Global and Regional Deep Learning Models for Multiple Sclerosis Stratification from MRI.
Journal of Magnetic Resonance Imaging(2023)
摘要
Background: The combination of anatomical MRI and deep learning-based methods such as convolutional neural networks (CNNs) is a promising strategy to build predictive models of multiple sclerosis (MS) prognosis. However, studies assessing the effect of different input strategies on model's performance are lacking. Purpose: To compare whole-brain input sampling strategies and regional/specific-tissue strategies, which focus on a priori known relevant areas for disability accrual, to stratify MS patients based on their disability level. Study Type: Retrospective. Subjects: Three hundred nineteen MS patients (382 brain MRI scans) with clinical assessment of disability level performed within the following 6 months (similar to 70% training/similar to 15% validation/similar to 15% inference in-house dataset) and 440 MS patients from multiple centers (independent external validation cohort). Field Strength/Sequence: Single vendor 1.5 T or 3.0 T. Magnetization-Prepared Rapid Gradient-Echo and Fluid-Attenuated Inversion Recovery sequences. Assessment: A 7-fold patient cross validation strategy was used to train a 3D-CNN to classify patients into two groups, Expanded Disability Status Scale score (EDSS) >= 3.0 or EDSS < 3.0. Two strategies were investigated: 1) a global approach, taking the whole brain volume as input and 2) regional approaches using five different regions-of-interest: white matter, gray matter, subcortical gray matter, ventricles, and brainstem structures. The performance of the models was assessed in the in-house and the independent external cohorts. Statistical Tests: Balanced accuracy, sensitivity, specificity, area under receiver operating characteristic (ROC) curve (AUC). Results: With the in-house dataset, the gray matter regional model showed the highest stratification accuracy (81%), followed by the global approach (79%). In the external dataset, without any further retraining, an accuracy of 72% was achieved for the white matter model and 71% for the global approach. Data Conclusion: The global approach offered the best trade-off between internal performance and external validation to stratify MS patients based on accumulated disability.
更多查看译文
关键词
multiple sclerosis,classification,structural MRI,deep learning,input sampling
AI 理解论文
溯源树
样例
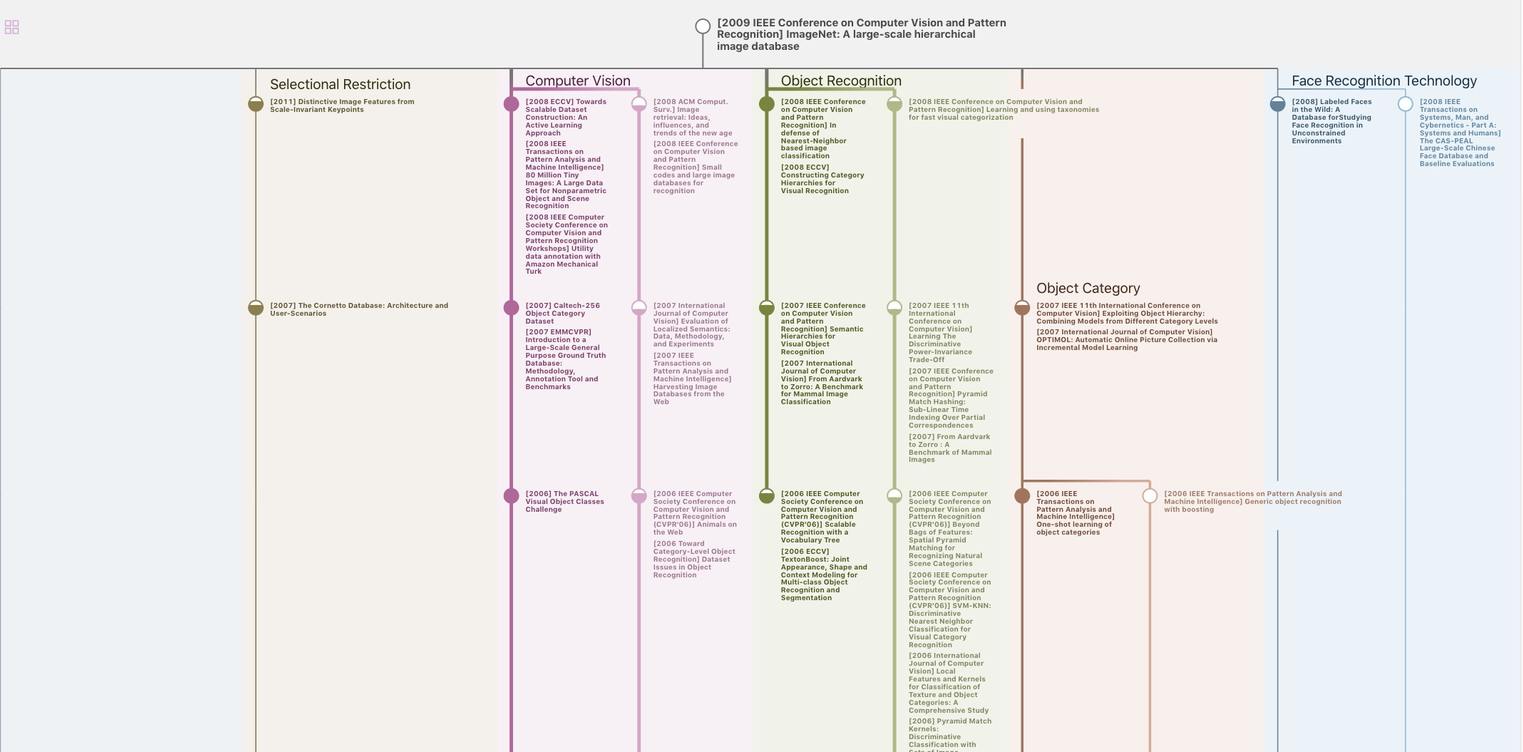
生成溯源树,研究论文发展脉络
Chat Paper
正在生成论文摘要