Feature multi-level attention spatio-temporal graph residual network: A novel approach to ammonia nitrogen concentration prediction in water bodies by integrating external influences and spatio-temporal correlations
SCIENCE OF THE TOTAL ENVIRONMENT(2024)
摘要
Accurate prediction of ammonia nitrogen concentration in water is of great significance for urban water quality management and pollution early warning. In order to improve the prediction accuracy of ammonia nitrogen concentration in water, this study developed a novel model based on graph neural networks called Feature Multilevel Attention Spatio-Temporal Graph Residual Network (FMA-STGRN). The FMA-STGRN model utilizes external influencing factors such as meteorological factors and point of interest data, as well as the spatiotemporal correlation information of ammonia nitrogen concentration between water quality monitoring stations, to accurately predict the concentration of ammonia nitrogen in water. The model consists of four main components: feature multi-level attention module, spatial graph convolution module, temporal-domain residual decomposition module, and feature fusion and output module. Through the organic combination of these four modules, FMA-STGRN can more effectively explore the complex spatio-temporal correlation relationships between water quality monitoring stations and more accurately integrate and utilize external influencing factors, thereby improving the prediction accuracy of ammonia nitrogen concentration in water. Experimental results show that the FMA-STGRN model outperforms other benchmark models such as RF, MART, MLP, LSTM, GRU, ST-GCN, and ST-GAT in various aspects. In addition, a series of feature ablation experiments were conducted to further reveal the key contributions of meteorological factors and point of interest data to the model perfor-mance. Overall, our research provides a powerful and practical tool for water quality monitoring and urban water management, with broad application prospects.
更多查看译文
关键词
Ammonia -nitrogen concentration prediction,Graph neural networks,Spatio-temporal data modeling,Urban water management
AI 理解论文
溯源树
样例
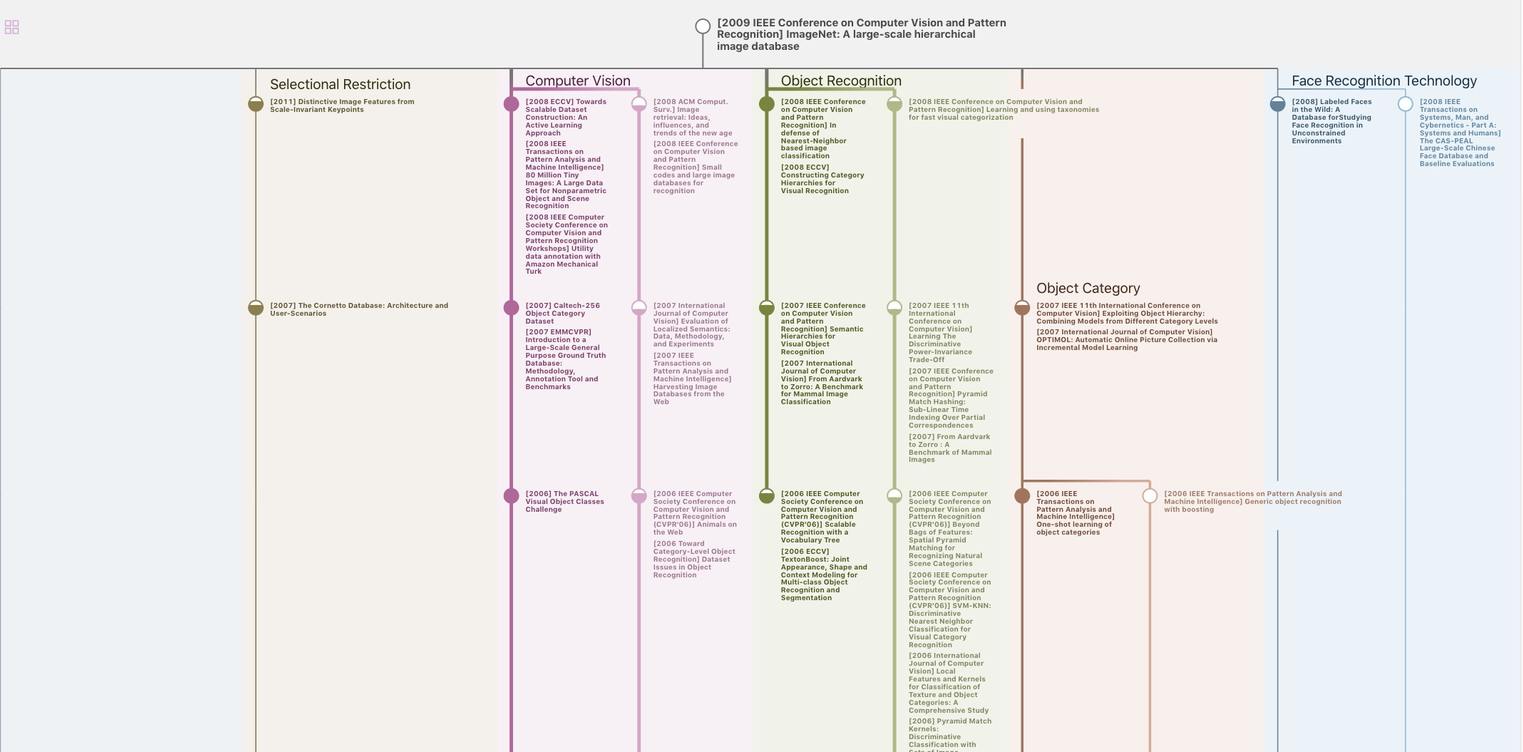
生成溯源树,研究论文发展脉络
Chat Paper
正在生成论文摘要