Multi-Features Fusion based Viewport Prediction with GNN for 360-Degree Video Streaming.
MetaCom(2023)
摘要
360-degree video streaming is one of the essential VR applications which provides viewers with immersive experiences. However, traditional viewport prediction methods for tile-based 360-degree video streaming that aim to maximize prediction accuracy cannot guarantee the Quality of Experience (QoE) for viewers because they do not fully exploit the correlation of video content and users’ viewing behavior. In this paper, we propose a multi-features fusion based viewport prediction method for 360-degree video streaming using graph neural network (GNN) to improve both prediction accuracy and users’ QoE. First, we extract three tile-level prediction features from the current user’s viewing information, video content, and cross-user information. Then, we create a sparse directed graph to represent the relationship between video content and viewing behavior by using the three tile-level features to characterize nodes and the viewed traces to describe edges. Following that, the GNN is used to receive the graph as input and output prediction viewing probability for each tile. The multi-features fusion based prediction model is obtained after data training. In addition, a tile-oriented quality adaptation is proposed to help with 360-degree video streaming. Extensive experiments using public datasets show that the proposed approach outperforms state-of-the-art solutions in terms of prediction accuracy and users’ QoE.
更多查看译文
AI 理解论文
溯源树
样例
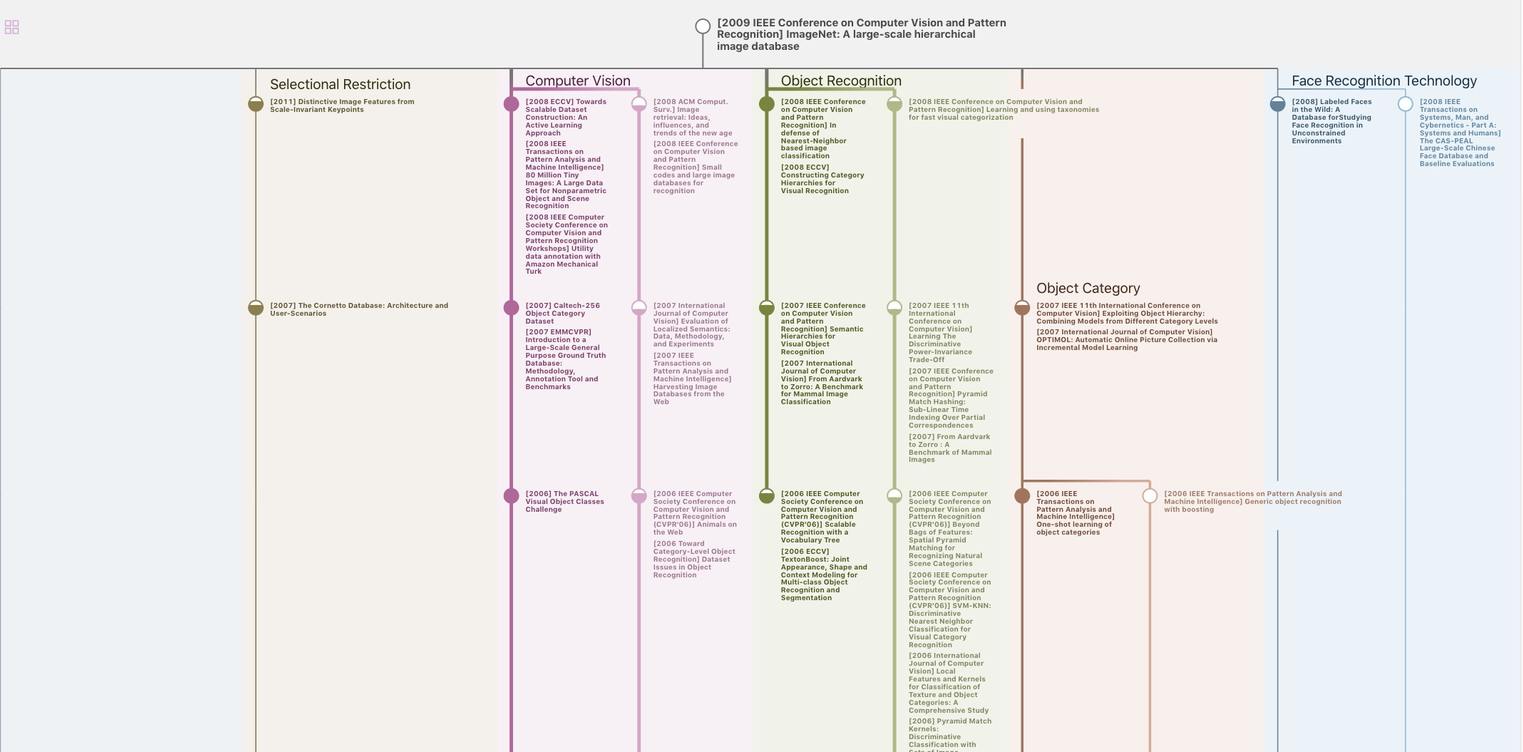
生成溯源树,研究论文发展脉络
Chat Paper
正在生成论文摘要