Classification of Fermi BCUs Using Machine Learning
The Astrophysical Journal(2023)
摘要
The Fermi Large Area Telescope (LAT) has detected 6659 γ -ray sources in the incremental version (4FGL-DR3, for Data Release 3) of the fourth Fermi-LAT catalog of γ -ray sources and 3743 of them are blazars, including 1517 blazar candidates of uncertain type (BCUs). Blazars are generally classified by properties of emission lines into BL Lac objects and flat spectrum radio quasars (FSRQs). However, BCUs are difficult to classify because of the lack of spectrum. In this work we apply five different machine-learning algorithms (K-nearest neighbors, logistic regression, support vector machine, random forest, CatBoost) to evaluate the classification of 1517 BCUs based on the observational data of 4FGL-DR3. The results indicate that the use of recursive feature elimination cross-validation can effectively improve the accuracy of models and reduce computation time. We use our models to predict the BCUs from 4FGL-DR3 and the results of the overlapping of the five models are as follows: 811 BL Lac objects, 397 FSRQs, and 309 BCUs.
更多查看译文
关键词
Blazars,BL Lacertae objects,Flat-spectrum radio quasars
AI 理解论文
溯源树
样例
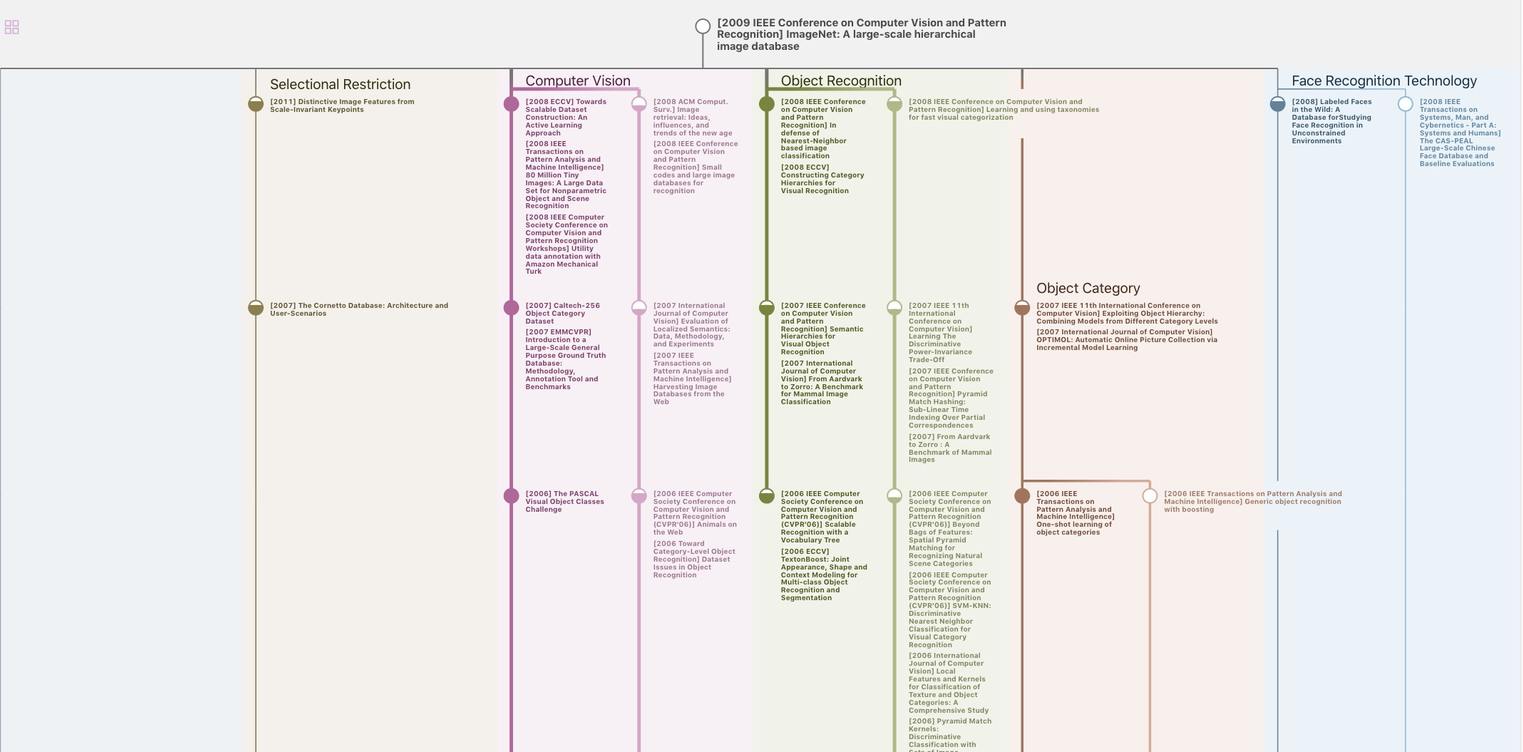
生成溯源树,研究论文发展脉络
Chat Paper
正在生成论文摘要