Identifying biomarkers for tDCS treatment response in Alzheimer’s disease patients: a machine learning approach using resting-state EEG classification
Frontiers in Human Neuroscience(2023)
摘要
BackgroundTranscranial direct current stimulation (tDCS) is a promising treatment for Alzheimer’s Disease (AD). However, identifying objective biomarkers that can predict brain stimulation efficacy, remains a challenge. The primary aim of this investigation is to delineate the cerebral regions implicated in AD, taking into account the existing lacuna in comprehension of these regions. In pursuit of this objective, we have employed a supervised machine learning algorithm to prognosticate the neurophysiological outcomes resultant from the confluence of tDCS therapy plus cognitive intervention within both the cohort of responders and non-responders to antecedent tDCS treatment, stratified on the basis of antecedent cognitive outcomes.MethodsThe data were obtained through an interventional trial. The study recorded high-resolution electroencephalography (EEG) in 70 AD patients and analyzed spectral power density during a 6 min resting period with eyes open focusing on a fixed point. The cognitive response was assessed using the AD Assessment Scale–Cognitive Subscale. The training process was carried out through a Random Forest classifier, and the dataset was partitioned into K equally-partitioned subsamples. The model was iterated k times using K−1 subsamples as the training bench and the remaining subsample as validation data for testing the model.ResultsA clinical discriminating EEG biomarkers (features) was found. The ML model identified four brain regions that best predict the response to tDCS associated with cognitive intervention in AD patients. These regions included the channels: FC1, F8, CP5, Oz, and F7.ConclusionThese findings suggest that resting-state EEG features can provide valuable information on the likelihood of cognitive response to tDCS plus cognitive intervention in AD patients. The identified brain regions may serve as potential biomarkers for predicting treatment response and maybe guide a patient-centered strategy.Clinical Trial Registrationhttps://classic.clinicaltrials.gov/ct2/show/NCT02772185?term=NCT02772185&draw=2&rank=1, identifier ID: NCT02772185.
更多查看译文
关键词
tdcs treatment response,alzheimers disease,biomarkers,resting-state
AI 理解论文
溯源树
样例
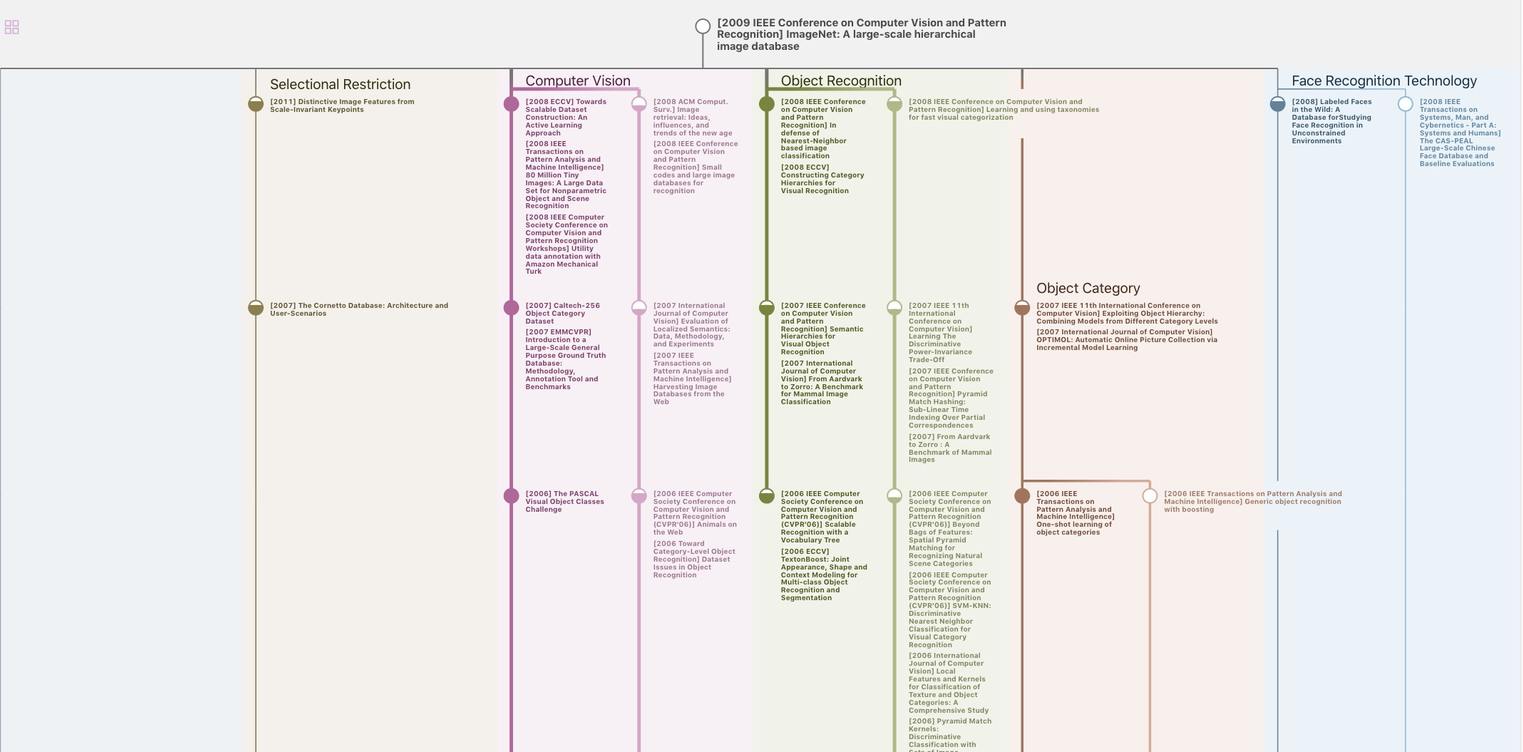
生成溯源树,研究论文发展脉络
Chat Paper
正在生成论文摘要