Causal Inference in Gene Regulatory Networks with GFlowNet: Towards Scalability in Large Systems
CoRR(2023)
Abstract
Understanding causal relationships within Gene Regulatory Networks (GRNs) is essential for unraveling the gene interactions in cellular processes. However, causal discovery in GRNs is a challenging problem for multiple reasons including the existence of cyclic feedback loops and uncertainty that yields diverse possible causal structures. Previous works in this area either ignore cyclic dynamics (assume acyclic structure) or struggle with scalability. We introduce Swift-DynGFN as a novel framework that enhances causal structure learning in GRNs while addressing scalability concerns. Specifically, Swift-DynGFN exploits gene-wise independence to boost parallelization and to lower computational cost. Experiments on real single-cell RNA velocity and synthetic GRN datasets showcase the advancement in learning causal structure in GRNs and scalability in larger systems.
MoreTranslated text
Key words
gene regulatory networks,regulatory networks,gflownet
AI Read Science
Must-Reading Tree
Example
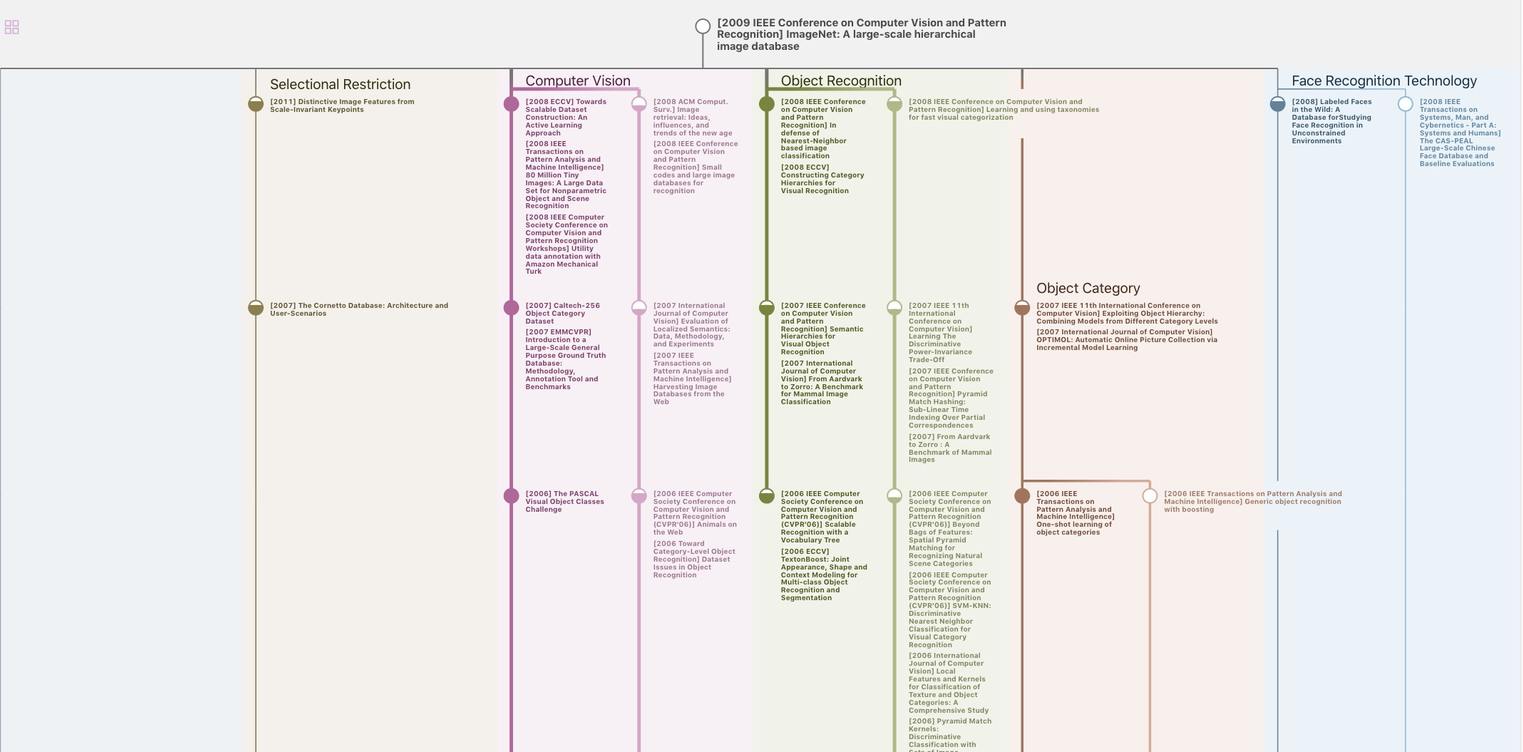
Generate MRT to find the research sequence of this paper
Chat Paper
Summary is being generated by the instructions you defined