MPass: Bypassing Learning-based Static Malware Detectors.
DAC(2023)
摘要
Machine learning (ML) based static malware detectors are widely deployed, but vulnerable to adversarial attacks. Unlike images or texts, tiny modifications to malware samples would significantly compromise their functionality. Consequently, existing attacks against images or texts will be significantly restricted when being deployed on malware detectors. In this work, we propose a hard-label black-box attack MPass against ML-based detectors. MPass employs a problemspace explainability method to locate critical positions of malware, applies adversarial modifications to such positions, and utilizes a runtime recovery technique to preserve the functionality. Experiments show MPass outperforms existing solutions and bypasses both state-of-the-art offline models and commercial ML-based antivirus products.
更多查看译文
关键词
adversarial attacks,adversarial modifications,commercial ML-based antivirus products,hard-label black-box attack,learning-based static malware detectors,machine learning,malware critical position location,malware samples,ML-based detectors,MPass,problem-space explainability method,tiny modifications
AI 理解论文
溯源树
样例
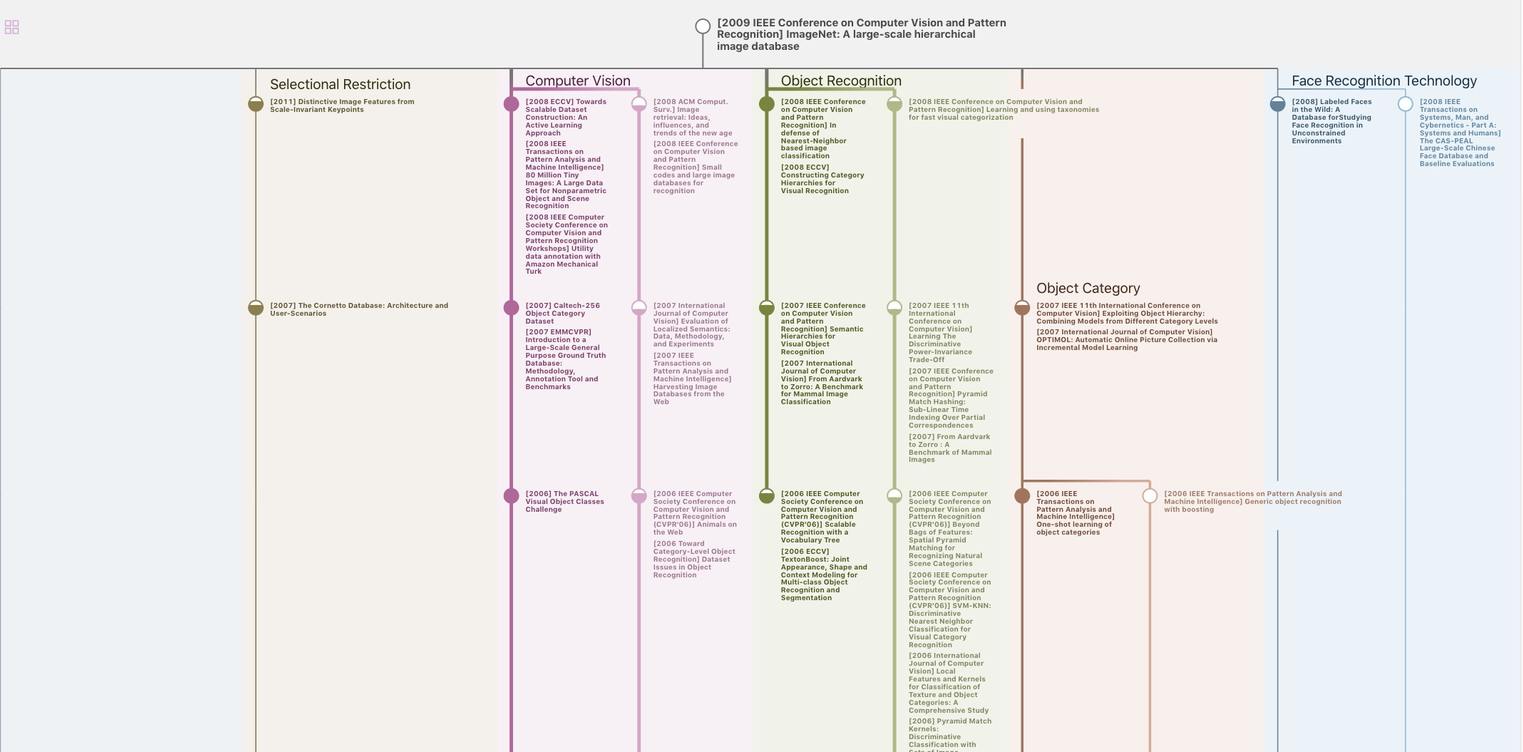
生成溯源树,研究论文发展脉络
Chat Paper
正在生成论文摘要