Efficiently Counting Triangles for Hypergraph Streams by Reservoir-Based Sampling
IEEE Transactions on Knowledge and Data Engineering(2023)
摘要
Hypergraph streams provide an efficient model to express and preserve complex connections in various applications. Triangles in a hypergraph can be formed by vertices and hyperedges. The specific counts of triangles are important to analyze various applications. Due to the huge costs of counting triangles based on the whole datasets, a sampling-and-estimating framework has low overhead while obtaining a relatively accurate result. However, existing sampling algorithms focus on pairwise graph streams, they estimate the counts of triangles formed by vertices with large estimation errors and can not be applied to count triangles formed by hyperedges. Therefore, this paper first proposes a sampling-and-estimating framework that produces hyperedge samples using a reservoir with static capacity to estimate the total counts of triangles by inferring the probabilities of forming the triangles respectively. Furthermore, to improve the estimation accuracy, this paper proposes another sampling-and-estimating framework to produce samples in the form of hyperedge pairs which can be used to compute the probabilities of the formations of triangles more accurately and then estimate the total triangle counts with smaller estimation variances. The extensive experiments based on real-world datasets confirm the efficiency and accuracy of our proposed frameworks for counting triangles in different types of hypergraphs at a small sampling ratio.
更多查看译文
关键词
Mercury (metals), Estimation error, Switches, Costs, Real-time systems, Image edge detection, Viruses (medical), Hypergraph streams, triangles counting, reservoir-based sampling, social networks
AI 理解论文
溯源树
样例
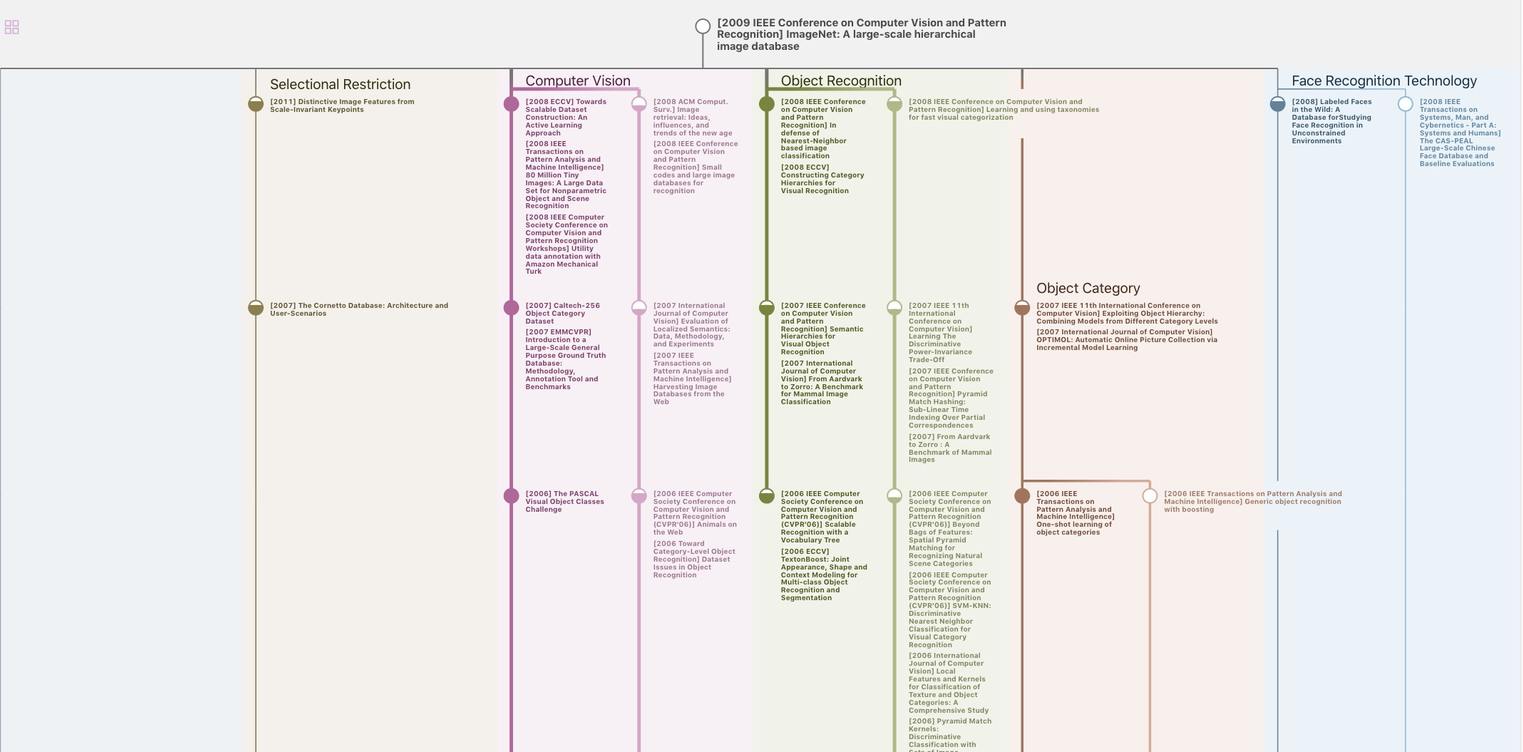
生成溯源树,研究论文发展脉络
Chat Paper
正在生成论文摘要