Structure-Based Inverse Reinforcement Learning for Quantification of Biological Knowledge
2023 IEEE CONFERENCE ON ARTIFICIAL INTELLIGENCE, CAI(2023)
摘要
Gene regulatory networks (GRNs) play crucial roles in various cellular processes, including stress response, DNA repair, and the mechanisms involved in complex diseases such as cancer. Biologists are involved in most biological analyses. Thus, quantifying their policies reflected in available biological data can significantly help us to better understand these complex systems. The primary challenges preventing the utilization of existing machine learning, particularly inverse reinforcement learning techniques, to quantify biologists' knowledge are the limitations and huge amount of uncertainty in biological data. This paper leverages the networklike structure of GRNs to define expert reward functions that contain exponentially fewer parameters than regular reward models. Numerical experiments using mammalian cell cycle and synthetic gene-expression data demonstrate the superior performance of the proposed method in quantifying biologists' policies.
更多查看译文
关键词
Inverse Reinforcement Learning,Machine Learning,Gene Regulatory Networks
AI 理解论文
溯源树
样例
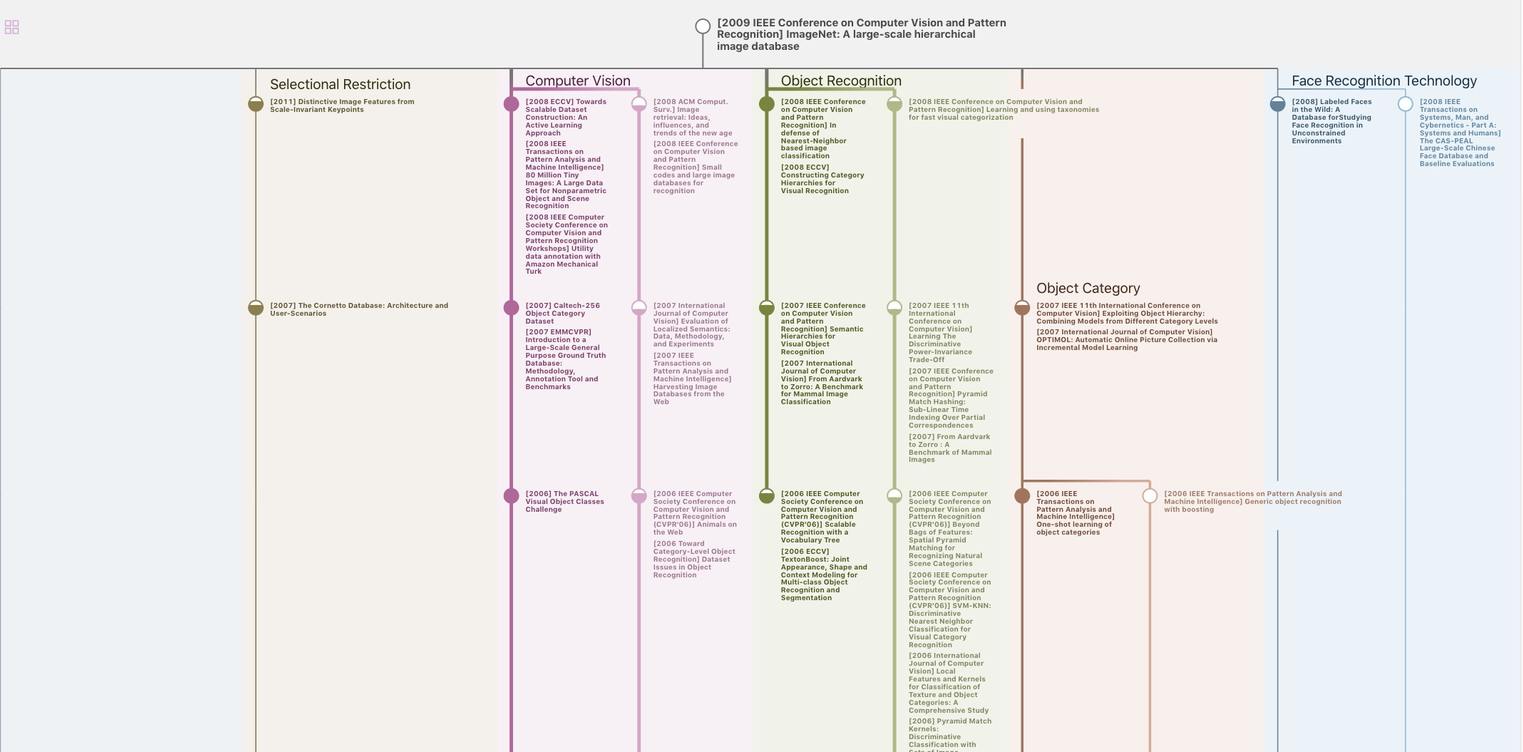
生成溯源树,研究论文发展脉络
Chat Paper
正在生成论文摘要