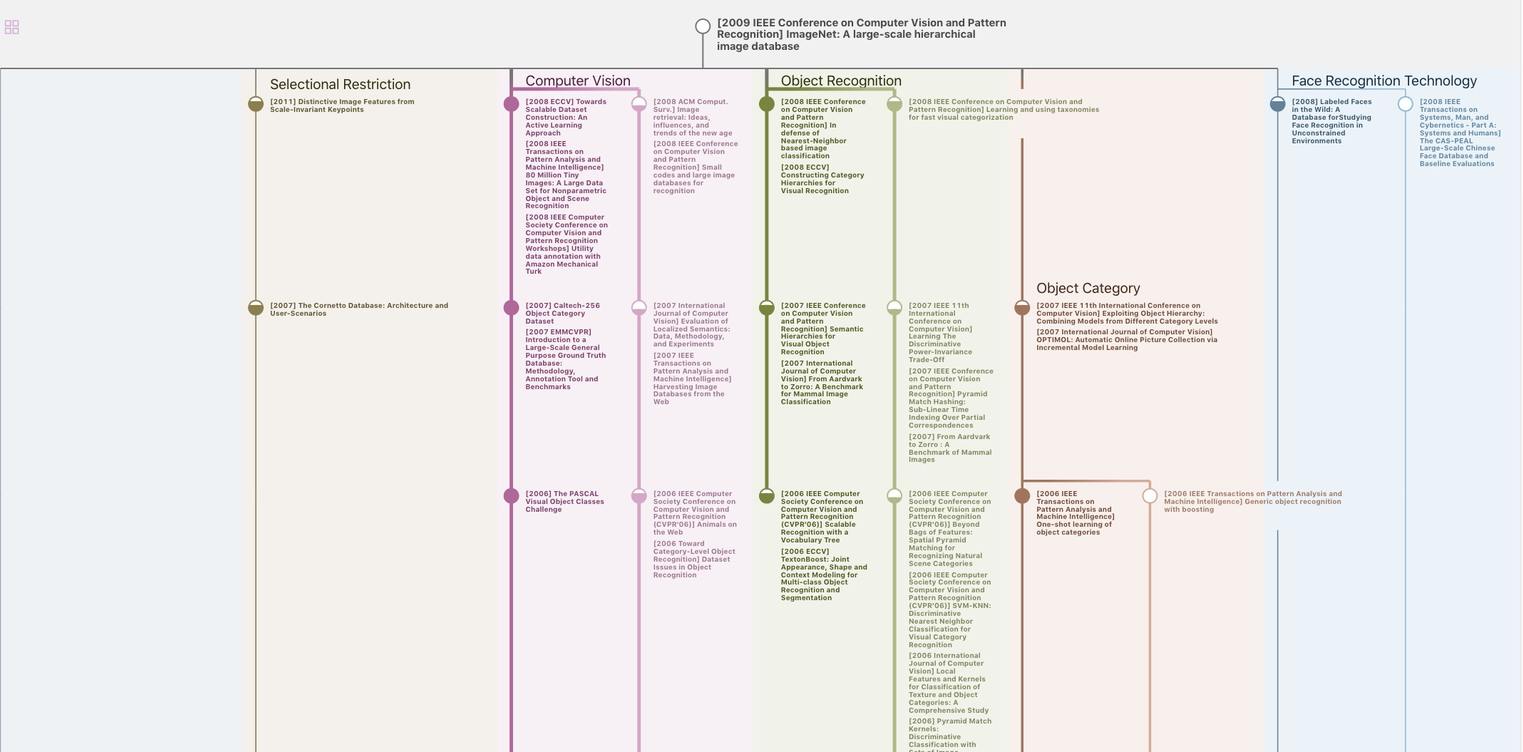
生成溯源树,研究论文发展脉络
Chat Paper
正在生成论文摘要