A 4.27TFLOPS/W FP4/FP8 Hybrid-Precision Neural Network Training Processor Using Shift-Add MAC and Reconfigurable PE Array
ESSCIRC 2023- IEEE 49th European Solid State Circuits Conference (ESSCIRC)(2023)
摘要
This paper presents an energy-efficient FP4/FP8 hybrid-precision training processor. Through hardware-software co-optimization, the design efficiently implements all general matrix multiply (GEMM) operations required for training using only shift-add multiply-accumulate (MAC) units. The reconfigurable processing element (PE) array further improves efficiency by significantly reducing on-chip memory access. The on-chip convolution decomposition technique supports a wide range of kernels using simple homogeneous data routing. Fabricated in 40nm CMOS, the processor achieves 2.61TFLOPS/W real-model efficiency for ResNet-18 training, outperforming prior art by 59%.
更多查看译文
关键词
Deep Learning, Low-precision Training, Logarithmic Weight, Reconfigurable PE Array
AI 理解论文
溯源树
样例
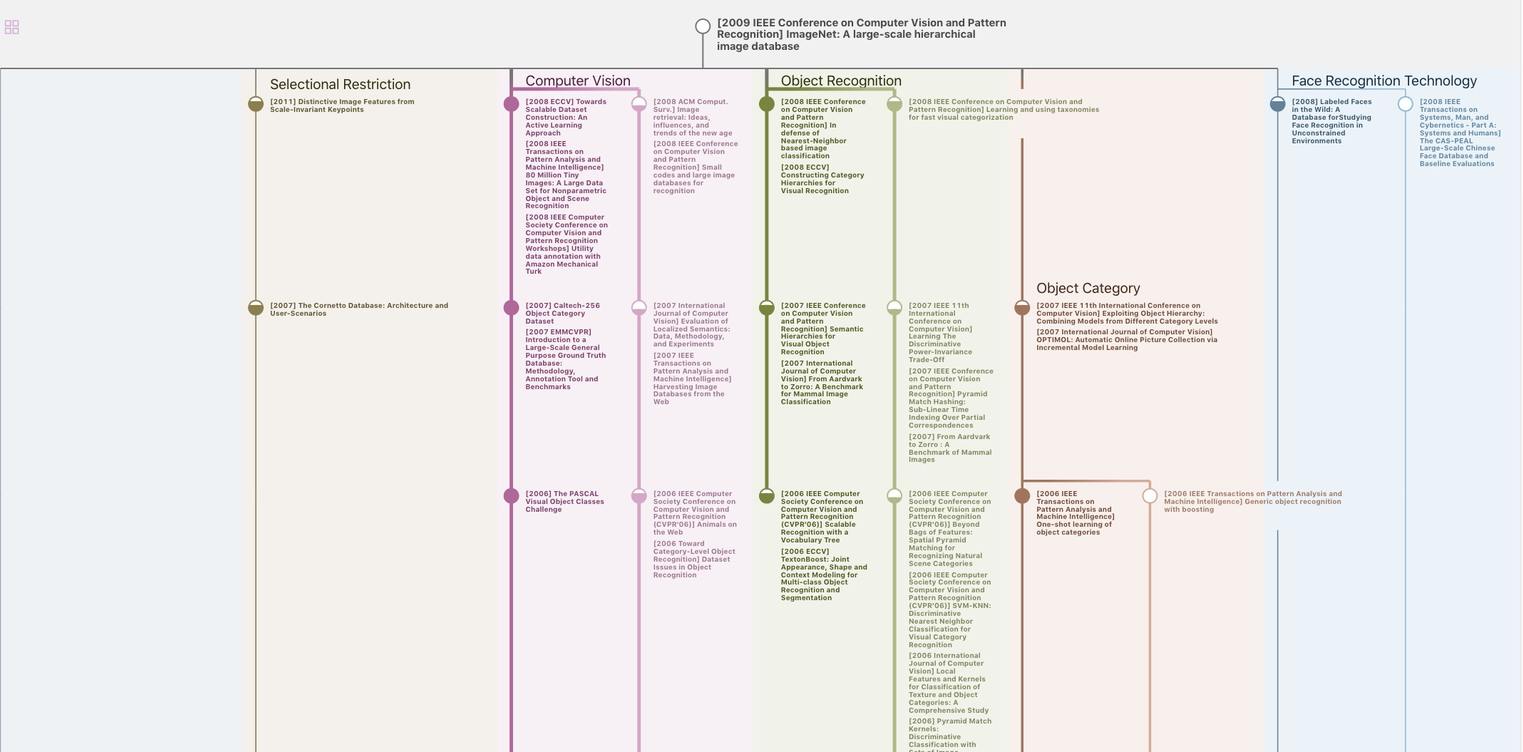
生成溯源树,研究论文发展脉络
Chat Paper
正在生成论文摘要