Effects of Data Transformation and Model Selection on Feature Importance in Microbiome Classification Data
biorxiv(2024)
摘要
Accurate classification of host phenotypes from microbiome data is essential for future therapies in microbiome-based medicine and machine learning approaches have proved to be an effective solution for the task. The complex nature of the gut microbiome, data sparsity, compositionality and population-specificity however remain challenging, which highlights the critical need for standardized methodologies to improve the accuracy and reproducibility of the results. Microbiome data transformations can alleviate some of the aforementioned challenges, but their usage in machine learning tasks has largely been unexplored. Our aim was to assess the impact of various data transformations on the accuracy, generalizability and feature selection by analysis using more than 8,500 samples from 24 shotgun metagenomic datasets. Our findings demonstrate the feasibility of distinguishing between healthy and diseased individuals using microbiome data with minimal dependence on the algorithm and transformation selection. Remarkably, presence-absence transformation performed comparably well to abundance-based transformations, and only a small subset of predictors is crucial for accurate classification. However, while different transformations resulted in comparable classification performance, the most important features varied significantly, which highlight the need to reevaluate machine-learning based biomarker detection. Our research provides valuable guidance for applying machine learning on microbiome data, offering novel insights and highlighting important areas for future research.
### Competing Interest Statement
The authors have declared no competing interest.
更多查看译文
AI 理解论文
溯源树
样例
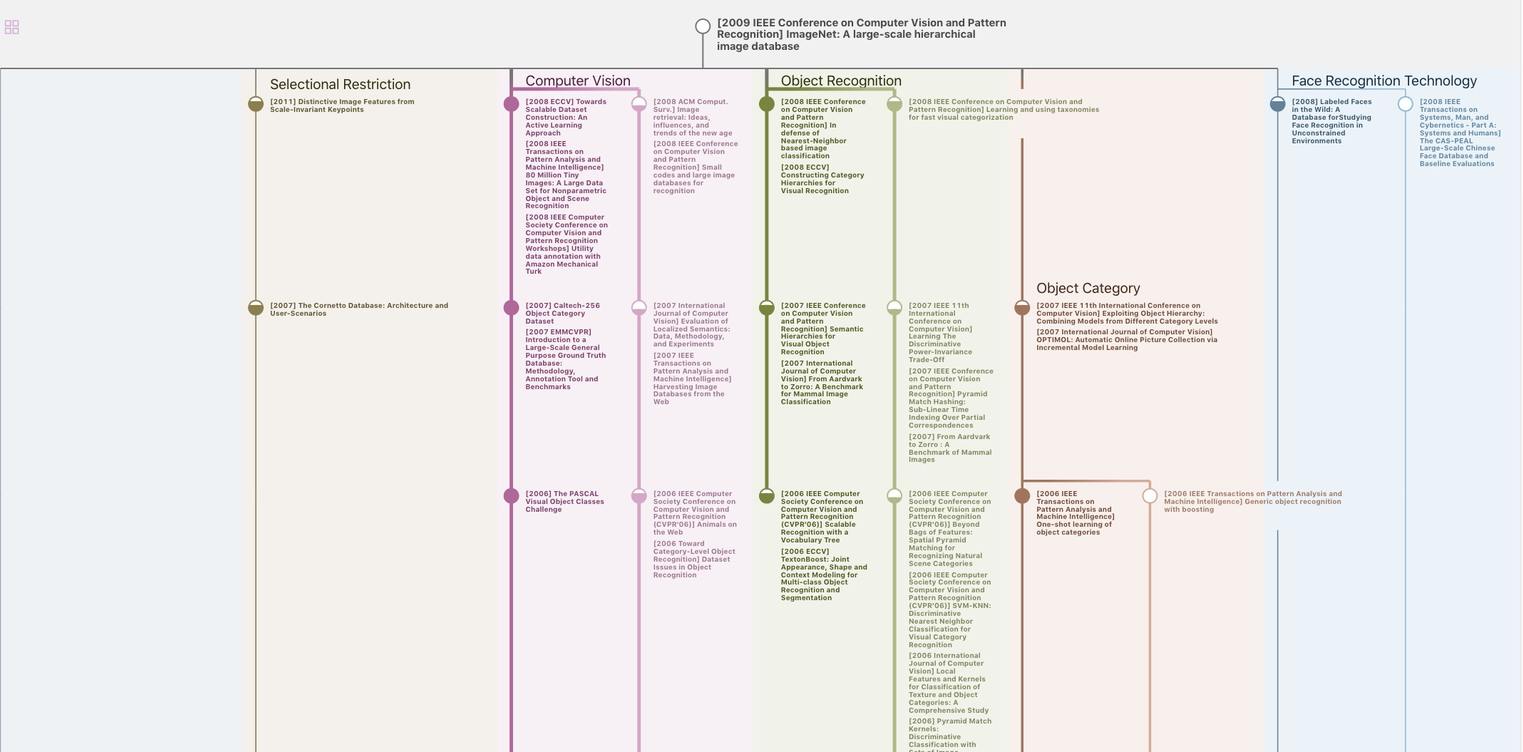
生成溯源树,研究论文发展脉络
Chat Paper
正在生成论文摘要