Learning-Aided Life Cycle Prediction of Batteries in Data Centers
2023 International Conference on Smart Electrical Grid and Renewable Energy (SEGRE)(2023)
摘要
Data centers (DCs) have become the foundation of cloud computing technology by providing powerful computing capabilities. To ensure supplemental power supply timely in case of a power outage, batteries are widely used in the power supply system in the DCs. Thus, The precise remaining useful life prediction of the batteries can enable the DCs to operate safely and energy-efficiently. This paper proposes a life cycle prediction scheme for batteries, which designs an AI-aided union-long-short term memory (un-LSTM) algorithm for the stationary stage and the sudden drop stage of battery performance. To reduce the prediction error, pre-processing is designed to provide an accurate start time for battery life prediction. Furthermore, the multidimensional failure analysis increases the dimensionality of the features of the life prediction samples. Through the introduction of fault analysis, the life prediction part can well predict the curve types with large difference in characteristics. The simulation results demonstrate that compared with the base LSTM operation and maintenance (O&M) and expert experience O&M, un-LSTM saves 6.8% and 64.2% of the O&M costs. In addition, the prediction accuracy of the life curve has also increased by 15.9%, which also presents a well performance of the proposed scheme in preventing security risks of data centers.
更多查看译文
关键词
energy-saving,AI,battery life prediction
AI 理解论文
溯源树
样例
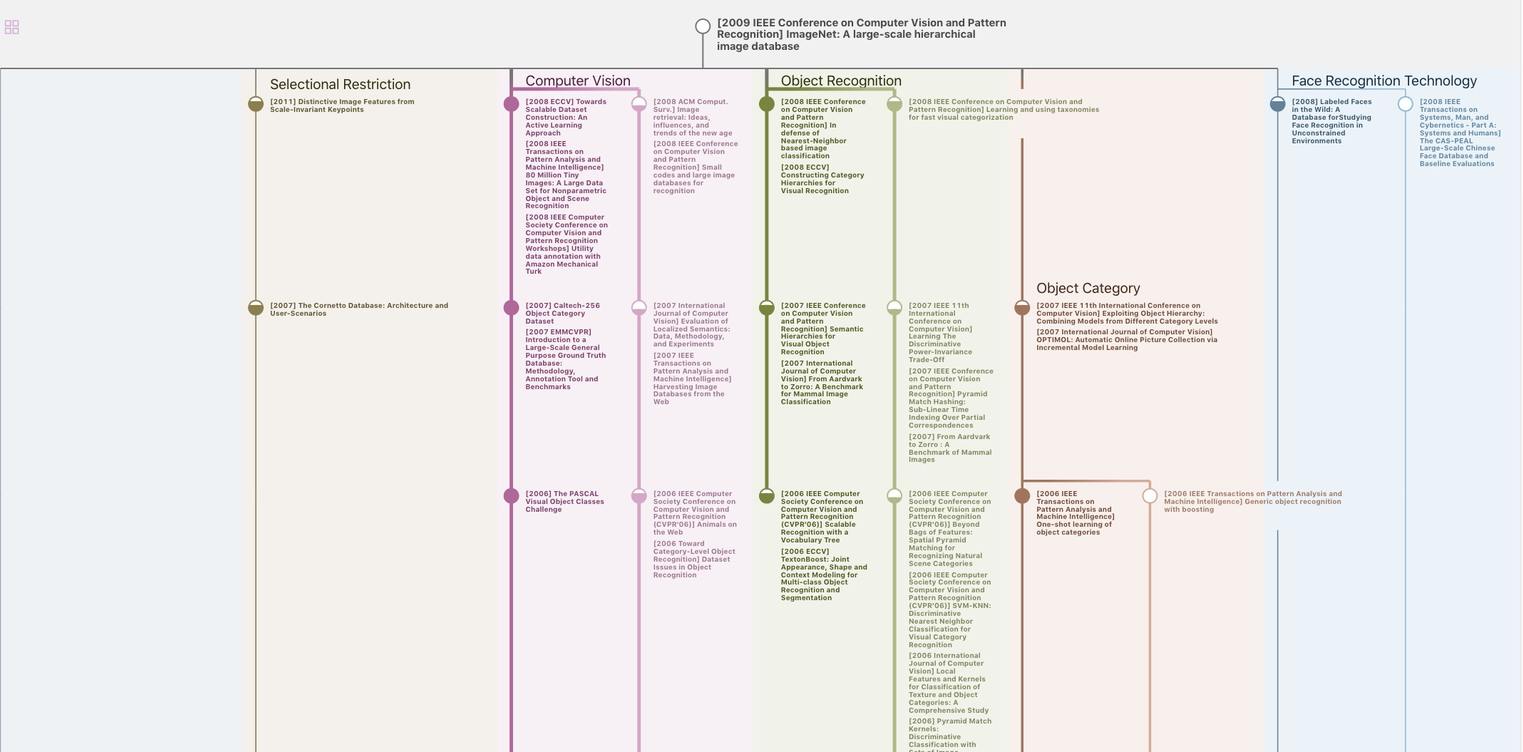
生成溯源树,研究论文发展脉络
Chat Paper
正在生成论文摘要