Deep Variational Autoencoders for Population Genetics
bioRxiv (Cold Spring Harbor Laboratory)(2023)
摘要
Motivation Modern biobanks provide numerous high-resolution genomic sequences of diverse populations. These datasets enable a better understanding of genotype-phenotype interactions with genome-wide association studies (GWAS) and power a new personalized precision medicine with polygenic risk scores (PRS). In order to account for diverse and admixed populations, new algorithmic tools are needed in order to properly capture the genetic composition of populations. Here we explore deep learning techniques, namely variational autoencoders (VAEs), to process genomic data from a population perspective. We hope this work will encourage the adoption of deep neural networks in the population genetics community.
Results In this paper, we show the power of VAEs for a variety of tasks relating to the interpretation, classification, simulation, and compression of genomic data with several worldwide whole genome datasets from both humans and canids and evaluate the performance of the proposed applications with and without ancestry conditioning. The unsupervised setting of autoencoders allows for the detection and learning of granular population structure and inferring of informative latent factors. The learned latent spaces of VAEs are able to capture and represent differentiated Gaussian-like clusters of samples with similar genetic composition on a fine-scale from single nucleotide polymorphisms (SNPs), enabling applications in dimensionality reduction, data simulation, and imputation. These individual genotype sequences can then be decomposed into latent representations and reconstruction errors (residuals) which provide a sparse representation useful for lossless compression. We show that different population groups have differentiated compression ratios and classification accuracies. Additionally, we analyze the entropy of the SNP data, its effect on compression across populations, its relation to historical migrations, and we show how to introduce autoencoders into existing compression pipelines.
### Competing Interest Statement
A.G.I. holds shares in Galatea Bio. The remaining authors declare no competing interests.
* ANN
: artificial neural network;
VAE
: variational autoencoder;
GWAS
: genome-wide association study;
SNP
: single nucleotide polymorphism;
MLP
: multilayer perceptron;
LAI
: local ancestry inference;
PPM
: prediction by partial matching;
GRU
: gated recurrent unit;
LSTM
: long short-term memory;
LD
: linkage disequilibrium;
GAN
: generative adversarial network;
RBM
: restricted boltzmann machine;
ReLU
: rectified linear unit;
GELU
: gaussian error linear unit;
MAF
: minor allele frequency;
BCE
: binary cross-entropy;
KL
: Kullback-Leibler;
MAP
: maximum a posteriori;
PCA
: principal component analysis;
DBI
: Davies-Bouldin index;
SC
: silhouette coefficient;
AFR
: African;
EUR
: European;
AMR
: Native American;
WAS
: West Asian;
SAS
: South Asian;
OCE
: Oceanian;
RLE
: run-length encoding;
MCAR
: missing completely at random;
MNAR
: missing not at random;
VQ-VAE
: vector quantized variational autoencoder;
更多查看译文
关键词
genetics,population,deep
AI 理解论文
溯源树
样例
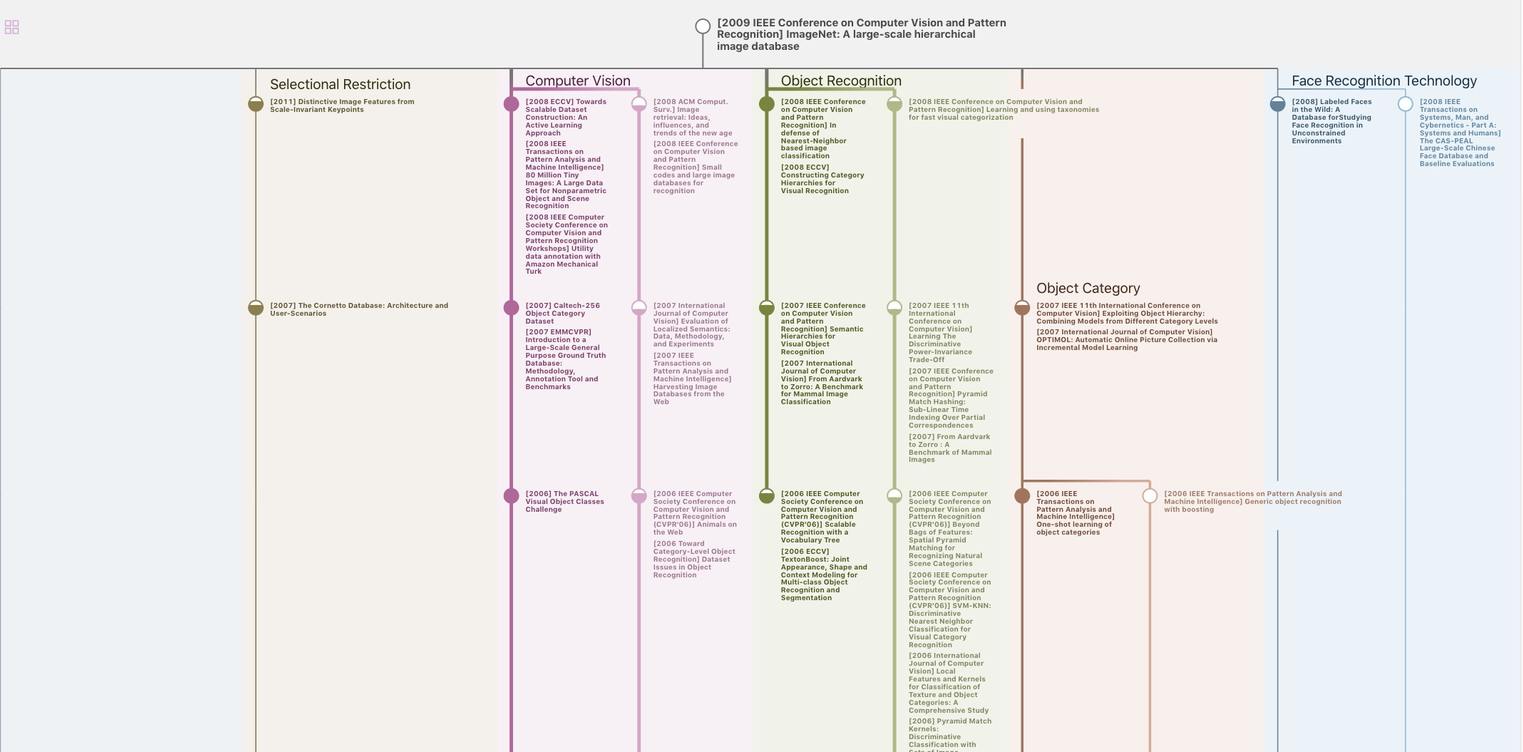
生成溯源树,研究论文发展脉络
Chat Paper
正在生成论文摘要