Cross-domain mechanism for few-shot object detection on Urine Sediment Image.
Computers in biology and medicine(2023)
摘要
Deep learning object detection networks require a large amount of box annotation data for training, which is difficult to obtain in the medical image field. The few-shot object detection algorithm is significant for an unseen category, which can be identified and localized with a few labeled data. For medical image datasets, the image style and target features are incredibly different from the knowledge obtained from training on the original dataset. We propose a background suppression attention(BSA) and feature space fine-tuning module (FSF) for this cross-domain situation where there is a large gap between the source and target domains. The background suppression attention reduces the influence of background information in the training process. The feature space fine-tuning module adjusts the feature distribution of the interest features, which helps to make better predictions. Our approach improves detection performance by using only the information extracted from the model without maintaining additional information, which is convenient and can be easily plugged into other networks. We evaluate the detection performance in the in-domain situation and cross-domain situation. In-domain experiments on the VOC and COCO datasets and the cross-domain experiments on the VOC to medical image dataset UriSed2K show that our proposed method effectively improves the few-shot detection performance.
更多查看译文
AI 理解论文
溯源树
样例
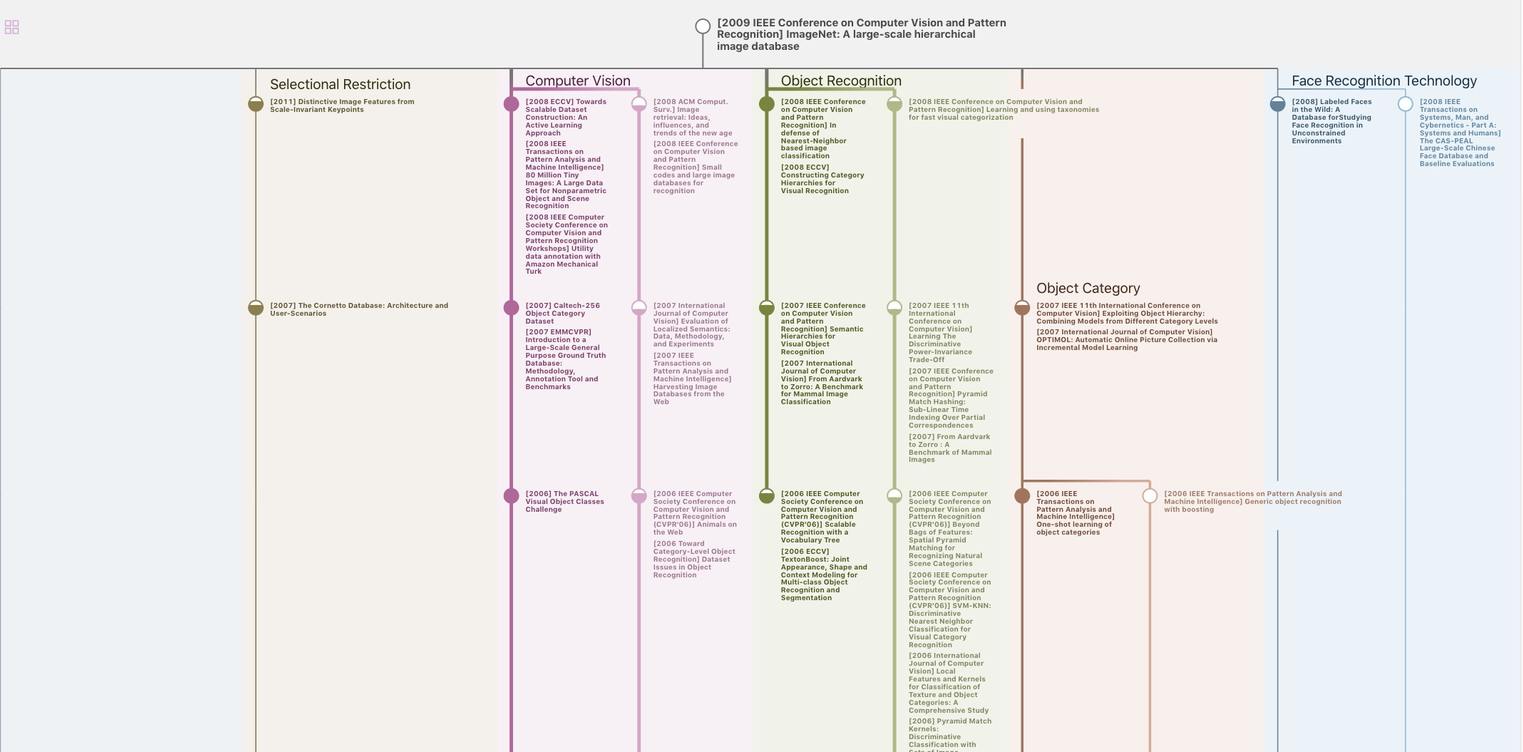
生成溯源树,研究论文发展脉络
Chat Paper
正在生成论文摘要