Myositis Detection From Muscle Ultrasound Images Using a Proposed YOLO-CSE Model
IEEE Access(2023)
摘要
Myositis is a rare muscle disorder resulting from the immune system’s attack on the muscle cells, leading to muscle weaknesses. A precise and early diagnosis of the illness is crucial for effective treatment. Differentiating among various types of Myositis remains a significant challenge, leading to false diagnoses and delayed treatment for IBM patients, which can cause muscular atrophy. This paper introduces a new Hybrid computer-aided model that can effectively differentiate among various categories: PolyMyositis (PM), DermatoMyositis (DM), and Inclusion Body Myositis (IBM). A novel method for detecting and classifying inflammatory myopathies from ultrasound images based on a modified lightweight YOLOv5 Architecture is proposed named YOLO-CSE. The proposed model utilizes the Convolutional Block Attention Module (CBAM) to focus on the significant minor extracted features in the image. Moreover, we reuse the extracted features by incorporating the Spatial Pyramid Pooling-Fast Plus (SPPF $^{+}$ ), strengthening the feature extraction capability while providing maximum information flow in the network. Furthermore, the Exponential Linear Unit (ELU) is integrated to activate the function for more accurate results by naming this layer (SPPF $^{+}$ ELU). The performance is tested on large benchmark datasets consisting of 3214 muscle ultrasound images for 80 patients, divided into 14 DermatoMyositis (DM), 14 PolyMyositis (PM), 19 Inclusion Body Myositis (IBM) and 33 Normal patients. Extensive and detailed analysis has been tested on several stages to clarify the role of the CBAM and SPPF Plus modules in our model. Additionally, we analyze the model’s performance through four different suggested classification strategies (binary and multiclass classification). Data Augmentation was proposed to suppress the dataset’s imbalance and boost the model’s performance. Mosaic data augmentation enhanced the dataset by randomly cropping, arranging and scaling images. Various techniques of augmentation were employed, including flipping, cropping, rotating, Contrast Limited Adaptive Histogram Equalization (CLAHE) and Median Blur filters. Experiments have been conducted to demonstrate the effectiveness of the proposed system. The proposed YOLO-CSE achieved highly acceptable accuracy, precision, recall, and F1-Scores for the different strategies. The suggested model achieved an average accuracy for binary and multiclass classification above 98% and average precision above 96%. The results demonstrate that the proposed model accurately detects and classifies inflammatory myopathies.
更多查看译文
关键词
Classification,deep learning,idiopathic inflammatory myopathy,muscle ultrasound,myositis,myopathies
AI 理解论文
溯源树
样例
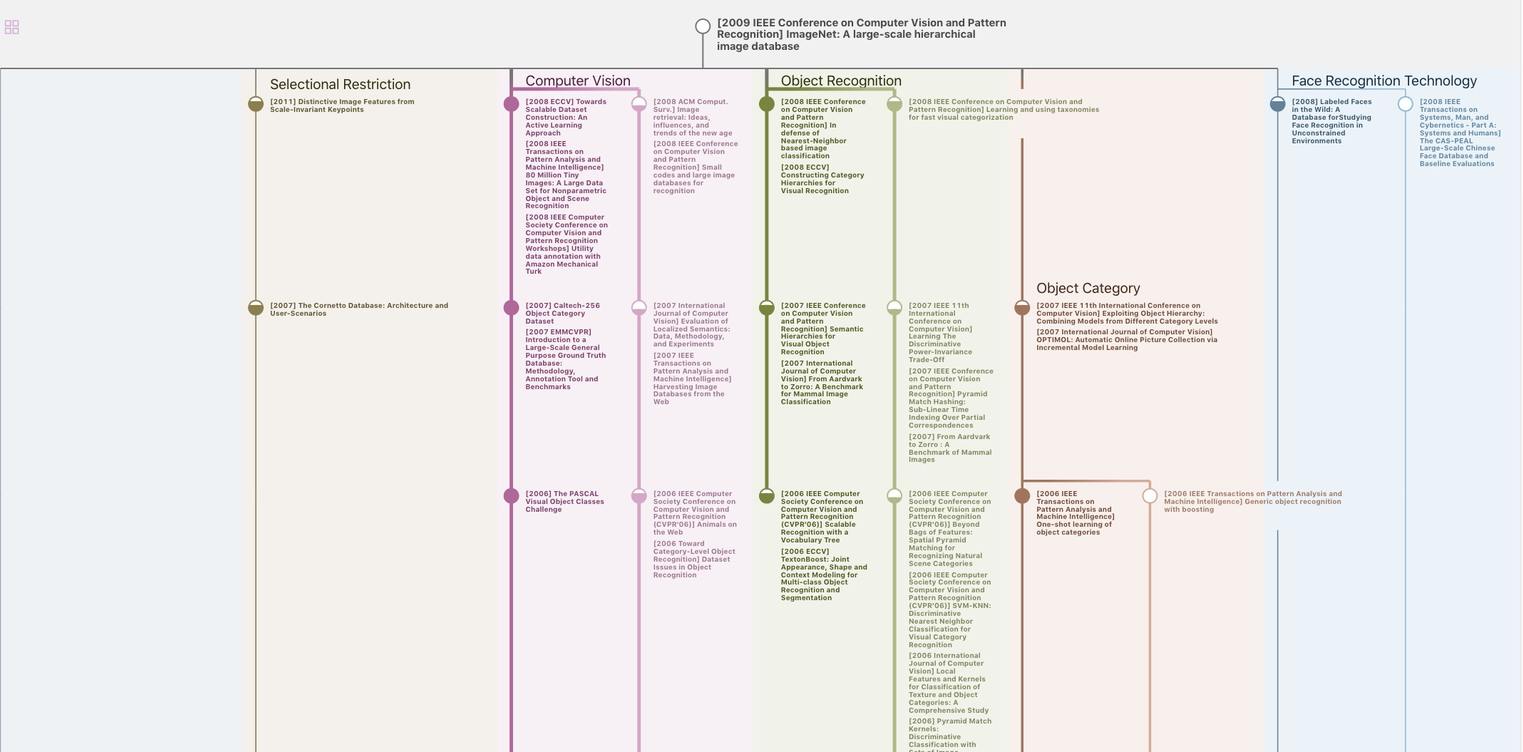
生成溯源树,研究论文发展脉络
Chat Paper
正在生成论文摘要