Interpolating between Clustering and Dimensionality Reduction with Gromov-Wasserstein
CoRR(2023)
摘要
We present a versatile adaptation of existing dimensionality reduction (DR) objectives, enabling the simultaneous reduction of both sample and feature sizes. Correspondances between input and embedding samples are computed through a semi-relaxed Gromov-Wasserstein optimal transport (OT) problem. When the embedding sample size matches that of the input, our model recovers classical popular DR models. When the embedding's dimensionality is unconstrained, we show that the OT plan delivers a competitive hard clustering. We emphasize the importance of intermediate stages that blend DR and clustering for summarizing real data and apply our method to visualize datasets of images.
更多查看译文
关键词
dimensionality reduction,clustering,gromov-wasserstein
AI 理解论文
溯源树
样例
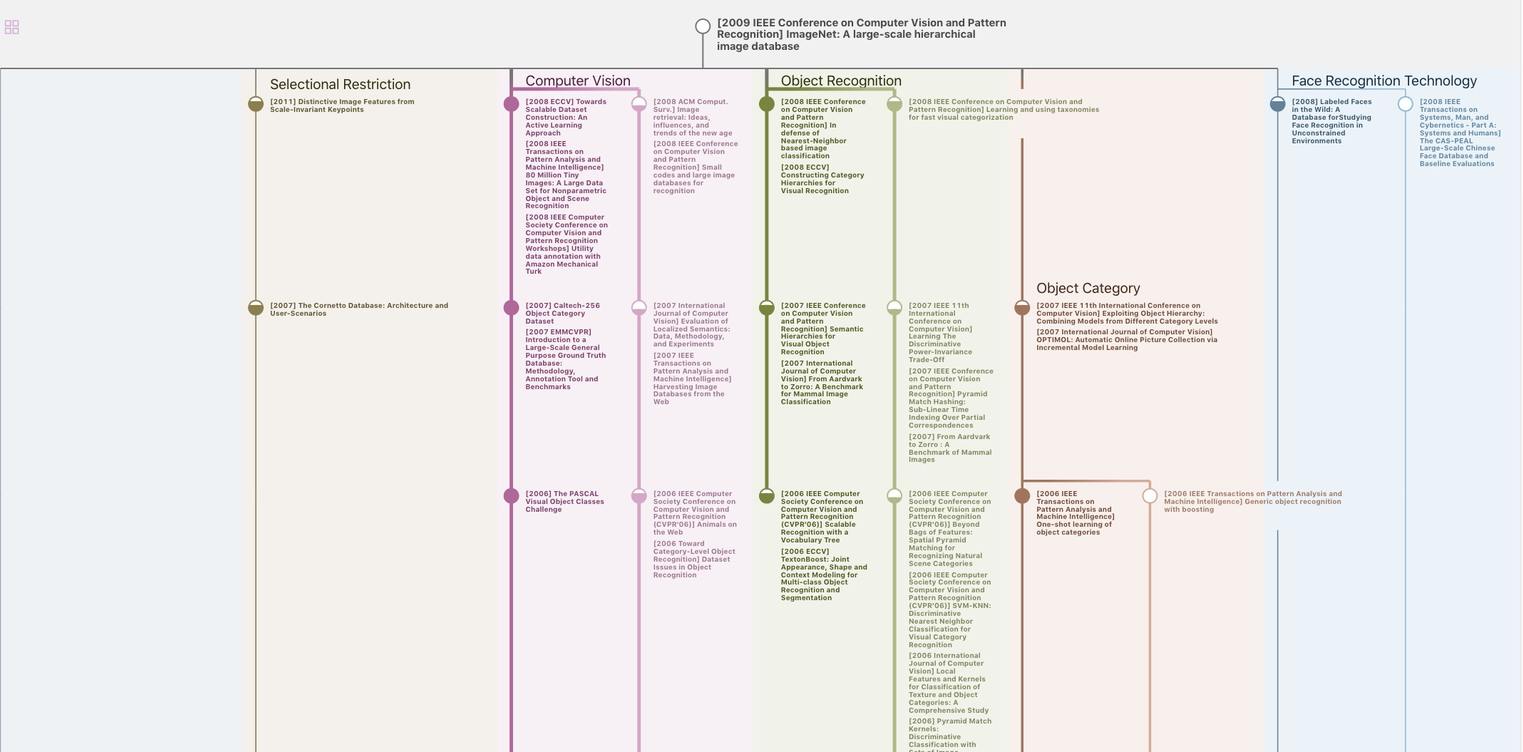
生成溯源树,研究论文发展脉络
Chat Paper
正在生成论文摘要