SACNet: A Multiscale Diffeomorphic Convolutional Registration Network with Prior Neuroanatomical Constraints for Flexible Susceptibility Artifact Correction in Echo Planar Imaging
bioRxiv (Cold Spring Harbor Laboratory)(2023)
摘要
Susceptibility artifacts (SAs), which are inevitable for modern diffusion brain MR images with single-shot echo planar imaging (EPI) protocols in wide large-scale neuroimaging datasets, severely hamper the accurate detection of the human brain white matter structure. While several conventional and deep-learning based distortion correction methods have been proposed, the correction quality and model generality of these approaches are still limited. Here, we proposed the SACNet, a flexible SAs correction (SAC) framework for brain diffusion MR images of various phase-encoding EPI protocols based on an unsupervised learning-based registration convolutional neural network. This method could generate smooth diffeomorphic warps with optional neuroanatomy guidance to correct both geometric and intensity distortions of SAs. By employing near 2000 brain scans covering neonatal, child, adult and traveling participants, our SACNet consistently demonstrates state-of-the-art correction performance and effectively eliminates SAs-related multicenter effects compared with existing SAC methods. To facilitate the development of standard SAC tools for future neuroimaging studies, we also created easy-to-use command lines incorporating containerization techniques for quick user deployment.
### Competing Interest Statement
The authors have declared no competing interest.
更多查看译文
关键词
prior neuroanatomical constraints,flexible susceptibility artifact correction,imaging
AI 理解论文
溯源树
样例
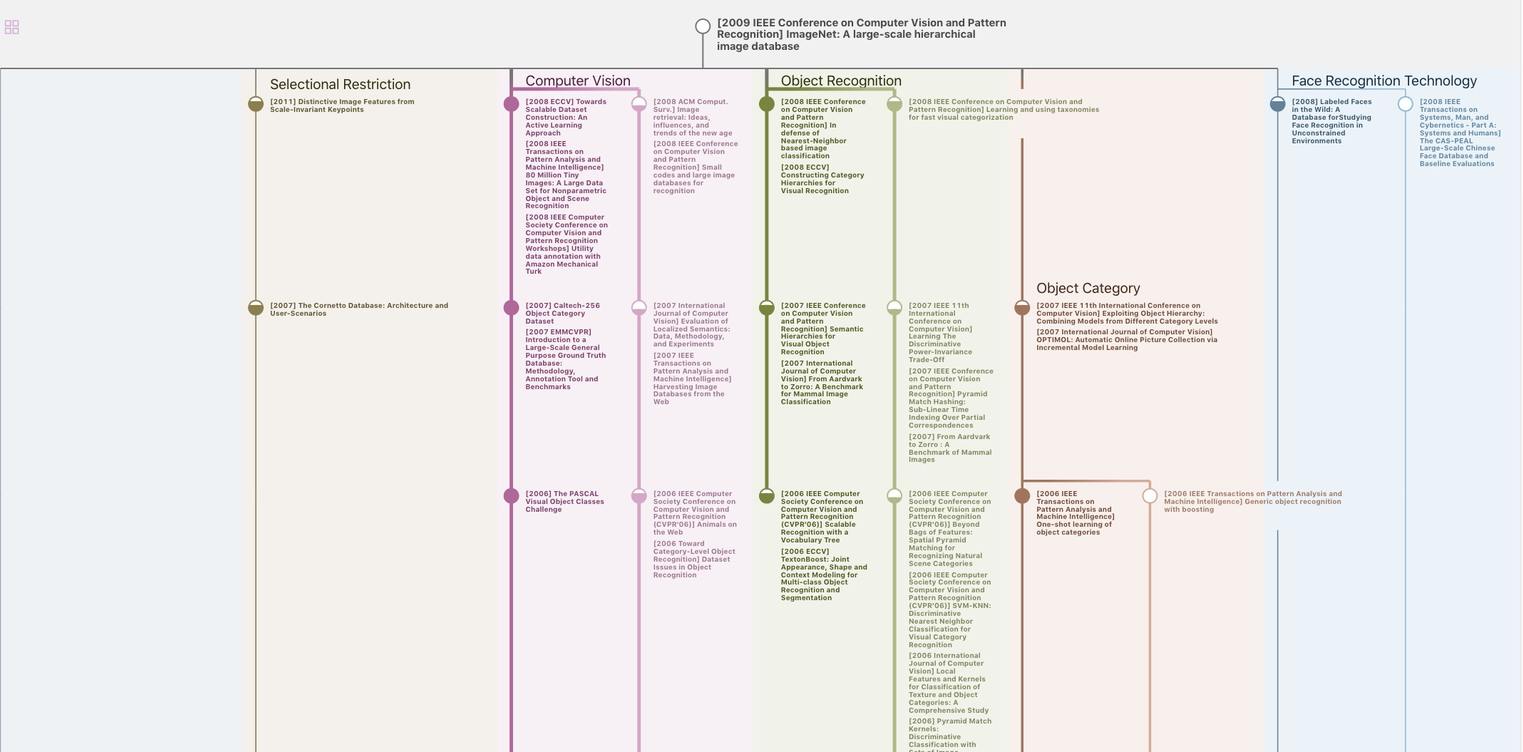
生成溯源树,研究论文发展脉络
Chat Paper
正在生成论文摘要