Comparative analysis of the capability of the extended biotic ligand model and machine learning approaches to predict arsenate toxicity.
Chemosphere(2023)
摘要
Assessment of inorganic arsenate (As(V)) is critical for ensuring a sustainable environment because of its adverse effects on humans and ecosystems. This study is the first to attempt to predict As(V) toxicity to the bioluminescent bacterium Aliivibrio fischeri exposed to varying As(V) dosages and environmental factors (pH and phosphate concentration) using six machine learning (ML)-guided models. The predicted toxicity values were compared with those predicted using the extended biotic ligand model (BLM) we previously developed to evaluate the toxic effect of oxyanion (i.e., As(V)). The relationship between the variables (input features) and toxicity (output) was found to play an important role in the prediction accuracy of each ML-guided model. The results indicated that the extended BLM had the highest prediction accuracy, with a root mean square error (RMSE) of 12.997. However, with an RMSE of 14.361, the multilayer perceptron (MLP) model exhibited quasi-accurate prediction, despite having been trained with a relatively small dataset (n = 256). In view of simplicity, an MLP model is compatible with an extended BLM and does not require expert knowledge for the derivation of specific parameters, such as binding fraction and binding constant values. Furthermore, with the development and employment of reliable in-situ sensing techniques, monitoring data are expected to be augmented faster to provide sufficient training data for the improvement of prediction accuracy which may, thus, allow it to outperform the extended BLM after obtaining sufficient data.
更多查看译文
关键词
extended biotic ligand model,toxicity,machine learning
AI 理解论文
溯源树
样例
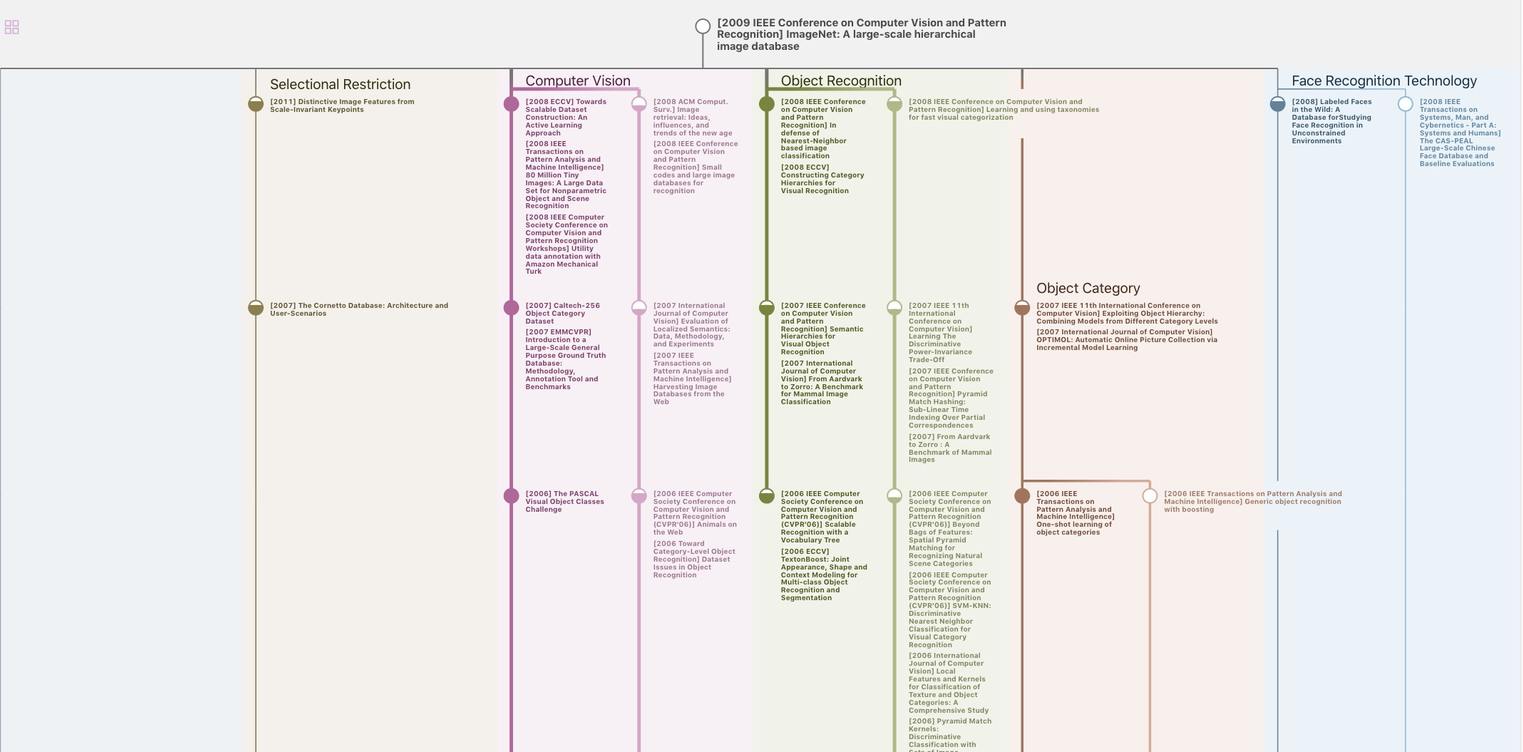
生成溯源树,研究论文发展脉络
Chat Paper
正在生成论文摘要